AI Training Data Practices Under Legal Scrutiny
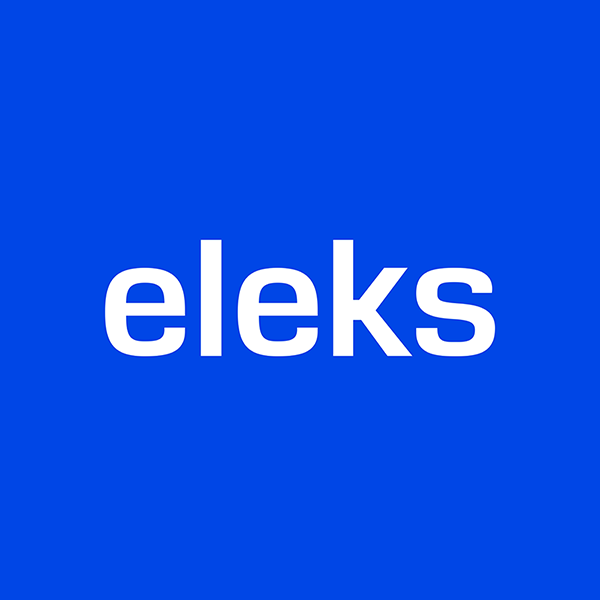
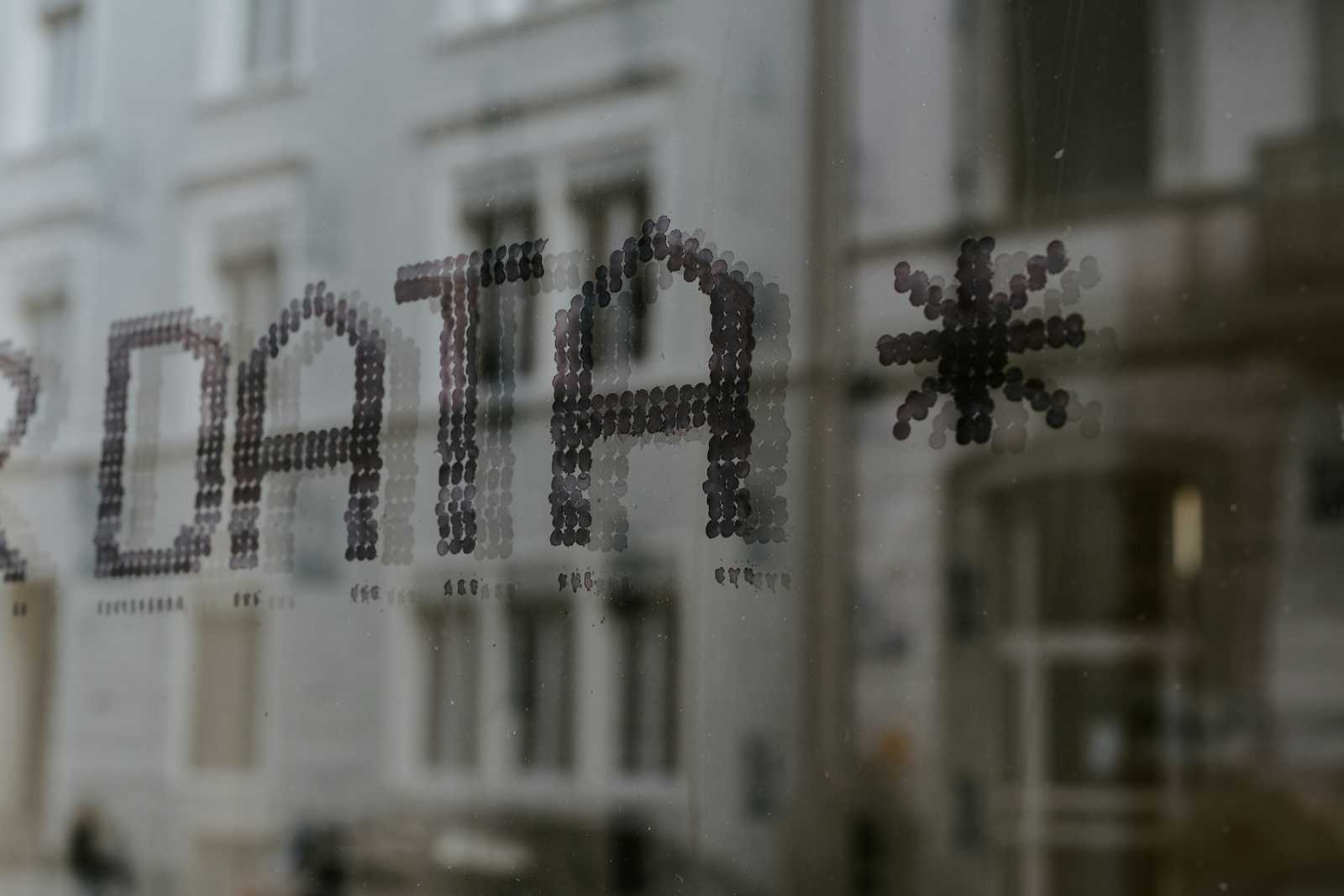
A recent U.S. court ruling in the case of Thomson Reuters v. Ross Intelligence is a significant moment for generative AI development. It could change how companies gather and use data for training their models. This is the first major case in the U.S. involving AI and copyright, and its effects go beyond just the companies involved.
