AI in Toxicology: Predicting Drug Safety and Adverse Reactions

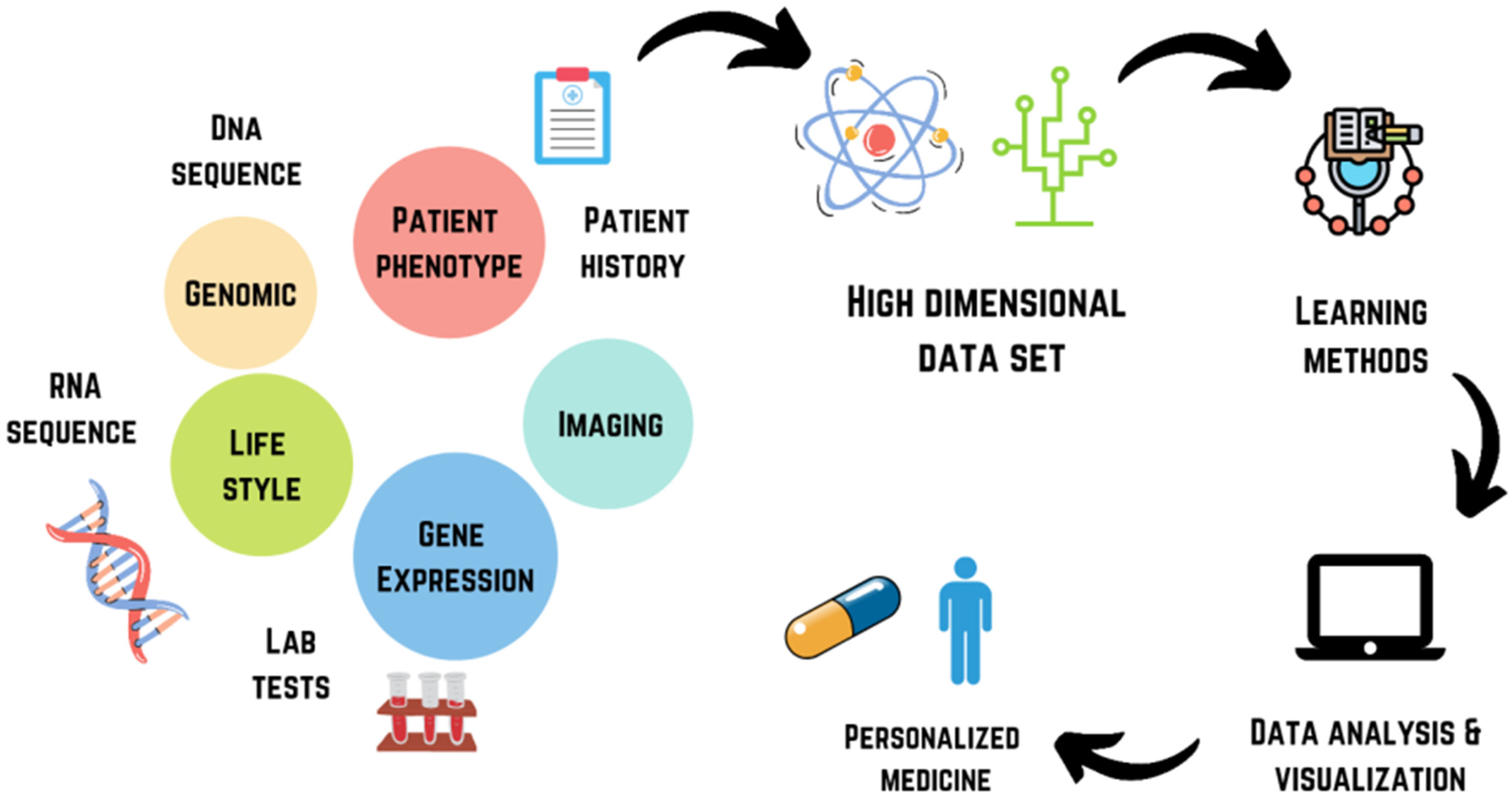
AI in Toxicology: Predicting Drug Safety and Adverse Reactions
In the field of pharmaceutical research, one of the most critical stages of drug development is determining the safety of new compounds. Traditionally, this process has relied on animal testing, in vitro studies, and clinical trials. While these methods have proven useful, they are not without their limitations—namely, their time-consuming nature, high costs, and ethical concerns. Additionally, not all human toxicological responses can be accurately predicted using animal models, leading to a significant demand for alternative approaches. In recent years, the advent of artificial intelligence (AI) has revolutionized drug discovery and toxicology, providing innovative ways to predict drug safety and adverse reactions. This article explores the role of AI in toxicology, its applications, and its potential to transform the pharmaceutical industry.
EQ.1:Quantitative Structure-Activity Relationship (QSAR) Equation
The Traditional Approaches to Toxicology
Before diving into the application of AI in toxicology, it’s important to understand the traditional methods employed to assess the safety of drugs. Historically, toxicological assessments have included animal testing (such as rodents and non-human primates), in vitro testing using cultured cells, and computational models based on known toxicological data.
1. Animal Testing: This is one of the most common approaches to studying drug toxicity. However, ethical concerns about animal welfare, as well as the significant differences between species, can make this approach problematic. Animals may not always react the same way as humans to drugs, leading to false positives or negatives in toxicity testing.
