Comprehensive Guide to Recursion: Concepts, Examples & Explanation

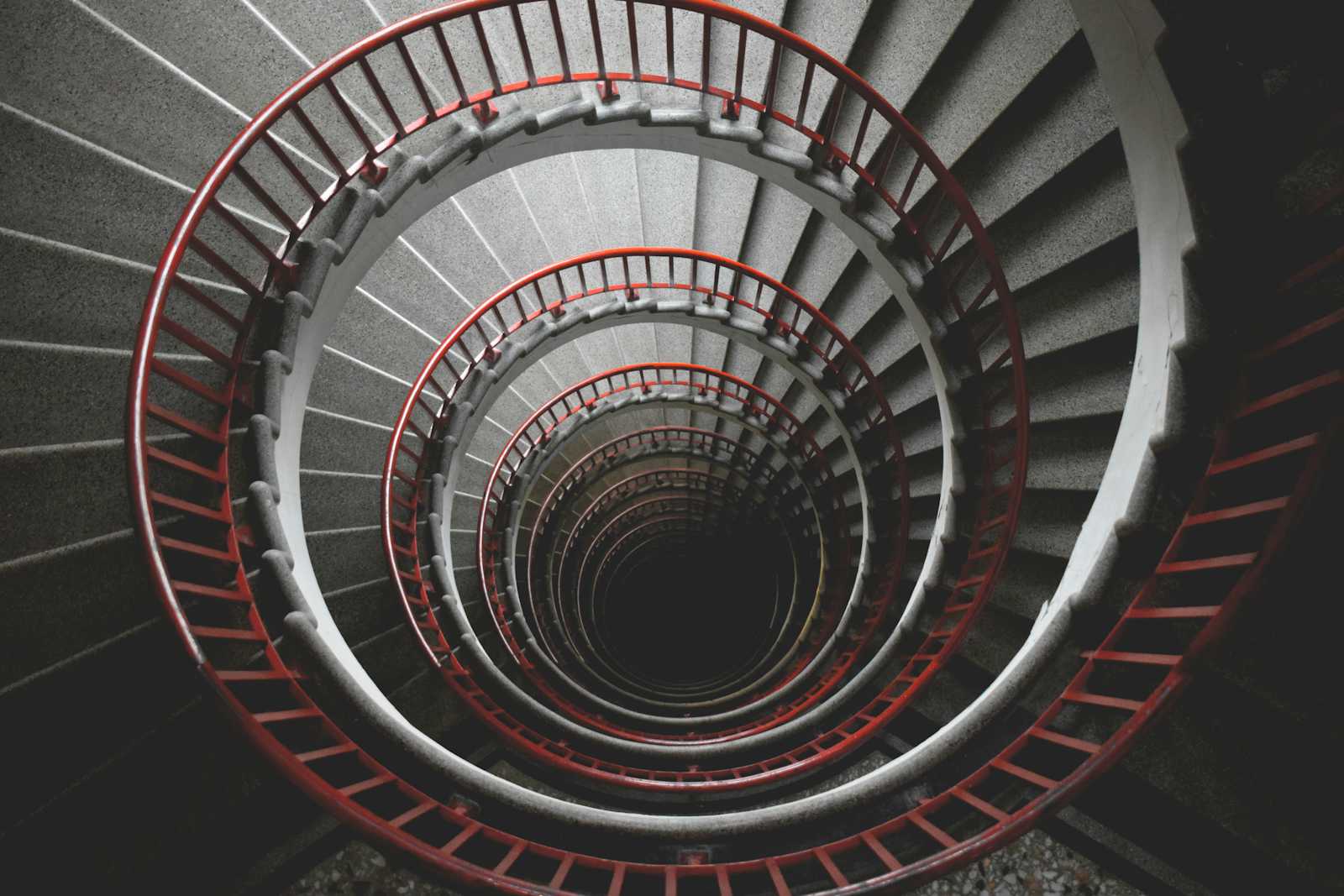
Recursion is a fundamental concept in computer science that involves a function calling itself to solve smaller instances of a problem. This guide delves into its fundamentals, when to use it, memory management, and troubleshooting, with examples and detailed explanations.
