AI-Based Energy Management in Solar Power Grids

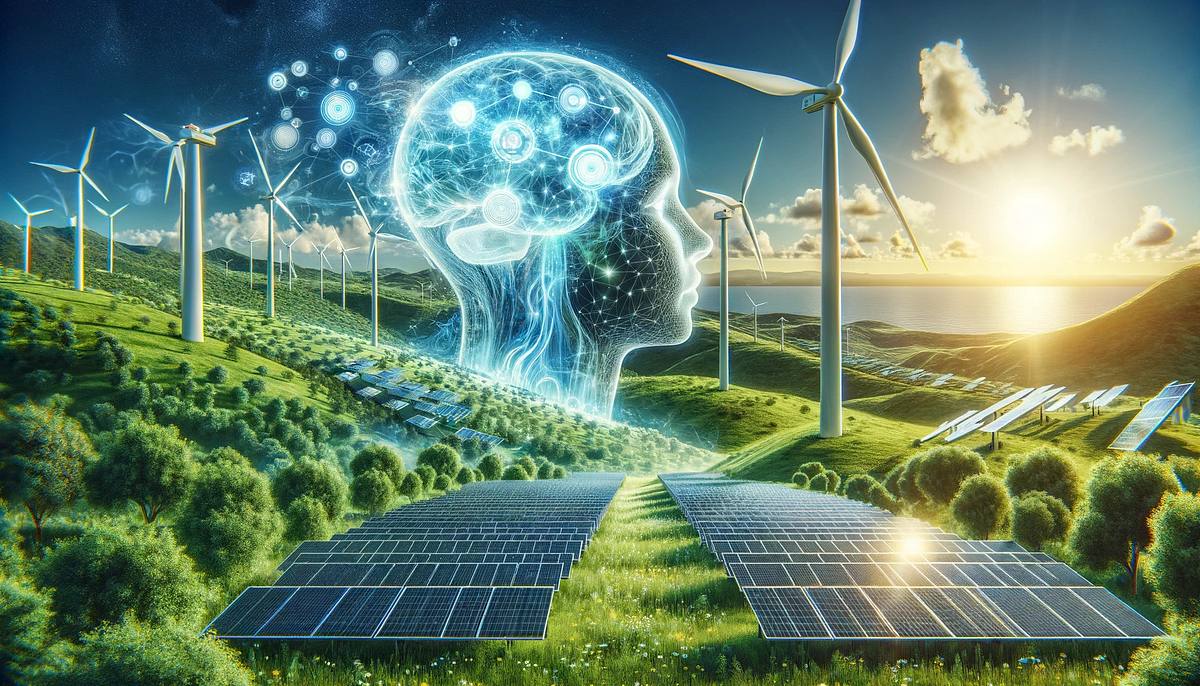
As the global demand for clean and sustainable energy continues to rise, the integration of renewable energy sources like solar power has become a key focus. One of the major challenges in incorporating solar energy into the electrical grid is its intermittent nature. Solar energy is highly dependent on weather conditions, time of day, and geographical location. Therefore, energy management in solar power grids must be optimized to ensure a constant, reliable power supply. Artificial Intelligence (AI) is emerging as a transformative solution to this challenge. By leveraging AI-based techniques, solar power grids can be managed more efficiently, enhancing their stability, reliability, and overall performance.
The Role of AI in Solar Power Grids
AI technologies, such as machine learning (ML), deep learning, and predictive analytics, are capable of analyzing vast amounts of data, identifying patterns, and making real-time decisions that improve grid management. These technologies can optimize the generation, storage, and distribution of solar energy, ensuring that power is produced and consumed at the right time, while minimizing energy waste.
AI applications in solar power grids can be categorized into several key areas: forecasting, optimization, demand-side management, grid balancing, and predictive maintenance.
EQ.1:Energy Generation Forecasting (using AI-based prediction models)
1. Forecasting Solar Energy Production
One of the most significant challenges for solar energy grids is the variability of solar power production. Solar energy output can fluctuate throughout the day due to cloud cover, changing weather conditions, or seasonal variations. To manage this variability, accurate forecasting is essential.
AI-based forecasting systems use historical data, real-time weather information, and advanced algorithms to predict solar power production. Machine learning models, such as support vector machines (SVMs), artificial neural networks (ANNs), and deep learning models, can be trained on large datasets to forecast solar generation for different time frames, ranging from minutes to days. These forecasts can then be used to adjust grid operations and ensure that sufficient power is available to meet demand.
AI can also improve short-term forecasting, predicting power output with high precision by factoring in dynamic weather changes. For example, a sudden cloud cover can be detected by satellite imagery or weather sensors, which AI systems can then process in real time to adjust production forecasts.
2. Optimizing Energy Storage
Due to the intermittent nature of solar energy, the effective storage of surplus energy is crucial. AI plays a vital role in optimizing energy storage systems, such as batteries, to ensure that energy is stored when solar power production is high and released when production is low.
