Fine-Tuning Generative Models on the Cloud: A Guide for Developers
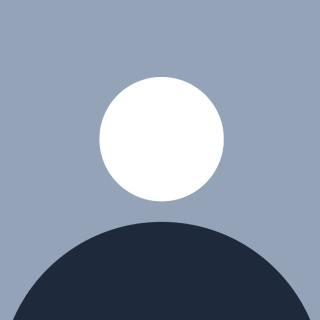

Generative AI models, such as GPT and other large language models (LLMs), have transformed industries by enabling applications in text generation, summarization, translation, and more. However, to maximize their potential for specific tasks, fine-tuning is essential. Cloud platforms provide scalable infrastructure and GPU-powered computing that simplify the process for developers.
This guide will explore how to fine-tune generative models on the cloud, highlight the best cloud services for LLM fine-tuning, and provide a step-by-step guide to fine-tuning AI models. It also covers techniques such as transfer learning for generative AI, optimizing cloud GPU usage, deploying custom solutions, and more.
Best Cloud Services for LLM Fine-Tuning
Cloud platforms offer specialized services for fine-tuning large language models. Below is a comparison of the top providers:
Cloud Platform | Key Features | Supported Models |
NeevCloud | Optimized GPU instances; pre-configured environments; cost-effective scaling | GPT-4, LLaMA, Falcon |
Google Vertex AI | Managed pipelines; hyperparameter tuning; integration with open-source models | Gemini 2.0 Flash |
Oracle Cloud (OCI) | Parameter-efficient tuning with T-Few; cost-effective GPU instances | Custom LLMs |
Azure Machine Learning | Integration with OpenAI APIs; automated workflows for fine-tuning | GPT-4, DALL-E |
AWS SageMaker | Distributed training with Hugging Face; Spot Instances for cost savings | Jurassic-2, Titan |
Why Choose NeevCloud?
NeevCloud stands out due to its focus on developer-friendly tools and optimized GPU configurations tailored for efficient model training. Its cost-effective scaling makes it an excellent choice for startups and enterprises alike.
Step-by-Step Guide to Fine-Tuning AI Models
Fine-tuning generative models on the cloud involves several steps. Let’s break it down:
Step 1: Prepare Your Dataset
A well-prepared dataset is critical for successful fine-tuning.
Guidelines:
Format your data in JSONL (JSON Lines) format:
json
{"input": "What is AI?", "output": "AI stands for Artificial Intelligence."}
Split your dataset into:
Training Set (80%)
Validation Set (20%)
Upload the data to cloud storage (e.g., NeevCloud Storage or AWS S3).
Example Use Case:
If you’re fine-tuning a chatbot for customer support, your dataset might include real customer queries and their corresponding responses.
Step 2: Choose a Pre-Trained Model
Select a pre-trained model that aligns with your task requirements. For example:
Use GPT-based models for text generation tasks.
Opt for Stable Diffusion or DALL-E for image generation.
Open-source alternatives like LLaMA or Falcon are excellent choices if you want more control over the model architecture.
Step 3: Configure Training Parameters
Set up hyperparameters such as:
Learning Rate: Start with a small value (e.g., 1e-5) to avoid overfitting.
Batch Size: Larger batch sizes speed up training but require more GPU memory.
Epochs: Typically 3–5 epochs are sufficient for most tasks.
Example Code Snippet (NeevCloud):
python
from neevcloud.tuning import sft
sft_tuning_job = sft.train(
source_model="gpt-4",
train_dataset="neevcloud://bucket/sft_train.jsonl",
validation_dataset="neevcloud://bucket/sft_validate.jsonl",
epochs=5,
adapter_size=128,
learning_rate_multiplier=1.0
)
Step 4: Train Your Model on Cloud GPUs
Training on GPUs significantly accelerates the process. Use distributed training if working with large datasets or models.
GPU Options:
NVIDIA A100 (40 GB VRAM): Ideal for large-scale LLMs.
NVIDIA H100 (80 GB VRAM): Best for cutting-edge performance.
Cost Optimization Tips:
Use Spot Instances: Save up to 70% compared to on-demand instances.
Enable Mixed Precision Training (fp16/bf16): Reduces memory usage without compromising accuracy.
Step 5: Monitor Training Metrics
Track key metrics such as:
Training Loss
Validation Accuracy
GPU Utilization
Most cloud platforms provide dashboards to visualize these metrics in real time.
