Building Responsible Generative AI
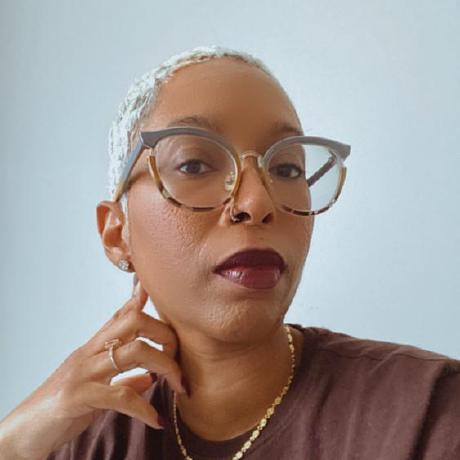
Abstract
Generative AI is rapidly transforming industries by providing innovative solutions for content creation, customer service, education, and more. However, as these technologies become more widespread, issues of bias and exclusion have emerged. This white paper outlines best practices for inclusive prompt writing that can help mitigate bias and promote equitable outputs across generative AI systems. Drawing from practical experience in prompt writing, quality assurance, and collaboration with diverse teams, these guidelines intend to enhance inclusivity in AI-generated content.
Introduction
Generative AI models have become powerful tools in various industries, ranging from creative writing to customer service automation. As these technologies evolve, so do concerns about the fairness and inclusivity of their outputs. In particular, biased prompts can result in harmful or exclusionary results, which undermine both the ethical and practical value of AI systems (Bender et al., 2021).
Inclusive prompt writing addresses these concerns by providing structured guidelines for crafting prompts that are neutral, culturally aware, and responsive to diverse perspectives. With my experience in mentoring prompt writers, conducting quality assurance reviews, and collaborating with cross-functional teams, I seek to provide actionable recommendations for improving inclusivity in generative AI (Google Developers, n.d.).
Problem Statement
Generative AI systems are only as good as the data and instructions provided to them. Biased prompts, whether crafted consciously or unconsciously, can lead to outputs that perpetuate stereotypes, exclude certain groups, or misrepresent cultural nuances (Noble, 2018). Such biases may manifest across various dimensions, including race, gender, religion, socioeconomic status, and more.
The absence of standardized guidelines for inclusive prompt writing exacerbates these issues, as developers and prompt writers may lack the tools or awareness needed to ensure equity in AI outputs. Addressing this gap requires a proactive approach that emphasizes inclusivity from the ground up (Bender & Friedman, 2018).
Proposed Solution: Best Practices for Inclusive Prompt Writing
Creating Neutral and Inclusive Prompts
Avoid biased language and assumptions (Google Developers, n.d.).
Ensure cultural sensitivity by considering the diverse backgrounds of end-users (Benjamin, 2019).
Use neutral wording that does not imply negative or stereotypical traits.
Collaborating with Diverse Teams
Involve individuals from various backgrounds in prompt writing and review processes.
Encourage open dialogue about inclusivity and bias during content creation.
Provide mentorship opportunities for underrepresented groups in AI development (Mitchell et al., 2019).
Testing for Bias and Refining Prompts
Continuously monitor outputs for signs of bias (IBM Research, n.d.).
Gather feedback from diverse users to improve inclusivity.
Regularly update prompts based on new insights and findings (OpenAI, n.d.).
Documentation and Guidelines
Develop clear, accessible standards for prompt writing that emphasize inclusivity (Bender & Friedman, 2018).
Maintain consistency in guidelines across teams and projects.
Provide training resources for prompt writers and reviewers (Hugging Face, n.d.).
Implementation Framework
Integrating these best practices into generative AI workflows requires intentional planning and collaboration. Teams can utilize tools such as embeddings and vector stores to improve content diversity and reduce biases. Additionally, establishing QA feedback loops can enhance prompt quality and inclusivity over time (Partnership on AI, n.d.).
Conclusion
Inclusive prompt writing is essential for developing ethical and effective generative AI systems. By implementing these best practices, developers, companies, and content creators can create more equitable technologies that serve a broader range of users. Ensuring inclusivity is an ongoing process that demands vigilance, collaboration, and a commitment to continuous improvement.
References
Bender, E. M., Gebru, T., McMillan-Major, A., & Shmitchell, S. (2021). On the Dangers of Stochastic Parrots: Can Language Models Be Too Big? Proceedings of the 2021 ACM Conference on Fairness, Accountability, and Transparency. https://doi.org/10.1145/3442188.3445922
Bender, E. M., & Friedman, B. (2018). Data Statements for Natural Language Processing: Toward Mitigating System Bias and Enabling Better Science. Transactions of the Association for Computational Linguistics, 6, 587–604. https://aclanthology.org/Q18-1041/
Google Developers. (n.d.). Inclusive Language in Technical Writing. https://developers.google.com/style/inclusive-documentation
OpenAI. (n.d.). Research Publications on Bias and Fairness. https://openai.com/research
Hugging Face. (n.d.). Ethics and Bias Documentation. https://huggingface.co/docs/transformers/index
Benjamin, R. (2019). Race After Technology: Abolitionist Tools for the New Jim Code. Polity Press.
Noble, S. U. (2018). Algorithms of Oppression: How Search Engines Reinforce Racism. NYU Press.
IBM Research. (n.d.). AI Fairness 360 Open Source Toolkit. https://aif360.mybluemix.net/
Partnership on AI. (n.d.). AI Incident Database. https://incidentdatabase.ai/
Mitchell, M., et al. (2019). Model Cards for Model Reporting. Proceedings of the Conference on Fairness, Accountability, and Transparency. https://modelcards.withgoogle.com/about
Subscribe to my newsletter
Read articles from Jo Sesay directly inside your inbox. Subscribe to the newsletter, and don't miss out.
Written by
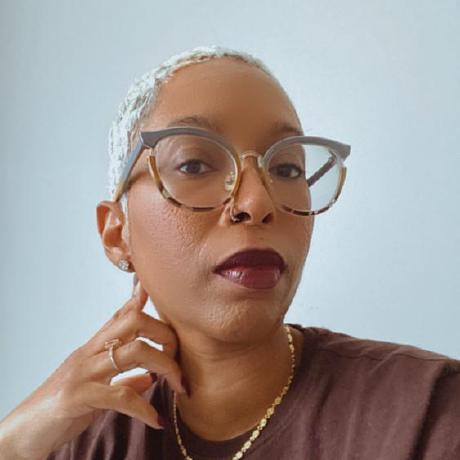
Jo Sesay
Jo Sesay
I’m Jo, a passionate full-stack developer specializing in machine learning and AI. With a background in tech editing and a strong foundation in JavaScript and Python, I thrive at the intersection of creativity and technology. My mission is to build innovative solutions that empower others. Welcome to my portfolio.