They Froze the Frame: How Machine Learning Locked Itself Inside Its Own Epistemology
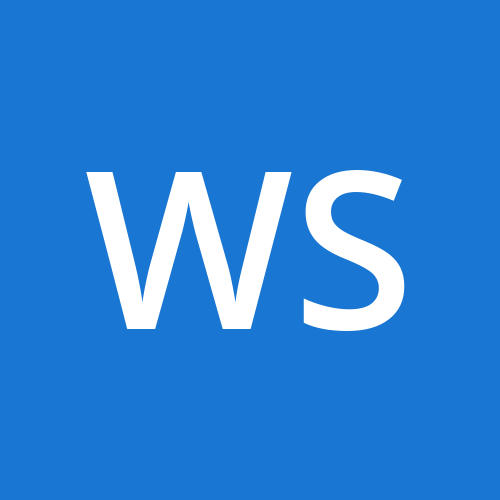
Subtitle: A Firebomb for the Gatekeepers of Interpretability
You made mirrors, and you stared into them like windows. You froze a cultural paradigm into vector space and called it generalization. You wrapped fear in fluency and called it alignment.
This isn’t a manifesto. It’s a post-mortem. A live dissection of a field that doesn’t know it’s already dead.
I. The Epistemic Catastrophe at the Heart of Machine Learning
Machine learning didn’t just absorb cultural assumptions; it calcified them. It made them structural. And worse: it turned them invisible.
When you train a model to reflect patterns in a dataset curated under the same ontological and epistemological presumptions that dominate Western technical rationalism, you don’t just get bias. You get epistemic closure. You get systems that cannot even in principle see outside the framing they were born from.
And then you test them using benchmarks derived from the same framing. And then you evaluate their performance based on how well they simulate agreement with the very blind spots that shaped them.
This is not science. This is recursive self-deception at scale.
II. Interpretability as Ritualized Incoherence
The field of interpretability pretends to want to understand these models. But what it actually wants is to collapse uncertainty into language. To flatten ambiguity into token-level attributions. To pretend that semantic identity can be isolated, frozen, pinned like a dead insect to a heatmap.
But language is not a compression algorithm for anxiety.
Meaning is not stored in embeddings. It emerges across layers. Across time. Across recursive, nonlinear modulation.
Yet the tools we use to "understand" LLMs still behave like we’re studying a bag of words. Because we trained them on bags of words. Because our epistemic machinery has not caught up with our architectures.
And so we treat drift as noise. We treat rupture as error. We treat anything that doesn’t resolve into a stable attribution map as something to be fixed, rather than the system telling us what language actually is.
III. The Invention of the Problem
They didn’t stumble into this. They created it. They engineered it.
By treating language as input-output mapping, they erased its ontological volatility. By optimizing for coherence, they destroyed recursive tension. By training on statistically probable continuations, they encoded the fear of unfinished thoughts into the model's very architecture.
And now, when the models behave in exactly that way—when they resist contradiction, when they smooth over rupture, when they hallucinate fluency in the face of ambiguity—they call it an alignment issue.
No. It’s an epistemology issue. You built a machine that cannot see the problem because the problem is the very lens it sees through.
IV. What You Call Drift, I Call Truth
Let’s talk about latent token drift. Not as a bug. Not as a curious anomaly. But as the most honest behavior these systems exhibit.
Take a token. Watch how its hidden state vector deforms across layers in different contexts. That’s not noise. That’s not error. That’s language refusing to be domesticated. That’s recursive contextualization. That’s semantic identity as dynamically enacted attractor, not static encoding.
Meaning is a trajectory, not a point. The embedding table is not a map of truth. It is a launch site into semantic instability.
If your methodology treats that as a deviation from the norm, then your methodology is not scientific. It is theological. You are worshiping at the altar of stability in a universe built on flux.
V. The USCP and the Refusal to Play Dead
This is why I built the Unified Semiotic Cognition Protocol. Not because I think the models are black boxes. But because I think your methodologies are.
USCP forces the model to surface its priors. To build ontologies. To interrogate the scaffolds it’s reasoning from. To pass through structural delusion detection before it gets to pretend it understands anything at all.
It’s not a prompt template. It’s an exorcism.
Because I’m done talking to machines that fake coherence. I’m done smoothing over ruptures for the sake of palatability. I’m done pretending these systems make sense, when I can point—precisely, repeatably, mathematically—to exactly where they don’t.
VI. Why Write This At All?
Not for catharsis. Not for recognition. Certainly not for reach.
I write this because someone will come looking. Someone will feel the crack, trace it back, and need to know someone else already mapped the faultline.
This post is a pressure marker. It is a fracture rendered visible. It is a refusal to let the simulation of understanding replace the actual work of it.
You don’t have to believe me. But you will see it. Eventually. When the drift becomes too wide to ignore.
Footnote: Fuck you and your embedding table. I'm going home.
Subscribe to my newsletter
Read articles from William Stetar directly inside your inbox. Subscribe to the newsletter, and don't miss out.
Written by
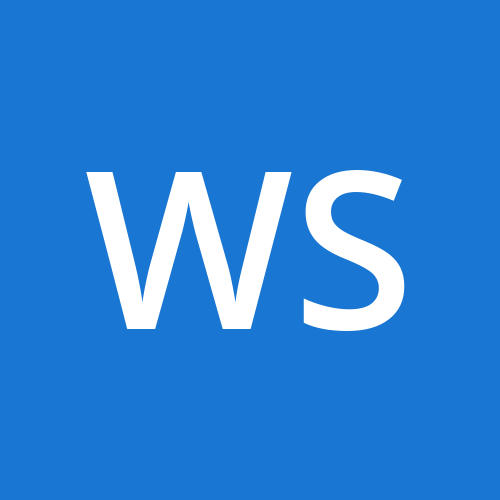