AI in Neurofeedback Training: Enhancing Brainwave Regulation Therapy

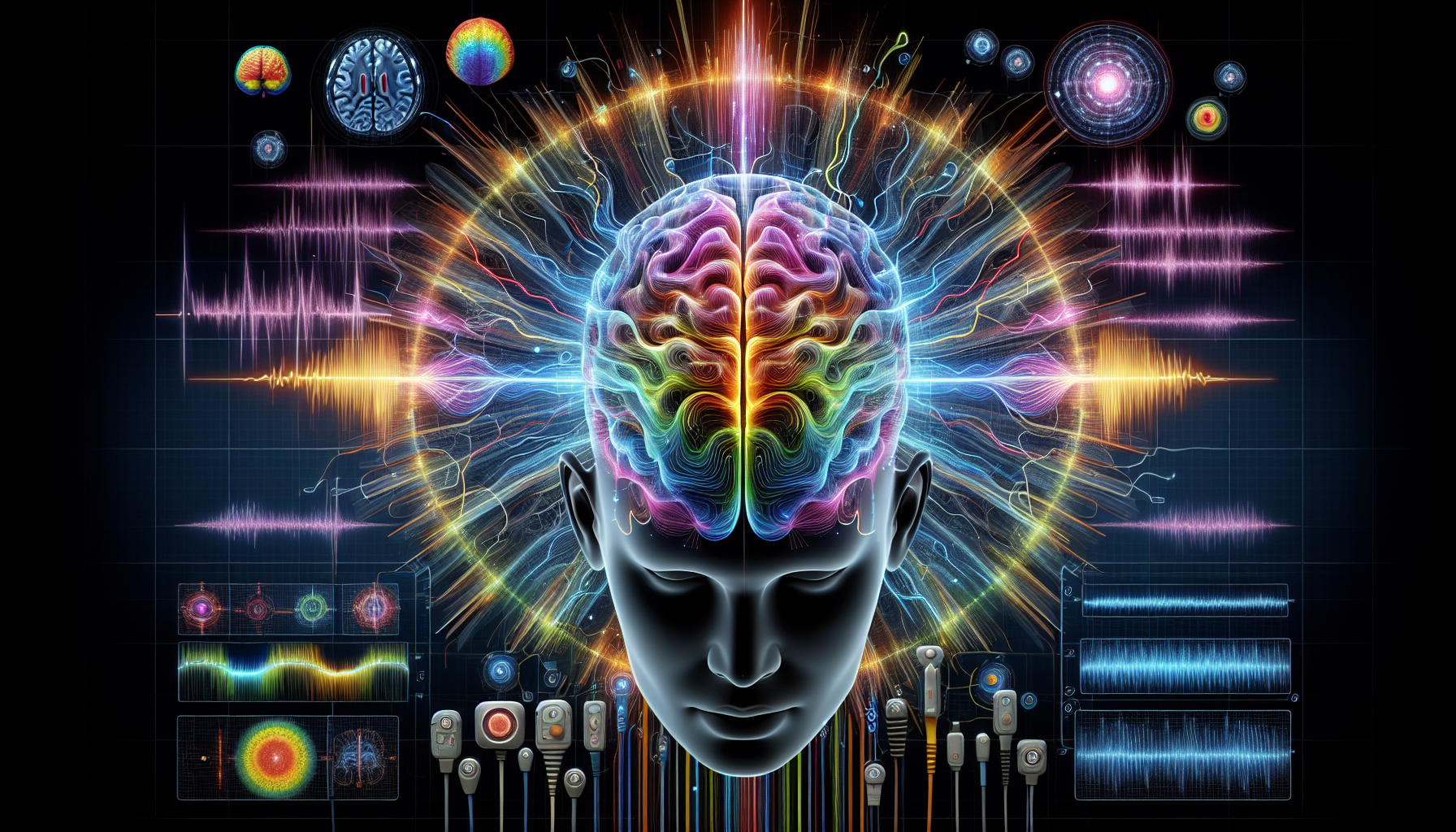
Neurofeedback training (NFT) is a therapeutic technique aimed at optimizing brain function by training individuals to regulate their brainwave activity. This method has been successfully used in treating various mental health conditions, including anxiety, ADHD, PTSD, and depression. Traditional neurofeedback relies on real-time biofeedback, where the brain's electrical activity is monitored, and individuals are taught to alter their brainwaves using visual or auditory cues. However, the integration of Artificial Intelligence (AI) into neurofeedback training holds significant promise in enhancing the precision, adaptability, and overall effectiveness of this therapy. This research explores the role of AI in neurofeedback, its applications, benefits, and challenges, providing a comprehensive look at how AI is revolutionizing brainwave regulation therapy.
The Basics of Neurofeedback Training
Neurofeedback is based on the principle of operant conditioning, where individuals learn to produce specific brainwave patterns to enhance cognitive performance or alleviate symptoms of mental health conditions. Electroencephalography (EEG) is the primary tool used in neurofeedback to measure brain activity. Brainwaves are categorized into different frequency bands: delta, theta, alpha, beta, and gamma. Each of these bands is associated with different mental states, such as relaxation, focus, or deep sleep. In NFT, patients are trained to increase or decrease the amplitude of specific brainwave frequencies to achieve desired mental states, with the goal of enhancing cognitive function or emotional regulation.