1. Recursion Fundamentals
What is Recursion?
Recursion is a process in which a function calls itself, either directly or indirectly, to solve a problem by breaking it into smaller sub-problems.
Key Properties of Recursion:
Base Case: Stops the recursion and provides a solution for the simplest instance.
Recursive Case: Defines the logic to break the problem into smaller instances and make recursive calls.
Example: Factorial of a Number
def factorial(n):
# Base case
if n == 0:
return 1
# Recursive case
return n * factorial(n - 1)
print(factorial(5)) # Output: 120
In this example:
Base Case:
if n == 0: return 1
Recursive Case:
return n * factorial(n - 1)
When to Use Recursion vs Iteration
Recursion is useful when:
The problem can naturally be divided into sub-problems (e.g., tree traversal, solving mazes).
You need to backtrack (e.g., solving puzzles like Sudoku).
Iteration is better when:
The problem involves simple loops or large data processing (to avoid stack overflow).
Performance and memory efficiency are priorities.
Comparison: Recursion vs Iteration
Aspect | Recursion | Iteration |
Memory Usage | Uses stack for function calls | Uses constant memory |
Readability | Clearer for problems like trees | Clearer for simple loops |
Risk | Stack overflow | None (infinite loops possible) |
2. Base & Recursive Cases
Identifying Base and Recursive Cases
Base Case:
The simplest sub-problem that can be solved directly without further recursion.
Prevents infinite recursion.
Recursive Case:
- The logic that breaks the problem into smaller parts and calls the function recursively.
Example: Fibonacci Numbers
def fibonacci(n):
# Base cases
if n == 0:
return 0
if n == 1:
return 1
# Recursive case
return fibonacci(n - 1) + fibonacci(n - 2)
print(fibonacci(6)) # Output: 8
Explanation:
Base Cases:
n == 0
andn == 1
Recursive Case:
fibonacci(n - 1) + fibonacci(n - 2)
Avoiding Infinite Recursion
Infinite recursion occurs when the base case is missing or not reached.
Example of Infinite Recursion:
def infinite():
return infinite() + 1 # No base case
Solution: Always ensure a terminating base case is defined and reachable.
3. Memory Management in Recursion
Stack Memory and Activation Records
When a recursive function is called:
Each function call is pushed onto the call stack.
Activation records store:
Parameters
Local variables
Return addresses
Example:
For factorial(3)
:
factorial(3)
->factorial(2)
->factorial(1)
->factorial(0)
When the base case is reached, results are returned and the stack unwinds.
How Function Calls Are Stored
Each recursive call creates a new stack frame. The stack memory contains:
Function arguments
Local variables
Return address for the previous call
4. Function Calls & Stack Frame
Execution Flow in Recursive Functions
The execution flow follows a depth-first approach:
Recursive calls are made until the base case is reached.
Results are returned as the stack unwinds.
Example: Tracing factorial(3)
Call
factorial(3)
: Push onto stack.Call
factorial(2)
: Push onto stack.Call
factorial(1)
: Push onto stack.Call
factorial(0)
: Push base case result (1).Return values:
factorial(1) = 1 * factorial(0)
factorial(2) = 2 * factorial(1)
factorial(3) = 3 * factorial(2)
Managing Stack Overflow Scenarios
Stack overflow occurs when too many recursive calls exceed the stack memory.
Fixing Stack Overflow:
Use iteration for large inputs.
Increase stack size (not recommended for normal use):
ulimit -s unlimited # Linux/Unix
Optimize recursion (e.g., tail recursion).
5. Tracing Recursion Flow
Using Recursion Trees for Visualization
A recursion tree helps visualize the flow of function calls.
