MoCha: Towards Movie-Grade Talking Character Synthesis

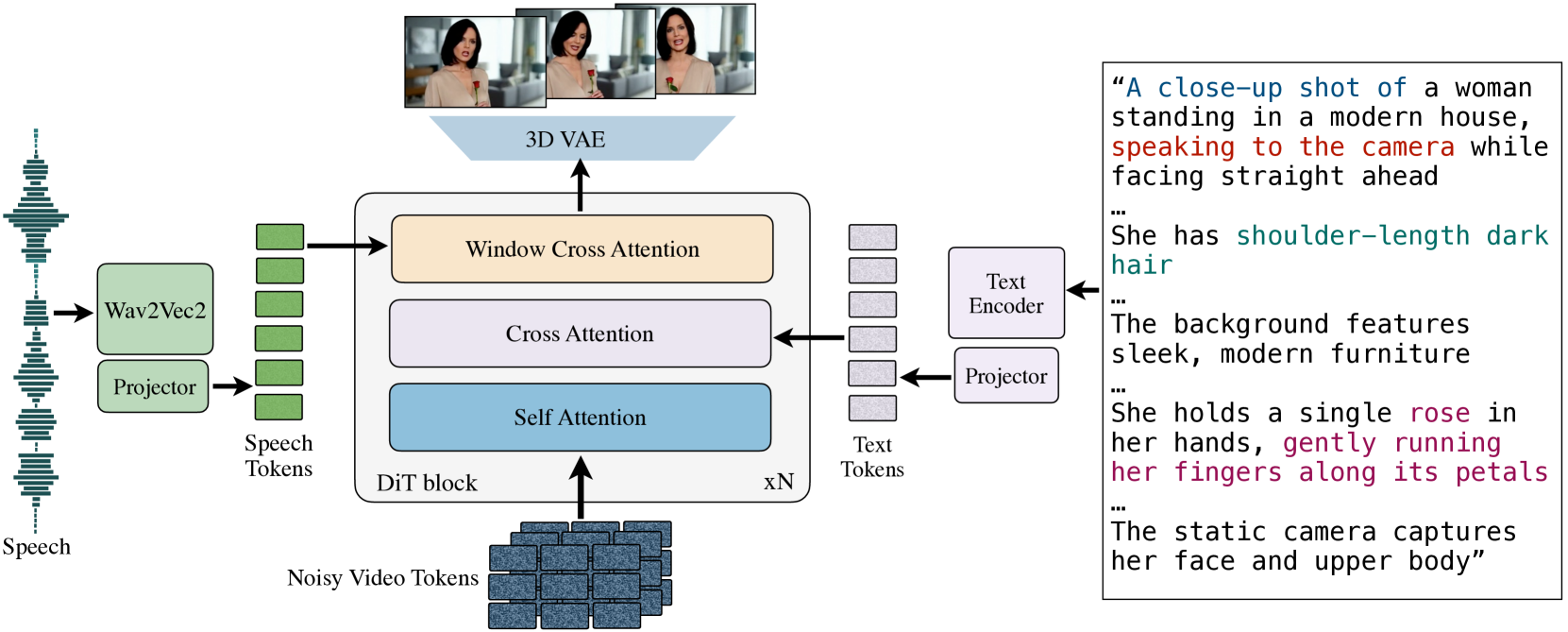
This is a Plain English Papers summary of a research paper called MoCha: Towards Movie-Grade Talking Character Synthesis. If you like these kinds of analysis, you should subscribe to the AImodels.fyi newsletter or follow me on Twitter.
Overview
- MoCha creates realistic talking characters from text and speech
- Uses advanced diffusion models trained on movie clips
- Preserves speaker identity while matching lip movements to speech
- Introduces innovative multi-stage training process
- Achieves state-of-the-art results in talking head synthesis
Plain English Explanation
MoCha stands for Movie-Grade Character synthesis, and it's a breakthrough in creating realistic talking video characters from just text and speech. Think of it as a digital puppeteer that can make any character speak naturally, with accurate lip movements that match the audio.
The core innovation is how MoCha uses a huge dataset of movie clips to learn what real talking humans look like. Unlike previous systems that focused only on faces or made robotic-looking results, MoCha creates natural-looking videos of people speaking that preserve their identity and character.