Example Graph:
Below is an example graph showing how distributed training reduces time:
Training Time vs GPU Configuration
GPUs | Epochs | Time (hours) |
1 | 5 | 12.5 |
4 | 5 | 3.8 |
8 | 5 | 2.1 |
Distributed training on 8 GPUs cuts time by 83% compared to single-GPU setups.
Step 6: Deploy the Fine-Tuned Model
Once training is complete, deploy your model as an API endpoint using managed services like NeevCloud Endpoints or AWS SageMaker Hosting.
Deployment Checklist:
Optimize the model using techniques like quantization or pruning.
Test latency and throughput under real-world conditions.
Secure your endpoint with authentication mechanisms (e.g., API keys).
Fine-Tuning Open-Source Models on Cloud Platforms
Open-source models like LLaMA, Falcon, and BLOOM are gaining popularity due to their flexibility and cost-effectiveness. Here’s how you can fine-tune them:
Download the model weights from repositories like Hugging Face.
Use frameworks like PyTorch or TensorFlow for customization.
Train on cloud GPUs using distributed strategies like data parallelism.
Example Frameworks:
Hugging Face Transformers
DeepSpeed (for memory-efficient training)
PyTorch Lightning
Transfer Learning in Generative AI
Transfer learning enables developers to adapt pre-trained models to new tasks without retraining from scratch. This approach is particularly useful when working with limited datasets.
Techniques:
Freezing Layers: Retain base layers of the model while retraining only task-specific layers.
Low-Rank Adaptation (LoRA): Update only a small subset of parameters to reduce computational overhead.
Prompt Engineering: Modify input prompts instead of altering model weights.
Efficient Model Training with Cloud GPUs
Cloud GPUs are essential for fine-tuning large-scale generative models efficiently.
Benefits of Using Cloud GPUs:
Faster Training Times: Parallel processing accelerates computation.
Cost Savings: Spot instances and auto-scaling reduce expenses.
Flexibility: Easily switch between different GPU types based on workload requirements.
Best Practices:
Use gradient checkpointing to save memory during backpropagation.
Enable mixed precision training (fp16/bf16) to optimize performance without sacrificing accuracy.
Monitor GPU utilization to avoid underutilization or bottlenecks.
Cloud Infrastructure for Machine Learning
The underlying cloud infrastructure plays a crucial role in enabling seamless model fine-tuning and deployment.
Key Components:
Compute Instances: High-performance VMs equipped with GPUs/TPUs.
Storage Solutions: Scalable storage options like S3 buckets or NeevCloud Storage.
Networking Services: Low-latency communication between compute nodes.
By leveraging these components effectively, developers can build robust pipelines for generative AI model training and deployment.
AI Model Optimization Techniques
Optimizing generative AI models ensures better performance and lower resource consumption:
Quantization: Reduce precision from fp32 to int8 or fp16.
Pruning: Remove redundant neurons in neural networks.
Knowledge Distillation: Train smaller models using outputs from larger ones.
These techniques are particularly useful when deploying models in resource-constrained environments.
Conclusion
Fine-tuning generative models on the cloud empowers developers to create customized solutions tailored to specific use cases while leveraging scalable infrastructure and cutting-edge hardware like GPUs. By following this guide, you can efficiently prepare datasets, configure training jobs, monitor metrics, and deploy optimized models using platforms like NeevCloud, Google Vertex AI, AWS SageMaker, Azure ML, and Oracle Cloud Infrastructure.
Whether you're working with open-source models or proprietary solutions like GPT-based systems, adopting best practices in transfer learning, cost optimization, and deployment will ensure success in building high-performance applications powered by generative AI.
Start your journey today by exploring cloud-based tools and services that simplify the complex process of AI model fine-tuning!
Subscribe to my newsletter
Read articles from Tanvi Ausare directly inside your inbox. Subscribe to the newsletter, and don't miss out.
Written by
Tanvi Ausare
Tanvi Ausare
Digital Marketer & Technical Writer at NeevCloud, India’s AI First SuperCloud company. I write at the intersection of technology, cloud computing, and AI, distilling complex infrastructure into real, relatable insights for builders, startups, and enterprises. With a strong focus on tech marketing, I simplify technical narratives and shape strategies that connect products to people. My work spans cloud-native trends, AI infra evolution, product storytelling, and actionable guides for navigating the fast-moving cloud landscape.