Cluster Analysis Techniques in SPSS for Segmentation Studies
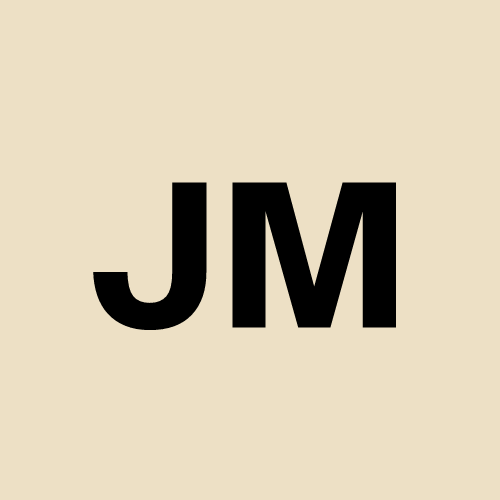

Ever wonder how marketers figure out exactly who to target with their flashy ads? Or how researchers group similar folks together in a study without just guessing? That’s where cluster analysis steps in. It’s one of those behind-the-scenes tools that doesn’t get enough love, but it’s a game changer when it comes to understanding patterns in data. And yep—you can totally do it in SPSS without having to be some math wizard.
In this article, we’re gonna dig into what cluster analysis is, why it matters for segmentation, and how to actually pull it off in SPSS without breaking a sweat. We’ll also talk about the different techniques you can use, how to make sense of the results, and throw in a few real-life examples and pro tips along the way.
Let’s roll.
So What Is Cluster Analysis?
Cluster analysis is basically a way to group stuff—people, products, behaviors, whatever—based on how similar they are. Think of it like this: if you dumped all your mixed socks in a pile and started grouping them by color or pattern, congrats, you’re doing a cluster analysis (sorta).
In research and marketing, it’s all about segmentation. You take a big ol’ messy dataset and sort it into neat lil’ groups (clusters) that have shared traits. That’s helpful when you wanna:
Identify customer segments in business
Find personality types in psychology
Categorize geographic areas in social studies
Analyze behavior patterns in public health
And SPSS? Yeah, it’s totally built to handle this kinda stuff.
Different Types of Cluster Analysis in SPSS
Now, SPSS doesn’t just give you one option for clustering—it’s got a couple tricks up its sleeve. Each method’s got its pros and cons, and honestly, it kinda depends on your data and what you’re trying to figure out.
1. Hierarchical Cluster Analysis
This method builds a tree-like structure called a dendrogram. It starts by treating every single case as its own cluster, then gradually merges 'em based on similarity until you’ve got one big cluster.
Good for smaller datasets (like under 100 cases)
You get a visual idea of how cases are grouped
Can be slow with big data though
2. K-Means Cluster Analysis
This one's more common when you already know how many clusters you want. You tell SPSS, “Hey, I want 3 clusters,” and it works out the best way to assign cases into those 3 groups.
Great for larger datasets
Quick and efficient
But yeah, you gotta decide the number of clusters first (not always easy)
3. TwoStep Cluster Analysis
This one’s newer and more automated. It’s super helpful when you’ve got a mix of numerical and categorical data.
Handles big data like a champ
Automatically suggests the optimal number of clusters
Bonus: You don’t have to do a ton of prep work
Getting Started: Preparing Your Data
Alright, before you dive into clustering, you gotta make sure your data’s clean. Like, not squeaky clean, but clean enough that the results aren’t garbage.
Here’s a few prep steps:
Standardize your variables. If one variable is measured in dollars and another is just a 1–5 rating scale, SPSS might treat the dollar variable like it matters more. Standardizing levels the playing field.
Handle missing data. You don’t wanna run a cluster analysis with a bunch of blanks. Either fill 'em in, drop those cases, or use some fancy imputation methods.
Remove outliers. Just trust me—outliers can mess up clustering bad.
Once your data’s lookin’ solid, it’s time to open up SPSS and get to work.
Running Cluster Analysis in SPSS
Let’s walk through how you’d actually do this in SPSS, step by step.