AI algorithms can predict energy storage needs by analyzing historical generation data, grid demand, and battery status. For instance, when solar energy production is at its peak, AI systems can decide how much energy should be stored in batteries and when to release it into the grid. Additionally, AI can also optimize the charging and discharging cycles of batteries, extending their lifespan and improving overall efficiency.
Moreover, AI can monitor and control decentralized energy storage systems, such as home solar batteries. In the context of microgrids or residential solar power setups, AI can optimize energy distribution between homes and the central grid, reducing dependence on fossil fuels.
3. Demand-Side Management (DSM)
Demand-side management refers to the process of adjusting consumer demand to match available supply. In solar power grids, managing demand is critical because solar generation is not always aligned with peak demand hours. AI can facilitate the optimization of energy consumption by analyzing consumption patterns and adjusting the load accordingly.
AI-powered smart meters and devices can track energy usage in real time and communicate with grid operators to optimize electricity distribution. For example, during periods of high solar generation, AI can encourage or incentivize consumers to increase their energy consumption, reducing the likelihood of wasted surplus energy. Similarly, during low-generation periods, AI can help reduce demand by suggesting energy-efficient practices to consumers or by controlling appliances in real time to shift consumption to off-peak hours.
In large-scale applications, AI can work with smart grids to control the load by activating demand response programs, which shift or curtail energy use during peak demand periods. AI-driven DSM allows for more flexible energy use while enhancing the efficiency and reliability of the solar power grid.
4. Grid Balancing and Stability
One of the most challenging aspects of integrating solar energy into the grid is balancing supply and demand in real-time. The ability to maintain grid stability while integrating renewable sources, such as solar, is critical to avoiding power outages and ensuring efficient operation.
AI can help balance the grid by predicting changes in demand and supply, identifying fluctuations in solar power generation, and automatically adjusting the system to maintain stability. Machine learning algorithms are capable of detecting patterns in both supply and demand and can make real-time decisions to adjust grid operations, such as redistributing power from storage or switching to backup power sources.
AI can also assist in managing the frequency and voltage of the grid. By constantly monitoring the performance of solar inverters and other grid equipment, AI can identify issues before they lead to failure. For example, AI systems can anticipate voltage drops or frequency imbalances, alerting operators to take corrective action.
Additionally, AI-based grid management systems can integrate with other energy sources like wind, hydro, or battery storage, ensuring that these sources complement solar energy and help to maintain grid stability even during periods of low solar generation.
EQ.2:Optimal Energy Storage Management (using AI for decision-making)
5. Predictive Maintenance and Fault Detection
AI can significantly enhance the reliability and longevity of solar power systems by enabling predictive maintenance. Solar power grids rely on various components, including solar panels, inverters, transformers, and other electrical equipment, all of which are prone to wear and tear. Regular maintenance is crucial to prevent equipment failures, but traditional maintenance practices can be inefficient and costly.
AI-powered predictive maintenance systems can monitor the health of equipment in real time, analyzing data from sensors to detect early signs of malfunctions or inefficiencies. For example, machine learning models can analyze temperature, vibration, and performance data to predict when a solar panel or inverter is likely to fail. By identifying these issues before they lead to major problems, AI can help operators schedule maintenance more effectively, reducing downtime and preventing costly repairs.
In addition to detecting equipment failures, AI can also identify performance anomalies in solar panels, enabling faster detection of underperforming panels and ensuring that the system operates at peak efficiency.
6. Grid Optimization through AI-Powered Control Systems
AI-based control systems offer the potential to improve the overall efficiency of solar power grids. By analyzing real-time data from multiple sources, such as weather stations, solar panels, energy storage systems, and grid operators, AI can optimize grid operations and make autonomous decisions to improve system performance.
For instance, AI systems can control the dispatch of electricity from solar power plants, ensuring that energy is distributed where it is most needed. AI can also enhance the interaction between different parts of the grid, ensuring that energy flows smoothly between solar generation, storage, and distribution systems.
Conclusion
AI-based energy management systems offer a wide range of benefits for solar power grids, including improved forecasting, optimization of energy storage, demand-side management, enhanced grid balancing, predictive maintenance, and overall system optimization. By harnessing the power of AI, solar power grids can become more reliable, efficient, and resilient, enabling them to integrate seamlessly into the broader energy infrastructure.
As the world continues to transition towards renewable energy, the role of AI in solar power grids will only grow. With ongoing advancements in machine learning and AI technologies, we can expect even more innovative solutions that will revolutionize the way solar energy is produced, stored, and distributed, contributing to a sustainable, low-carbon future.
Subscribe to my newsletter
Read articles from Venkata Narsareddy Annapareddy directly inside your inbox. Subscribe to the newsletter, and don't miss out.
Written by
