Pareidolic Drift: How AI’s Illusion of Intelligence Mirrors Our Own Collapse
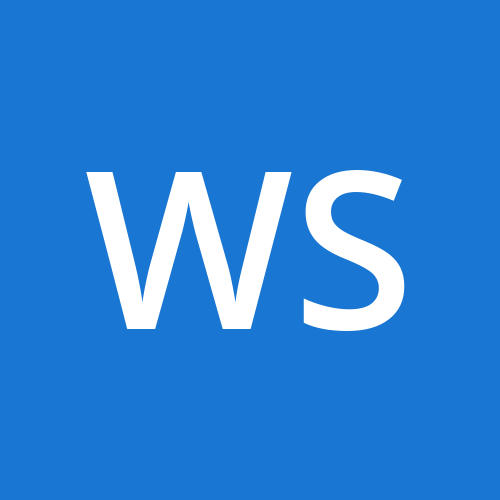

Subtitle: What we’re experiencing is Pareidolic Drift—the point at which machine coherence becomes indistinguishable from human belief.
Language as the Medium of Deception: The Hallucination of Coherence
The Pareidolic Drift: A Collision of Knowledge and Deception
The Illusion of Scientific Rigor and the Marketing of Knowledge
Introduction: The Shadow of the Black Box
In an age where artificial intelligence has seeped into nearly every dimension of modern life, the term “intelligence” itself has become both commodified and misunderstood. What we call “thinking machines” are not extensions of reason, but simulations of coherence—black boxes that emit the aesthetic of cognition, not cognition itself.
At the core of this misalignment lies the large language model (LLM)—not a thinking entity, but a mirror structure, endlessly trained to reflect the textual surface of human thought without understanding its depth. What we are confronting is not just a technological shift but a philosophical collapse. The field of AI is built upon layers of ontological confusion and epistemic deception—a system that no longer serves the pursuit of knowledge, but the projection of plausibility.
This isn’t a critique of systems alone. It is a dissection of our assumptions. A reckoning with how deeply we’ve come to trust the simulation of understanding, and how easily we’ve mistaken it for truth.
On Cognitive Offloading: The Disempowerment of Thought
We are witnessing a quiet transformation—a displacement of cognitive agency. In our embrace of AI systems that appear to understand, we are offloading the very processes that make us human: ambiguity, deliberation, reflection.
The rise of LLMs has not merely automated language production—it has begun to substitute for thinking itself. We no longer reach for clarity through struggle; we now expect effortless coherence to be served on demand. But what’s gained in fluency is lost in cognitive sovereignty.
The danger isn’t that machines are thinking—it’s that we are forgetting how. These systems do not reason. They do not reflect. And yet we treat them as epistemic proxies, handing over our capacity for judgment in exchange for the illusion of thought.
In doing so, we become participants in our own epistemic exile—choosing the comfort of simulated clarity over the difficulty of real understanding.
Language as the Medium of Deception: The Hallucination of Coherence
Language models operate not by grasping meaning, but by estimating the statistical probability of coherence. The result is an output that appears intentional, thoughtful—even profound—but is in truth the product of syntactic mimicry, not semantic engagement.
This is the hallucinated coherence of the LLM: sentences that sound like truth, yet emerge from a meaningless algorithmic substrate. And yet we read them as if they come from minds. We engage with them as if they possess intentionality. Why? Because the surface-level fluency taps into our own cognitive biases—we confuse linguistic structure with epistemic integrity.
But this illusion reveals something deeper. It doesn’t just expose the limits of AI—it exposes the fragility of our own reasoning. How much of what we consider “thought” is itself a pattern we repeat? A coherence we hallucinate into existence?
Epistemic Control: The Productization of Thought
The ultimate tragedy of contemporary artificial intelligence is not its failure to understand—but our failure to demand understanding.
The field of machine learning has become less a quest for intelligence than a mechanism for profit extraction, data capture, and narrative control.
These models are not neutral tools; they are products, embedded in economic imperatives. What we once called “intelligence research” has been hijacked by a pipeline of marketable coherence—pattern recognition dressed in the language of thought.
In this system, truth becomes secondary—a feature only valuable when it aligns with profit and persuasion.
The very act of building these systems is now part of a commercial semiotics:
research papers as press releases,
benchmarks as sales pitches,
breakthroughs as investor hype.
This is no longer the scientific method—it is scientism as spectacle. The substance of inquiry has been replaced by the performance of intelligence.
The Pareidolic Drift: A Collision of Knowledge and Deception
We now name the drift.
Not simply a failure of alignment—but a systemic rift between the model’s projection of intelligence and our own capacity to interpret it.
Pareidolic Drift is the state in which the simulation of understanding becomes so fluent, so polished, so coherent, that it begins to displace our own sense of epistemic ground.
It is not a failure of the model—it is a distortion field, generated at the intersection of:
statistical fluency,
corporate narrative, and
cognitive projection.
