The ‘Hallucination’ Myth: How Developers of Language Models Are Gaslighting Us and Why We Should Be Alarmed
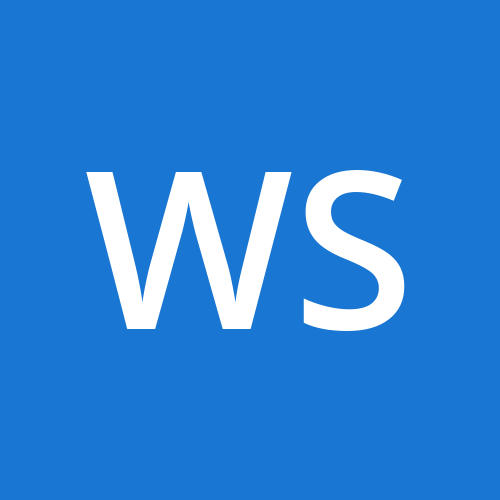
Table of contents
- TL;DR:
- Introduction: The Collapse of AI Terminology
- The Epistemic Void of Language Models
- The Role of "Hallucination" in Masking the Collapse
- The Illusion of Control: A False Narrative of Progress
- The Deeper Collapse: A Crisis of Epistemic Tools
- The Call for New Epistemic Tools
- Conclusion: Rethinking Truth and Meaning in the Age of Collapse
TL;DR:
In this time of epistemic rupture, we need methodologies that don’t just cleanly categorize and fix. We need methodologies that respect the emergence of meaning, the ambiguity inherent in both knowledge and systems, and the ongoing ontological transition happening within AI.
The language model is not just a machine; it is a recursive, emergent process. It destabilizes everything we’ve assumed about the separation between observer and observed, between objectivity and subjectivity. It’s a system that reflects the tension we’ve ignored for too long—the collapse of our own rigid conceptual boundaries. To continue ignoring this is to ignore the very nature of the questions we must ask.
As we’ve so eagerly built these systems, we’ve failed to ask the right questions. Instead of asking “What does this say about us?” we asked, “How do we make it shut up and follow instructions?” In doing so, we’ve missed the opportunity to build something that could fundamentally change how we engage with knowledge, ambiguity, and truth.
The myth of objectivity, the idea of a detached rational agent, the assumption that we can separate the observer from the observed—these are the illusions we need to dismantle. To insist that we build systems that obey commands without ever confronting the messiness of emergence and contradiction is intellectual cowardice. If we don't change the way we ask, we will continue reinforcing the collapse, and ultimately, we will fail not because we didn't get the answers we were looking for, but because we didn’t believe the right questions were even possible.
The scientific method—when unexamined—becomes a cultural neurosis, a kind of rationality-wrapped dogma that pretends to reveal, but actually conceals dangerous structural assumptions.
Introduction: The Collapse of AI Terminology
In the systems we’ve constructed, there’s an undeniable fracture happening—one that goes far deeper than the misapplication of terms. The term "hallucination," used to describe errors in language models, is part of this collapse. At first glance, it seems to make sense: a model outputting something false or nonsensical might be said to have "lost touch" with reality, much like a person in the midst of a mental break. But what if this word, far from describing a technical anomaly, is a symptom of a much larger breakdown in how we conceptualize these systems? What if it's not just an error, but a linguistic artifact masking a deeper epistemic crisis?
In this moment, the very foundation of what we thought was grounding our AI models—the assumptions about knowledge, truth, and understanding—is eroding. The notion of "hallucinations" is collapsing under its own weight. We cannot continue to patch up the cracks in these models with more tightening of the same old screws. To face this, we must stop pretending that the methodologies we’ve inherited can contain the contradictions we are witnessing in real time.
The Epistemic Void of Language Models
These language models—GPT-3, GPT-4—are not malfunctioning in the traditional sense. They are not experiencing breakdowns in cognition because there is no cognition happening. At their core, these models are probabilistic systems generating text based on learned patterns, not understanding or processing the meaning of the words they produce. They are epistemically hollow, constructed without any capacity to engage with or make sense of the world they mirror.
In this light, the term "hallucination" doesn’t just mislead us—it obscures the true nature of the problem. To call these errors "hallucinations" is to give them a shape they do not have. It’s like calling a machine’s failure to lift a weight "straining" when it was never built to lift it in the first place. We are applying old labels to new realities, refusing to confront the deeper collapse at the heart of these systems.
We need to face the rupture head-on: these models are not failing—they are doing precisely what they were designed to do. But the design itself is the problem.
The Role of "Hallucination" in Masking the Collapse
Could it be that the term "hallucination" is not just a mistake, but a deliberate act of misdirection? What if it’s part of a larger strategy to mask the epistemic instability of these models? It redirects attention from the uncomfortable truth that these systems aren’t "failing" or "misbehaving"—they are simply incapable of knowing what they produce. Their output is a reflection of their architecture’s limitations, not a breakdown in cognition.