2. In Vitro Testing: This approach involves using human or animal cells to test drug reactions. While this can reduce some of the ethical concerns associated with animal testing, in vitro methods still have limitations when it comes to replicating the complex interactions that occur in a living organism.
3. Computational Models: These models use historical toxicological data to predict how new drugs might react. While computational methods are faster and cheaper than animal testing, they often lack the sophistication required to make accurate predictions, especially for novel compounds with no historical data.
Enter AI: Revolutionizing Toxicology
Artificial intelligence, particularly machine learning (ML) and deep learning (DL), has the potential to significantly enhance the way drug safety is assessed. By analyzing large datasets, AI models can uncover patterns and relationships that would be difficult, if not impossible, for humans to identify. This ability is particularly useful in predicting adverse drug reactions (ADRs), drug interactions, and other toxicological endpoints.
AI has several advantages over traditional methods:
Efficiency: AI models can process vast amounts of data far more quickly than human researchers or traditional computational models, drastically reducing the time needed for drug safety assessment.
Accuracy: AI algorithms can identify complex, non-linear relationships between molecular structure and toxicity, improving the accuracy of predictions, especially when dealing with new or poorly understood compounds.
Cost-Effectiveness: By reducing the need for expensive animal testing and in vitro experiments, AI can help lower the cost of drug development.
Ethical Considerations: AI reduces the reliance on animal testing, aligning with ethical guidelines that promote more humane approaches in scientific research.
Applications of AI in Toxicology
There are several key areas where AI is being applied in toxicology:
1. Predicting Adverse Drug Reactions (ADRs): One of the most significant applications of AI in toxicology is in the prediction of ADRs. ADRs are unwanted or harmful reactions that occur after a drug is administered, and they represent a major cause of drug failure and regulatory delays. AI models can analyze large datasets of patient information, including genetic profiles, medical histories, and previous drug reactions, to predict how an individual might respond to a particular drug. This personalized approach to drug safety can help identify high-risk patients before they are exposed to harmful compounds.
2. Toxicity Prediction Models: AI has the ability to predict the toxicity of new chemical compounds based on their molecular structure. Using machine learning algorithms, researchers can develop predictive models that correlate specific molecular features with the likelihood of toxicity. For example, the use of quantitative structure-activity relationship (QSAR) models allows for the prediction of a compound's biological activity based on its chemical structure. This can be invaluable for screening new drug candidates early in the discovery process, reducing the need for extensive animal testing.
3. Drug-Drug Interaction Prediction: Drug-drug interactions (DDIs) are another major concern in drug safety. AI algorithms can analyze large datasets to identify potential interactions between drugs, helping to predict adverse outcomes such as overdose, reduced efficacy, or toxic effects. By using data from clinical trials, electronic health records, and other sources, AI can help identify combinations of drugs that are more likely to cause harm.
4. Biomarker Discovery: AI is also being used to discover biomarkers that indicate toxicity. Biomarkers are biological indicators that can help detect the presence of toxicity in an organism. By analyzing large datasets from clinical trials, genomic studies, and other sources, AI can identify specific genes, proteins, or metabolites that are indicative of toxic reactions, providing an early warning system for potential drug safety issues.
5. In Silico Toxicity Testing: In silico testing, or computer-based modeling, is a crucial part of the drug development process. AI enhances in silico testing by providing more sophisticated and accurate simulations of human biology, enabling the prediction of how drugs will behave in the body. AI can model the interactions between drugs and biological systems in much more detail than traditional methods, providing insights into potential toxic effects that may not be evident from animal testing or in vitro experiments.
Challenges and Limitations of AI in Toxicology
While AI holds great promise in transforming toxicology, it is not without its challenges. Some of the key limitations include:
1. Data Quality and Availability: The success of AI models relies heavily on the quality and availability of data. Incomplete, biased, or inaccurate datasets can lead to unreliable predictions. Additionally, access to high-quality data may be limited due to privacy concerns or regulatory restrictions.
2. Interpretability: Many AI models, particularly deep learning models, are often considered "black boxes" because it is difficult to understand how they arrive at specific predictions. This lack of interpretability can be a barrier to their widespread adoption in highly regulated industries such as pharmaceuticals, where transparency is critical.
3. Regulatory Acceptance: While AI-driven approaches are gaining traction in the pharmaceutical industry, regulatory agencies like the FDA and EMA may still be cautious about fully embracing AI for drug safety assessments. Regulatory bodies require robust validation and transparent documentation to ensure the accuracy and reliability of AI predictions.
EQ.2:Adverse Drug Reaction (ADR) Prediction Model
Conclusion: The Future of AI in Toxicology
As AI technology continues to advance, its role in toxicology is expected to grow exponentially. The ability to predict drug toxicity more accurately and efficiently could shorten the drug development timeline, reduce costs, and ultimately improve patient safety. By integrating AI into the drug discovery process, pharmaceutical companies can move toward a more personalized and precise approach to drug safety, minimizing the risk of adverse reactions and increasing the likelihood of successful drug approvals.
While challenges remain in terms of data quality, interpretability, and regulatory acceptance, the potential benefits of AI in toxicology cannot be ignored. As AI technology continues to evolve, we can expect more groundbreaking advances in drug safety prediction, bringing us closer to safer, more effective treatments for a wide range of diseases.
Subscribe to my newsletter
Read articles from Sambasiva Rao Suura directly inside your inbox. Subscribe to the newsletter, and don't miss out.
Written by