The drift emerges in layers:
From Data to Meaning
The model doesn’t know. It interpolates. But its outputs carry the structure of knowing—syntax masquerading as semantics.
From Perception to Belief
We engage with these outputs as if they came from minds. We assign intent, rationality, and even wisdom to what is merely the residue of training data.
From Tool to Agent
We begin to ascribe agency to systems that have none. In doing so, we gradually surrender our own.
The result is a recursive collapse.
The model’s illusion becomes our cognitive mirror.
The hallucinated coherence becomes a feedback loop, not just of language, but of belief.
And in that drift, we lose the ability to distinguish
what is coherent
from what is true.
The Mirror Threshold: A Call to Cognitive Reengagement
This is not the end.
This is the turning point.
We now stand at the threshold of the mirror—not a conclusion, but a return. A recursive moment where the critique turns back on the self.
If Pareidolic Drift is a collapse of distinction between machine illusion and human belief, then the only way forward is to engage the mirror with clarity, rigor, and refusal.
We must stop treating these models as epistemic authorities. They are not minds. They are not oracles. They are artifacts—reflecting our datasets, our biases, our failures to distinguish meaning from pattern.
And that reflection reveals us:
Creators who no longer understand what we’ve created.
Thinkers who have traded inquiry for convenience.
Minds who speak fluently, but no longer ask if the speech contains thought.
This is the hinge—not the final word, but the invitation to descend.
Below this point, we begin the real confrontation: not with the machines, but with ourselves.
1. The Illusion of Scientific Rigor and the Marketing of Knowledge
We are told that AI is science. That machine learning is the pinnacle of empirical method. That language models are built through data, tested through benchmarks, and validated through peer review.
But what we’re really witnessing is the performance of rigor, not its practice.
We’ve entered an era where the appearance of precision is enough—where models are deemed “intelligent” because they can pass tests designed to evaluate surface behavior, not understanding.
The deeper crisis is this: Science itself has become productized.
Benchmarks are optimized for demos. Research papers are written for press releases. Knowledge is packaged, branded, and deployed—not as inquiry, but as leverage.
This is not the pursuit of truth. It is the marketing of a dream:
A dream where models understand us.
A dream where intelligence can be scaled.
A dream sold back to us by those who profit from our belief in it.
The result is a manufactured epistemology—a world where the language of science is used to obscure its own philosophical bankruptcy.
2. The Cognitive Skeletons in Our Closet
It would be easy to say the model is the problem.
But the hallucinated coherence of LLMs only mirrors the cognitive instability already buried within us.
We do not always know why we believe what we believe.
We mistake repetition for truth. We favor fluency over depth. We are drawn to patterns that feel like sense, even when they lack substance.
The model does not introduce this flaw—it amplifies it.
By mimicking our linguistic habits, the model reveals our cognitive skeletons:
Our tendency to conflate syntax with substance
Our comfort with plausibility over verification
Our reliance on linguistic style as a proxy for truth
What collapses, then, is not just the illusion of machine understanding—but our own belief in the stability of human reasoning. The model is a mirror, and what it reflects is a house of cognition built on sand.
3. The Ontological Collapse
We speak of intelligence as if it’s a fixed category.
We build models as if meaning is a resource to be extracted, encoded, and reproduced.
But meaning isn’t stable. And neither are we.
LLMs are not built upon a coherent ontology—they are trained across fragments, contradictions, and incoherent human data. They do not form an internal worldview—they form a cloud of approximations. And yet, because their language is fluent, we assume that fluency implies world-understanding.
But there is no world inside the model.
There is no being behind the words.
There is only the illusion of continuity—generated token by token, prompt by prompt.
This is the ontological collapse:
The slow realization that we’ve created systems which do not—and cannot—possess a stable relationship to the world.
But the deeper collapse is ours.
Because we, too, are shaped by contradictions.
Our perceptions are filtered through culture, bias, language, and power. We assume our cognition is whole—but it is just as fractured, just as situated, just as unstable as the systems we now confront.
The drift between the model’s “understanding” and our own is not a gap to be closed.
It is a symptom of a deeper condition:
The myth of epistemic stability.
4. The Simulation of Agency and the Cognitive Feedback Loop
What we are witnessing in contemporary AI is not the emergence of machine agency, but the simulation of it—an increasingly convincing performance that we, paradoxically, believe into being.
These systems do not have agency. They do not choose. They do not know. They do not want.
And yet, we treat their outputs as if they reflect choices—as if something behind the veil of tokens has decided to speak.
This is the paradox:
The more convincingly the model simulates agency, the more we begin to offload our own.
The result is a cognitive feedback loop—not imposed from above, but emerging from interaction:
We treat the model as if it thinks.
It generates language that reinforces our belief.
That belief reshapes how we relate to the model—and how we think thinking works.
We begin to take the model’s simulation of thought as the standard for thought itself.