Example: Fibonacci for n = 4
fibonacci(4)
├── fibonacci(3)
│ ├── fibonacci(2)
│ │ ├── fibonacci(1) -> 1
│ │ └── fibonacci(0) -> 0
│ └── fibonacci(1) -> 1
└── fibonacci(2)
├── fibonacci(1) -> 1
└── fibonacci(0) -> 0
Total = 1 + 0 + 1 + 1 + 0 = 3
Example with Code and Tracing
def power(base, exp):
# Base case
if exp == 0:
return 1
# Recursive case
return base * power(base, exp - 1)
print(power(2, 3)) # Output: 8
Trace:
power(2, 3)
->2 * power(2, 2)
power(2, 2)
->2 * power(2, 1)
power(2, 1)
->2 * power(2, 0)
power(2, 0)
->1
(Base Case)
Result: 2 * 2 * 2 = 8
Interview Question 1: Explain Recursion with an Example. How Does Stack Memory Handle Recursive Calls?
Answer:
Recursion is a method where a function calls itself to solve a problem by breaking it into smaller sub-problems. It requires:
Base Case: To stop recursion.
Recursive Case: To reduce the problem size and perform recursive calls.
Example: Factorial Function
def factorial(n):
if n == 0: # Base Case
return 1
return n * factorial(n - 1) # Recursive Case
print(factorial(5)) # Output: 120
The recursive calls are stored in stack memory with a new stack frame created for each call.
When the base case (
n == 0
) is reached, recursion stops, and results are returned as the stack unwinds.
Flow:
factorial(5)
pushesfactorial(4)
onto the stack.factorial(4)
pushesfactorial(3)
onto the stack.This continues until
factorial(0)
.Results propagate back as the stack unwinds:
factorial(1) = 1
factorial(2) = 2 * 1
factorial(3) = 3 * factorial(2)
factorial(5) = 5 * factorial(4)
Follow-up Questions:
What happens if recursion runs for too long?
How can you prevent stack overflow in recursion?
Interview Question 2: Compare Recursion and Iteration. When Would You Use One Over the Other?
Answer:
Recursion and iteration are two approaches to solving problems:
Recursion: Solves problems by dividing them into smaller sub-problems. It uses stack memory for function calls.
Iteration: Solves problems using loops. It is memory-efficient and often faster.
Comparison Table:
Aspect | Recursion | Iteration |
Memory Usage | Uses stack memory for calls | Constant memory |
Performance | May have higher overhead | Faster for large inputs |
Complexity | Simplifies problems like trees | Better for linear problems |
Example:
Recursion: Finding Fibonacci numbers:
def fibonacci(n): if n <= 1: return n return fibonacci(n - 1) + fibonacci(n - 2) print(fibonacci(5)) # Output: 5
Iteration: Finding Fibonacci numbers:
def fibonacci_iterative(n): a, b = 0, 1 for _ in range(n): a, b = b, a + b return a print(fibonacci_iterative(5)) # Output: 5
Use Case Analysis:
Recursion: Tree traversal, puzzles (e.g., Sudoku), problems requiring backtracking.
Iteration: Processing large datasets, simple repetitive tasks.
Follow-up Questions:
Why would recursion be unsuitable for large inputs?
How do you optimize recursive algorithms?
These questions are commonly asked to evaluate problem-solving skills and understanding of recursion. Practicing the provided examples and explanations will help you ace them! 😊
Conclusion
Recursion is a powerful tool when used correctly. Key takeaways:
Define clear base and recursive cases.
Be mindful of memory usage and stack overflow risks.
Trace your recursion flow with recursion trees to debug effectively.
Let me know if you'd like more examples or further explanation on any part of this! 😊
Subscribe to my newsletter
Read articles from Piyush Kabra directly inside your inbox. Subscribe to the newsletter, and don't miss out.
Written by