Particularly significant is that the judge rejected the fair use argument, which AI companies often cite in similar disputes. In the judge's opinion, Ross was creating a direct competitor to Westlaw rather than transforming the content for research or educational purposes. If courts start following this case as a precedent, similar lawsuits will likely be filed against almost all companies involved in model training.
We talked about this with Volodymyr Getmanskyi, Head of the Data Science Office at ELEKS, to learn more about key complications around data governance and AI model training.
How will the ruling impact the way companies collect data for AI training?
Firstly, this is the problem around the question of how to classify data, especially in Web 4.0, starting from the question of how your browser caches data and whether you can reuse it further.
Data science or AI professionals are typically aware of such issues (public sources or copyright metadata in datasets) and always check it before usage. However, there can be some controversial cases, like when OpenAI's CTO, Mira Murati, couldn’t say what data was used to train Sora in a WSJ interview.
How can training data origins be tracked?
There are some thoughts around it that look quite innovative, like using blockchain blocks to track the distribution or some kind of steganography to hide copyright information in data. However, the main question regarding the verification of trained models remains open, especially in cases of distillation or transfer learning; how to inspect the parameters and forward propagation path to determine the presence of specific samples is still unclear.
How effective are synthetic data generation methods?
There are many cases where synthetic datasets help a lot, but there is also another side question–in case some module/algorithm/model knows how to generate data, knows all dependencies and differences inside data, why not use it as the primary model w/o generation and additional training or architecture search?
From a technical viewpoint, can companies create data filtering systems that exclude any copyrighted content?
The data or samples should be labelled first, and then go through the easiest filter based on IP labels. Without that, the only option is to find each sample somewhere on the internet, check for the primary source (origin), and verify the copyright. And it looks too complicated and time-consuming.
Want to ensure your AI training practices are future-proof? Book a consultation with the expert!
Subscribe to my newsletter
Read articles from ELEKS directly inside your inbox. Subscribe to the newsletter, and don't miss out.
Written by
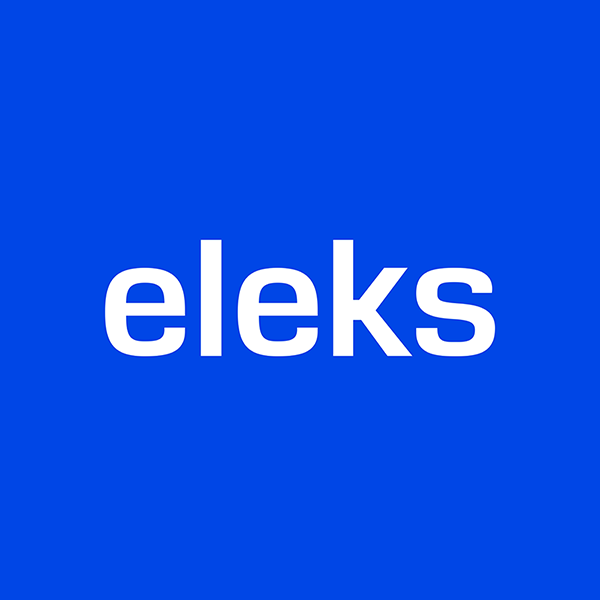
ELEKS
ELEKS
A global software development and technology consulting company. We're passionate about pioneering innovation and crafting elegant, sustainable technology solutions.