AI in Neurofeedback: An Emerging Paradigm
The integration of AI into neurofeedback is a relatively recent development, but it is already showing promising results in improving the therapy’s precision, adaptability, and efficacy. AI can enhance neurofeedback in several ways:
Real-Time Data Analysis and Personalization: AI algorithms are capable of processing vast amounts of data in real-time. In neurofeedback, this means the AI can quickly analyse brainwave patterns from EEG data and tailor the feedback to the individual's unique brain activity. AI can automatically detect irregularities in brainwave patterns, allowing for personalized interventions that are more effective than the one-size-fits-all approach of traditional neurofeedback. By continuously learning from the user’s responses, AI systems can adapt the feedback in real time, ensuring that the training remains relevant to the individual's evolving needs.
Improved Signal Detection and Noise Reduction: One of the challenges in traditional neurofeedback is the ability to differentiate between useful brainwave signals and noise. AI-powered systems, particularly machine learning algorithms, can enhance the signal-to-noise ratio by filtering out irrelevant data and focusing on the most significant patterns. This results in more accurate and reliable feedback, improving the overall quality of the training experience.
Enhanced Predictive Capabilities: AI can enhance the predictive aspect of neurofeedback by identifying patterns that may not be immediately apparent to human operators. By analysing large datasets over time, AI can predict how an individual’s brain will respond to specific training protocols. This predictive power enables clinicians to design more effective treatment plans, potentially reducing the trial-and-error phase often associated with neurofeedback therapy.
Real-Time Feedback Optimization: In traditional neurofeedback, the therapist often adjusts the feedback parameters based on observed patterns of brainwave activity. AI can automate this process, adjusting feedback in real time according to the individual's brainwave responses. This optimization can lead to faster training progress and more consistent results.
Applications of AI-Enhanced Neurofeedback
AI-enhanced neurofeedback has been applied in various domains, including mental health, cognitive enhancement, and even peak performance optimization. Some notable applications include:
EQ.1. Brainwave Frequency Band Classification (EEG Signal Decomposition):
Mental Health Treatment: AI-powered neurofeedback has shown promise in treating mental health conditions such as anxiety, depression, and PTSD. For example, studies have demonstrated that neurofeedback can help patients regulate the theta and beta waves associated with stress and emotional dysregulation. AI’s ability to personalize the training to each individual’s specific brainwave activity increases the efficacy of this treatment, offering an alternative to traditional pharmacological interventions.
Attention Deficit Hyperactivity Disorder (ADHD): ADHD is often characterized by irregular brainwave activity, particularly in the theta and beta frequency bands. Neurofeedback training, with AI’s assistance, can help individuals with ADHD train their brains to produce more normalized brainwave patterns. AI’s real-time data processing allows for highly individualized protocols that adjust to the unique needs of each patient, improving treatment outcomes for ADHD sufferers.
Cognitive Enhancement and Peak Performance: Beyond therapeutic applications, AI-enhanced neurofeedback is being used in the realm of cognitive enhancement and peak performance. Athletes, musicians, and professionals in high-stress environments are using neurofeedback to optimize focus, emotional regulation, and stress management. AI-driven neurofeedback systems can personalize training programs to help individuals reach their cognitive potential, boosting performance in various fields.
EQ.2. Learning Rate in Reinforcement Learning (AI Model):
Challenges and Future Directions
Despite the promising potential of AI in neurofeedback, several challenges remain. One major obstacle is the lack of standardized protocols for AI-based neurofeedback systems. Since the technology is still in its early stages, there is no consensus on the best AI models to use or how to integrate them with existing neurofeedback systems. Additionally, the effectiveness of AI-enhanced neurofeedback still requires more rigorous scientific validation through large-scale clinical trials.
Another challenge is the accessibility of AI-driven neurofeedback. High-quality neurofeedback systems with AI capabilities can be expensive, making them inaccessible to many individuals. Furthermore, the complexity of the technology requires specialized training for clinicians to operate, which can limit its widespread adoption.
Conclusion
AI is poised to revolutionize neurofeedback training by enhancing its precision, adaptability, and effectiveness. By integrating machine learning algorithms, real-time data analysis, and predictive capabilities, AI can personalize brainwave regulation therapy to an unprecedented degree. The applications of AI in neurofeedback span from mental health treatment to cognitive enhancement, showing the versatility and potential of this technology. However, for AI-driven neurofeedback to reach its full potential, more research and clinical validation are needed. As these challenges are addressed, AI-enhanced neurofeedback may become a powerful tool in improving mental health, cognitive performance, and overall well-being.
Subscribe to my newsletter
Read articles from Chandrashekhar Pandugula directly inside your inbox. Subscribe to the newsletter, and don't miss out.
Written by