The team essentially built a system that can "watch" thousands of movie scenes, learn how people move their lips and faces when speaking, and then reproduce those movements on any character. It's like teaching a computer to be a master impressionist who can make any character appear to speak any words naturally.
What makes this special is that MoCha handles the entire talking character synthesis process in one system - it doesn't just paste a mouth onto a still image, but creates complete, natural video of a person speaking.
Key Findings
- MoCha produces significantly more realistic talking head videos than previous methods
- The system maintains character identity while precisely matching lip movements to speech
- The multi-stage training approach (pre-training and fine-tuning) dramatically improves results
- MoCha can generate high-quality 4-second video clips at 512x512 resolution
- Human evaluators strongly preferred MoCha's outputs over previous state-of-the-art methods
- The model works well with various speech styles, languages, and character appearances
The researchers demonstrated that their novel training approach - combining movie clips with a specific speech-to-video fine-tuning phase - creates significantly more natural motion character synthesis than previous methods. Their human evaluation studies showed viewers consistently rated MoCha's videos as more realistic.
Technical Explanation
MoCha is built on a diffusion transformer architecture that learns to generate video frames conditioned on speech audio and text. The system consists of three key components: a speech encoder, a text encoder, and a video diffusion model.
The training process occurs in two distinct phases. First, the model undergoes pre-training on a large dataset of movie clips where it learns general speech-to-video correspondence patterns. Then, it goes through a fine-tuning phase specifically focused on talking head synthesis.
For the speech encoder, MoCha uses CLAP (Contrastive Language-Audio Pre-training), which effectively extracts audio features from speech. The text encoder leverages T5, a powerful language model that converts text descriptions into meaningful embeddings. These encoders feed into a diffusion transformer that gradually denoises random patterns into coherent video frames.
The 3D human synthesis model uses a cross-attention mechanism to align the speech and text features with the video generation process. This allows precise control over the lip movements and facial expressions in relation to the speech content.
One of the most significant technical innovations is the two-stage training approach. By pre-training on general movie content and then fine-tuning specifically for talking heads, MoCha learns both broad human motion patterns and detailed speech articulation. This creates more coherent and realistic results than previous approaches that lacked this structured training methodology.
Critical Analysis
Despite its impressive results, MoCha has several limitations. The model is currently restricted to generating relatively short video clips (around 4 seconds), which limits its practical applications for longer content. The researchers acknowledge this limitation and suggest that future work should focus on extending temporal capabilities.
The training data, while extensive, may contain biases present in movie content, potentially leading to uneven performance across different demographics. The paper doesn't thoroughly address how these biases might affect the system's fairness or representation abilities.
Another limitation is that MoCha doesn't provide explicit control over non-speech expressions or body movements. While the system preserves character identity, users cannot specifically direct emotional expressions or gestures beyond what's implied by the speech patterns. This contrasts with some controllable character video approaches that offer more granular control.
The computational requirements for training and running MoCha are substantial, which may limit accessibility. The paper doesn't fully explore lighter-weight alternatives that might make the technology more widely available.
Finally, there are ethical considerations around deepfake potential that deserve more examination. The ability to make realistic videos of people saying things they never said raises important questions about consent and misuse that the paper acknowledges but doesn't fully address.
Conclusion
MoCha represents a significant step forward in realistic talking character synthesis, bringing us closer to creating digital humans that can naturally communicate through speech. Its movie-trained approach results in videos that maintain character identity while accurately matching lip movements to speech input.
The implications extend beyond entertainment applications like dubbing films or creating virtual influencers. This technology could transform how we interact with digital assistants, virtual teachers, and other AI systems by giving them more natural human interfaces. The pose-based motion capabilities could also enhance accessibility tools for those with communication challenges.
As this technology continues to develop, addressing the current limitations in duration, control, and ethical safeguards will be crucial. MoCha lays important groundwork for future research that might eventually enable seamless, realistic digital human communication across any language or context.
If you enjoyed this summary, consider subscribing to the AImodels.fyi newsletter or following me on Twitter for more AI and machine learning content.
Subscribe to my newsletter
Read articles from Mike Young directly inside your inbox. Subscribe to the newsletter, and don't miss out.
Written by