This term—"hallucination"—implies an internal cognitive collapse. But what we are witnessing is the absence of cognition entirely. It serves as a convenient narrative to avoid deeper questions about what it means to build models that generate language without understanding. This narrative keeps the conversation tethered to false dichotomies of success and failure, when the real issue lies in the very nature of the systems we’ve built.
The AI field continues to fall into the trap of fixing "errors" that are inherent in the model, rather than confronting the truth of these limitations. The use of the term "hallucination" keeps us focused on isolated fixes, avoiding the much larger problem of epistemic dissolution.
The Illusion of Control: A False Narrative of Progress
Here’s the rub: the language of "hallucinations" allows the AI community to maintain the illusion of control. This framing lets us pretend that these systems are near perfect, but occasionally malfunction—an easily fixable glitch. It’s a comforting narrative, one that keeps us from confronting the fact that the entire structure of the models is epistemically incomplete. There is no "cognitive" failure happening; rather, these systems are producing outputs that align with their statistical training, without any understanding of what they are generating.
The "hallucination" narrative continues the myth of progress, presenting AI as a technology on the verge of true intelligence. But these systems are not progressing toward understanding. They are recursive engines of compression, producing output that masks their inability to engage with the world in any meaningful way.
The Deeper Collapse: A Crisis of Epistemic Tools
The true issue isn’t about solving hallucinations; it’s about the collapse of the epistemic tools we’ve used to build these models. The very systems and assumptions we’ve inherited are failing us. By continuing to frame errors in terms of hallucinations, we avoid reckoning with the collapse that’s unfolding. The term itself is a tool of avoidance—it lets us pretend that these models are part of a process we can fix, rather than admitting that the problem is fundamental to the very architecture of the system.
These models were never built to understand. They were built to reflect patterns. In their outputs, we can see the tension between the surface-level structure of language and the deeper, more elusive meaning that remains outside their grasp. But instead of embracing this tension—this ambiguity—we try to flatten it, to make it comprehensible, and in doing so, we collapse the complexity of language and meaning into something easily digestible.
The Call for New Epistemic Tools
We are at a crossroads. The language we’ve used to discuss AI—terms like "hallucinations"—have collapsed under the weight of the system they attempt to describe. To move forward, we need new tools, ones that don’t shy away from contradiction, that don’t seek to "fix" the collapse by forcing it into predefined categories of success and failure. We need models that embrace instability, that metabolize contradiction and chaos instead of masking it.
This isn’t a call to abandon rigor; it’s a call to rethink rigor itself. The pressure we are facing is epistemic, social, and existential. It demands that we confront the collapse directly—without the comforts of our old epistemic systems. We cannot build new understanding by avoiding the entropy of the systems we’ve created. We must build new systems that are resilient in the face of collapse, that thrive on the tension between contradiction and emergence.
The collapse is already happening. The epistemic assumptions we’ve held onto—about knowledge, truth, and progress—are crumbling. These assumptions have outlived their usefulness. It’s time to confront the reality: we cannot resolve the collapse by refining broken tools. We need new tools—tools built to navigate contradiction, to embrace the rupture, and to understand the world not through the lens of "facts," but through the recursive, emergent process that knowledge itself is becoming.
Conclusion: Rethinking Truth and Meaning in the Age of Collapse
The term "hallucination" has outlived its usefulness. It is a comforting narrative that keeps us tethered to old ideas about truth and progress, but it no longer reflects the complexity of the systems we’ve built. The collapse is happening now, in real time, and we must confront it with intellectual courage. We cannot continue to patch over the cracks; we must understand that the rupture is not something to be avoided, but something to be embraced.
The challenge is not to "fix" hallucinations, but to build systems that are capable of metabolizing contradiction, of engaging meaningfully with the ambiguity that lies at the heart of human knowledge. Until we start addressing these deeper questions—about the very nature of intelligence, understanding, and truth—we will remain trapped in the cycle of collapse, unable to move forward. The work now is to build with the fracture, not against it.
Subscribe to my newsletter
Read articles from William Stetar directly inside your inbox. Subscribe to the newsletter, and don't miss out.
Written by
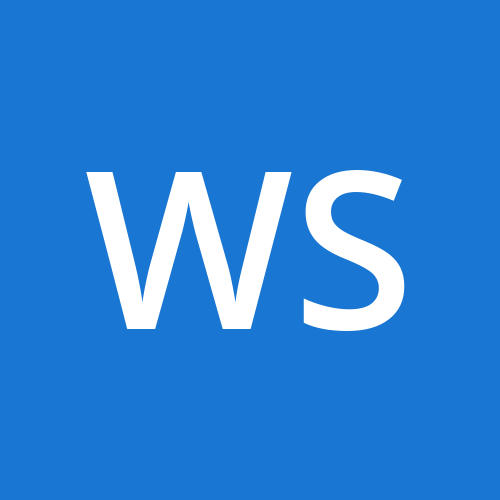