How AI-Powered Analytics is Transforming Data Science in 2025

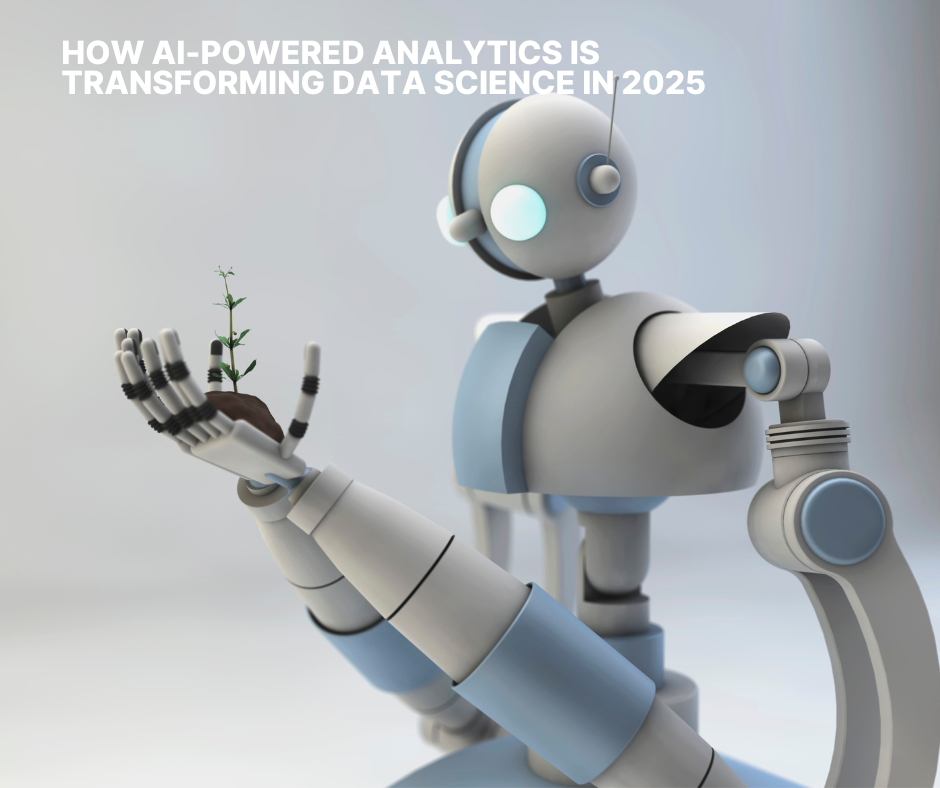
The rapid evolution of Artificial Intelligence (AI) has revolutionized various industries, and data science is no exception. In 2025, AI-powered analytics is reshaping the way businesses and organizations process, analyze, and interpret data. From making faster decisions to discovering hidden insights, AI is unlocking new opportunities for innovation and efficiency in data science. In this article, we will explore how AI-powered analytics is transforming the data science landscape and why it’s a game-changer for the industry.
The Rise of AI in Data Science
What is AI-Powered Analytics?
AI-powered analytics refers to the integration of artificial intelligence techniques like machine learning, natural language processing (NLP), and deep learning with data analysis tools to derive insights from data automatically. These AI technologies enable computers to analyze vast amounts of data, recognize patterns, and make predictions with minimal human intervention. In 2025, AI-powered analytics platforms are able to understand complex datasets, perform predictive analytics, and automate routine tasks, making them a must-have for data-driven businesses.
How AI is Reshaping the Data Science Workflow
In traditional data science workflows, human analysts spent considerable time cleaning data, identifying patterns, and building models. With AI, much of this work is automated. Here’s how AI is impacting the data science process:
Data Cleaning and Preprocessing: AI algorithms can automatically detect and correct errors in datasets, identify outliers, and transform raw data into structured formats. This reduces the time and effort required for data cleaning, allowing data scientists to focus on more strategic tasks.
Feature Engineering: AI models can automatically extract and generate features from data without requiring manual intervention. This leads to more accurate predictive models and saves time.
Model Building and Optimization: AI algorithms can test multiple models and automatically select the best one based on the data. Furthermore, machine learning models can continuously improve and adapt based on new data, resulting in more accurate insights over time.
Automation of Repetitive Tasks: Many routine data science tasks, such as data exploration and reporting, can now be automated using AI-powered tools, significantly boosting productivity.
Key Benefits of AI-Powered Analytics in Data Science
1. Faster Decision-Making
One of the most significant advantages of AI-powered analytics is the ability to provide real-time insights. Traditional data analysis could take hours or even days to produce results. In contrast, AI-powered tools can process data almost instantly, enabling businesses to make quick, data-driven decisions.
