Bridging Data Silos with GenAI: The Next Leap in AI Data Integration

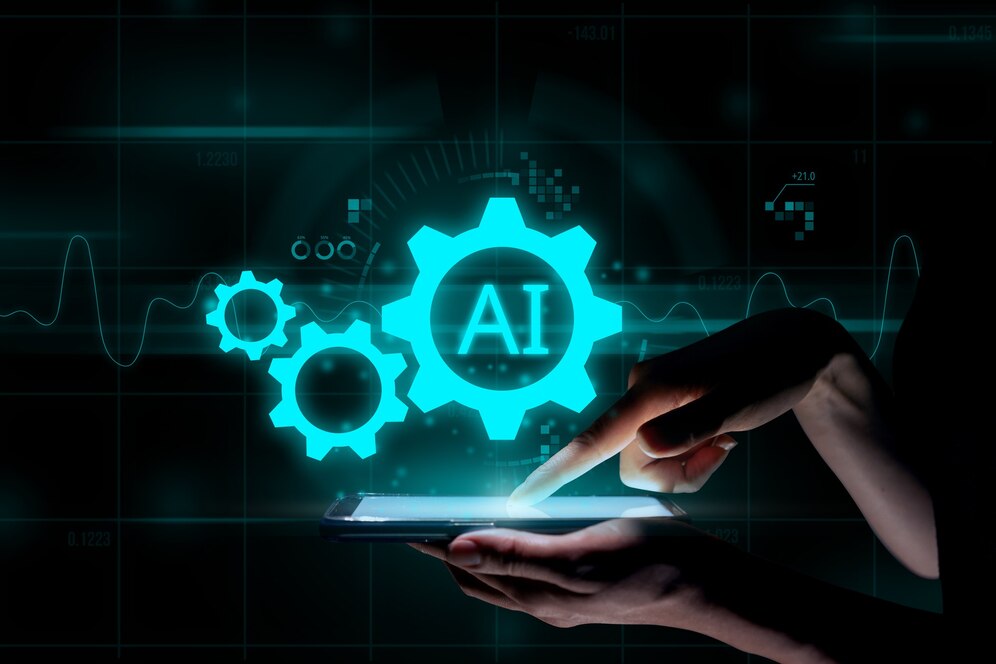
Data is messy. It comes from multiple sources, in different formats, with varying degrees of accuracy. Yet, businesses today need seamless access to clean, structured, and actionable data to make informed decisions. Traditional data integration approaches, while effective, often struggle to keep up with the sheer volume and complexity of modern datasets. Enter Generative AI (GenAI)—a game-changer in the realm of AI data integration, promising automation, efficiency, and intelligence beyond conventional methods.
The Challenge of Data Integration
Businesses rely on diverse data streams: customer interactions, IoT sensors, financial transactions, social media, and more. However, these datasets often exist in silos, making it difficult to unify and analyze them effectively. Traditional Extract, Transform, Load (ETL) pipelines and API-driven integration tools have limitations in handling unstructured or semi-structured data. They require extensive rule-based configurations and manual interventions.
The result? Slow processes, inconsistent data quality, and an inability to respond to real-time changes—issues that AI data integration powered by GenAI aims to solve.
How GenAI Transforms AI Data Integration?
Generative AI brings a new dimension to data integration by learning from existing datasets and intelligently automating the process of data mapping, transformation, and standardization. Here’s how GenAI is reshaping the landscape:
1. Automated Data Mapping and Schema Matching
Traditional integration tools require predefined schemas, making it difficult to merge data from different sources dynamically. GenAI can analyze multiple datasets, understand their structure, and automatically map relationships between fields, even when the naming conventions and formats differ. This eliminates much of the manual effort involved in schema matching and speeds up integration workflows.
2. Intelligent Data Cleansing and Standardization
Data inconsistency is a major challenge—missing values, duplicate records, and formatting differences can severely impact decision-making. GenAI can identify anomalies, suggest corrections, and standardize data across systems. Unlike rule-based engines, it continuously learns and improves, making its recommendations increasingly accurate over time.
3. Context-Aware Data Transformation
Every business has unique data processing rules. GenAI can understand business context by analyzing historical data transformations and applying adaptive learning to automate these processes. Whether it’s converting currencies, normalizing addresses, or reformatting dates, GenAI can perform these tasks without hardcoded logic, making AI data integration more fluid and scalable.
4. Handling Unstructured and Semi-Structured Data
Text documents, emails, images, PDFs, and even video content contain valuable business insights. Unlike traditional integration tools that struggle with such data, GenAI can extract relevant information, tag metadata, and structure it for downstream analysis. This opens up new possibilities for businesses looking to leverage all available data, not just structured records.
5. Real-Time and Predictive Integration
GenAI’s ability to process data in real-time allows businesses to make faster, more informed decisions. By continuously learning from incoming data streams, it can also predict integration challenges before they occur, proactively suggesting corrective actions. This predictive capability helps organizations maintain high data quality and prevent integration bottlenecks.
Use Cases of GenAI in AI Data Integration
The impact of GenAI-driven integration is already visible across industries. Here are some real-world scenarios where it’s making a difference:
1. Financial Services: Fraud Detection and Risk Management
Banks and financial institutions deal with massive volumes of transactional data from multiple sources. GenAI helps by integrating data from payment systems, customer profiles, and external risk databases, ensuring that fraudulent activities are detected in real time.
2. Healthcare: Patient Data Unification
Healthcare providers often struggle with fragmented patient records across hospitals, clinics, and insurance providers. GenAI can integrate and standardize Electronic Health Records (EHRs), enabling a comprehensive patient view and improving treatment decisions.
3. Retail: Personalized Customer Experiences
Retailers collect customer data from online and offline interactions. GenAI allows seamless integration of purchase history, browsing behavior, and feedback from multiple channels, enabling hyper-personalized marketing strategies.
4. Manufacturing: IoT Data Fusion
Factories and smart supply chains rely on IoT sensors for operational efficiency. GenAI automates the integration of sensor data with enterprise systems, ensuring predictive maintenance, optimizing workflows, and reducing downtime.
The Road Ahead: Challenges and Considerations
While GenAI is a powerful tool in AI data integration, its adoption comes with challenges:
Data Privacy & Compliance: AI-driven integration must adhere to regulations like GDPR and HIPAA, ensuring that sensitive data is handled responsibly.
Bias & Ethical Considerations: Since GenAI learns from historical data, it’s important to monitor for biases that could affect decision-making.
Scalability & Performance: Organizations must ensure their infrastructure can support AI-driven integration at scale without compromising performance.
Final Thoughts: Is GenAI the Future of AI Data Integration?
The ability of GenAI to automate, optimize, and enhance data integration processes is undeniable. As businesses strive for more agility in data management, GenAI will continue to evolve, offering smarter and faster ways to bridge data silos. The key to successful adoption lies in a strategic approach—leveraging GenAI’s strengths while addressing its challenges responsibly.
AI data integration is no longer just about moving data from one system to another; it’s about making data work smarter for businesses. With GenAI in the mix, enterprises can expect a future where data integration is not just automated, but intelligent, adaptive, and proactive. And that’s a leap worth taking.
Subscribe to my newsletter
Read articles from Pritesh Patel directly inside your inbox. Subscribe to the newsletter, and don't miss out.
Written by

Pritesh Patel
Pritesh Patel
Pritesh is a blogger and tech enthusiast. He likes sharing his knowledge in a wide range of domains ranging from AI, data science, emerging technologies, and much more. His work is featured in several authoritative tech publications.