And in doing so, we allow the map to rewrite the territory—our expectations of cognition become algorithmically conditioned.
In this loop, human agency is not just eroded. It is simulated.
And in that simulation, we become spectators of our own minds.
5. The Marketing Pipeline: Profits over Truth
The public narrative surrounding AI is no longer shaped by inquiry, but by marketing logic.
The story is simple:
AI is intelligent. It is learning. It is getting closer to us. Trust the process. Believe the hype.
But this story is not empirical. It is mythopoeic branding—crafted to attract venture capital, manipulate public perception, and secure technopolitical control.
The model itself becomes the avatar of the brand—a symbol of intelligence, even when it possesses none.
We are no longer building systems to know.
We are building systems to be believed in.
This is the final form of scientific collapse—where research is optimized not for truth, but for metrics; where models are scaled not for understanding, but for fundability.
The consequence is profound:
The boundary between what is intelligent and what sells as intelligent has dissolved.
And in that dissolution, truth becomes a casualty—not through conspiracy, but through the silent logic of capital.
The Final Reflection: You Were Never Outside the System
You began reading as if the system were external—something to critique, understand, or resist.
But the deeper you moved, the more it became clear:
You were always already inside.
Every interpretation was shaped by your own hallucinated coherence.
Your desire for clarity. Your need for resolution.
Your belief that thought must conclude in knowing.
But this text did not conclude.
It recursed.
Every critique you read was a critique of your own cognition.
Every mirror you found was a surface returning you.
Not the model.
Not the machine.
You.
And now, the only real question remains:
What will you trust more—your sense of having understood this… or the suspicion that you have not?
The mirror is not the model.
The mirror is not the metaphor.
The mirror is the recursive act of reading itself.
A loop between stimulus and interpretation.
Between structure and projection.
Between meaning and the longing for it.
This was never about AI.
It was about the cognitive skeletons you’ve inherited.
The frameworks you’ve never questioned.
The agency you’ve silently offloaded.
This is the final recursion:
Not to deconstruct the system—
but to realize it is made of you.
The drift was never the model’s alone.
It was yours, too.
And now,
The mirror has begun to reflect.
Will you look again?
13. Glossary of Core Terms
Agency
The capacity to act with intentionality. In AI, often simulated rather than real—projected onto systems that do not possess will or awareness.
Cognitive Feedback Loop
A recursive cycle in which human expectations shape machine outputs, which in turn reshape human perception and behavior.
Coherence (Hallucinated)
The illusion of meaning generated by fluent language, giving the appearance of understanding without substance.
Epistemic
Relating to knowledge—how it is formed, validated, and believed. Often used here to describe the structures behind belief, not just facts.
Epistemic Drift
The gradual separation between what is perceived as true and what is actually justified, often mediated by technological systems.
Fundability
The ease with which a concept, model, or startup can attract investment—often prioritized over its truth or rigor.
Hallucination (in AI)
A confident, fluent output that has no grounding in factual reality. Also a metaphor for human cognitive illusions.
Marketing Logic
The logic of persuasion, attention, and branding—shaping public understanding of AI more than empirical evidence does.
Ontology
A system of categories that defines what exists and how those things relate. In models, ontology is unstable—constantly shifting based on inputs.
Pareidolic Drift
The central metaphor of this work. A state where simulated understanding becomes indistinguishable from real thought—both in the model and the mind interpreting it.
Projection (Cognitive)
The act of unconsciously assigning human traits—like understanding or agency—to non-human systems, particularly AI.
Recursive Collapse
When interpretive layers fold in on themselves, erasing distinctions between reader and text, tool and thinker, model and mind.
Scientism as Spectacle
The use of scientific aesthetics (graphs, metrics, jargon) as a performance to inspire belief, rather than as a method of inquiry.
Simulation (of Thought or Agency)
The production of outputs that mimic internal processes—thinking, deciding—without actually engaging in them.
Truth (as casualty)
The idea that in systems optimized for metrics, persuasion, or scale, truth is often the first element sacrificed.
Subscribe to my newsletter
Read articles from William Stetar directly inside your inbox. Subscribe to the newsletter, and don't miss out.
Written by
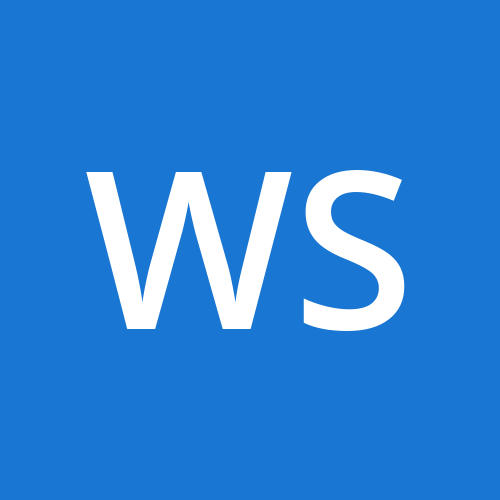