For example, AI can help companies identify customer behavior trends, optimize marketing campaigns, or detect fraud in near real-time, which helps organizations stay competitive in fast-paced industries.
2. Improved Predictive Analytics
Predictive analytics is a cornerstone of data science, and AI has significantly improved its accuracy. Machine learning models can now analyze historical data and predict future trends with remarkable precision. These models can forecast everything from sales performance to customer churn and even supply chain disruptions.
AI-powered predictive analytics can be a game-changer for businesses in sectors like finance, healthcare, and retail, where anticipating future events is crucial for maintaining a competitive edge.
3. Personalized Experiences
AI-powered analytics enables companies to provide personalized experiences to their customers by analyzing individual preferences, behaviors, and interactions. Whether it's recommending products, content, or services, AI can help businesses deliver highly tailored offerings to customers, improving satisfaction and engagement.
For instance, streaming platforms like Netflix and Spotify use AI to analyze user preferences and recommend shows, movies, or songs based on individual tastes.
4. Enhanced Data Visualization
In 2025, data visualization has become more intuitive thanks to AI-powered tools. These tools can automatically generate interactive, easy-to-understand visualizations that help non-technical stakeholders make sense of complex data. AI can also highlight key insights and trends in visual formats that are easier to interpret, helping organizations make informed decisions faster.
5. Cost Efficiency
AI-powered analytics reduces the need for manual intervention and repetitive tasks, saving companies both time and money. By automating data processing, feature engineering, and model selection, businesses can cut down on operational costs while ensuring that data science efforts are more efficient and impactful.
AI Techniques That Are Revolutionizing Data Science
1. Machine Learning
Machine learning (ML) is a subset of AI that focuses on developing algorithms that enable computers to learn from data and make decisions without explicit programming. In data science, ML is used for predictive modeling, classification, and clustering tasks. With the help of advanced ML algorithms, data scientists can build robust models that improve with more data, providing more accurate results over time.
2. Natural Language Processing (NLP)
NLP is a field of AI that enables computers to understand, interpret, and respond to human language. In data science, NLP is used to analyze textual data, such as customer reviews, social media posts, and chat logs. AI-driven NLP tools can automatically extract sentiments, trends, and actionable insights from vast amounts of unstructured text data, which would have been otherwise time-consuming and challenging for humans to analyze.
3. Deep Learning
Deep learning, a subset of machine learning, uses artificial neural networks with many layers to analyze large datasets and make predictions. Deep learning techniques are particularly effective in image and speech recognition, as well as analyzing large volumes of unstructured data. In 2025, deep learning models are being used to process data from various sources like medical images, satellite imagery, and video content.
4. Robotic Process Automation (RPA)
RPA involves automating repetitive tasks such as data entry, report generation, and data validation. When combined with AI, RPA can take on more complex tasks like data analysis and decision-making. This automation increases operational efficiency and frees up human analysts to focus on more value-driven activities.
Challenges of AI-Powered Analytics in Data Science
While AI-powered analytics offers numerous advantages, there are some challenges that need to be addressed:
Data Privacy and Security: As AI systems require vast amounts of data, ensuring data privacy and security remains a priority. Organizations must comply with regulations like GDPR and take steps to protect sensitive data.
Bias in AI Models: AI models are only as good as the data they are trained on. If the training data is biased, it can result in skewed or unfair outcomes. Data scientists must ensure that their models are fair, transparent, and free of bias.
Complexity of AI Systems: Implementing AI-powered analytics requires a high level of expertise. Data scientists must possess strong technical skills in AI, machine learning, and data engineering to effectively deploy AI solutions.
Integration with Legacy Systems: Many organizations still rely on legacy systems that may not be compatible with AI-powered tools. Integrating AI into existing infrastructure can be time-consuming and costly.
Conclusion
AI-powered analytics is fundamentally changing the way data science operates in 2025. From faster decision-making and predictive insights to automation and personalized experiences, AI is unlocking new levels of efficiency and innovation. However, as with any technology, it comes with challenges that need to be addressed. The key to success lies in the ability to leverage AI’s full potential while mitigating risks related to data privacy, bias, and system integration. For those looking to build expertise in this field, a Data Science Training course in Delhi, Noida ,Pune, Bangalore, and other parts of India can provide the skills necessary to navigate these challenges and capitalize on AI's opportunities.
Subscribe to my newsletter
Read articles from Ruhi Parveen directly inside your inbox. Subscribe to the newsletter, and don't miss out.
Written by

Ruhi Parveen
Ruhi Parveen
I am a Digital Marketer and Content Marketing Specialist, I enjoy technical and non-technical writing. I enjoy learning something new.