A. For Hierarchical Cluster Analysis
Go to
Analyze > Classify > Hierarchical Cluster
Toss your variables into the “Variables” box
Pick a clustering method (Ward’s is usually a safe bet)
Choose a distance measure (Euclidean distance is standard)
Hit OK and check out your dendrogram!
B. For K-Means Cluster Analysis
Head to
Analyze > Classify > K-Means Cluster
Pick your variables
Choose the number of clusters (e.g., 3)
Let SPSS run its magic
Review the cluster centers and ANOVA tables
C. For TwoStep Cluster Analysis
Go to
Analyze > Classify > TwoStep Cluster
Choose your variables (mix of categorical and scale works)
Select whether you want the number of clusters auto-detected or set it yourself
Click “OK” and let the algorithm do its thing
Boom. You just clustered.
Interpreting the Results
Okay, now you’ve got some output—but what does it even mean?
Cluster membership tells you which case belongs to which cluster
Cluster centers show the average values for each variable within each cluster (super useful for naming the clusters, like “High Spenders” or “Low Risk Users”)
Distances or “final cluster centers” help you figure out how different the clusters really are
Here’s where the real fun starts: translating these clusters into actual insights.
Maybe Cluster 1 is your budget shoppers, Cluster 2 are your trend chasers, and Cluster 3 are loyal repeat customers. Once you’ve got that down, you can target each group differently or dig deeper into their habits.
Real-World Example
Let’s say you’re working on a thesis about social media usage and mental health. You surveyed 200 people on variables like hours per day on social apps, self-esteem scores, anxiety levels, and sleep quality.
Cluster analysis could help you identify natural groupings like:
Cluster A: High usage, high anxiety, poor sleep
Cluster B: Moderate usage, decent self-esteem, okay sleep
Cluster C: Low usage, low anxiety, high sleep quality
Boom. You’ve got meaningful segments that could totally help guide intervention strategies or further studies.
Oh—and if all this sounds like a lot to handle on your own, getting SPSS Homework Help can make a huge difference. Whether you’re stuck with interpretation, or just need someone to double-check your clustering setup, it’s a lifesaver in the middle of a data crisis.
Pitfalls to Avoid
Quick heads up: cluster analysis isn’t always sunshine and rainbows. There’s a few things you’ll wanna watch out for:
Over-interpreting clusters – Just 'cause SPSS grouped your data doesn’t mean the clusters are always meaningful. Use your brain and domain knowledge.
Too many variables – If you use 20 variables, the clusters may become noisy. Stick to the key ones.
Guessing the number of clusters – Especially with K-means, it helps to try a few different cluster counts and compare the results.
And remember: cluster analysis is exploratory. It's not like a hypothesis test. It helps you see structure in the data, not prove causation.
Final Thoughts
Cluster analysis in SPSS is one of those underrated tools that can make your research, marketing strategy, or thesis stand out. It’s flexible, powerful, and honestly not that hard once you’ve tried it a couple times. Whether you’re using K-Means, Hierarchical, or TwoStep clustering, you’ve got options—and SPSS makes it all pretty manageable.
Just make sure your data’s clean, your variables are solid, and your interpretation’s thoughtful. Don’t be afraid to experiment with different techniques, and if you’re deep in the weeds, reach out for SPSS Homework Help to save yourself a late-night stats meltdown.
Subscribe to my newsletter
Read articles from Jones Miller directly inside your inbox. Subscribe to the newsletter, and don't miss out.
Written by
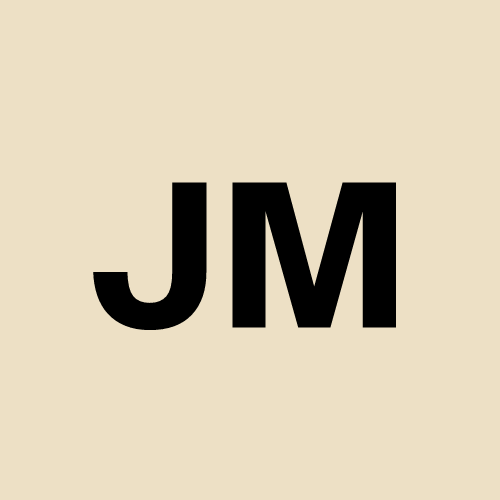