AI Jargon Made Simple: How to Begin with Generative AI
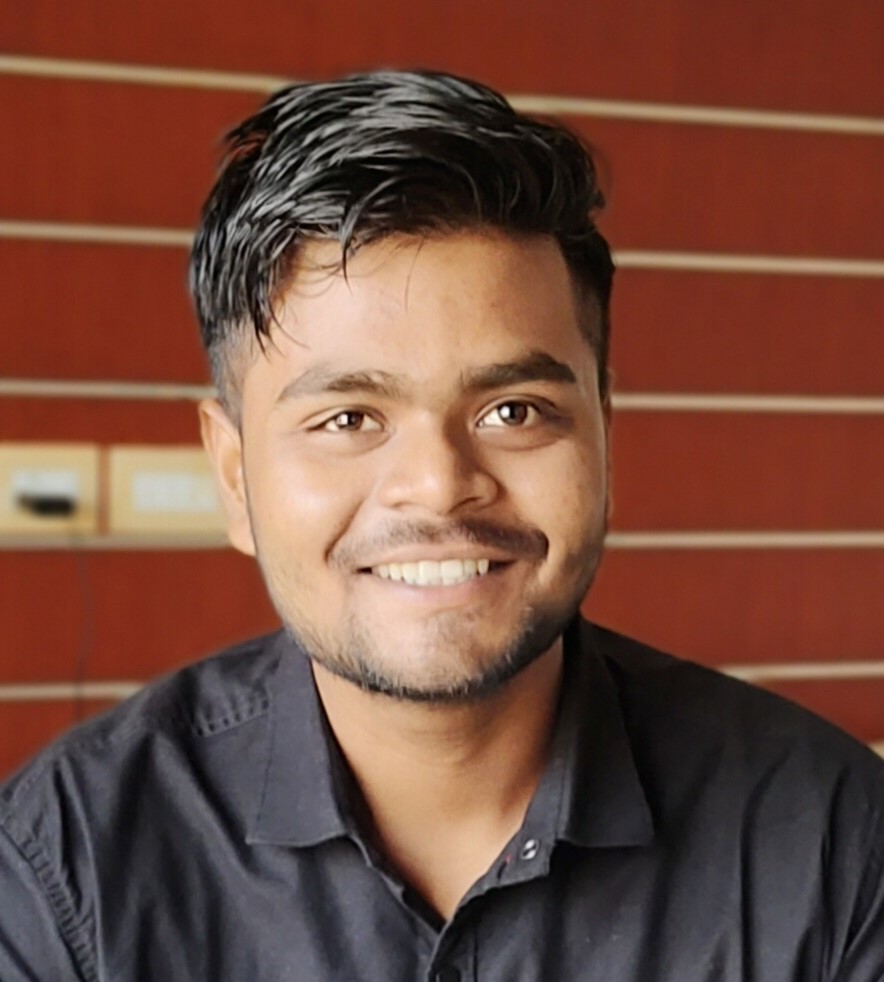
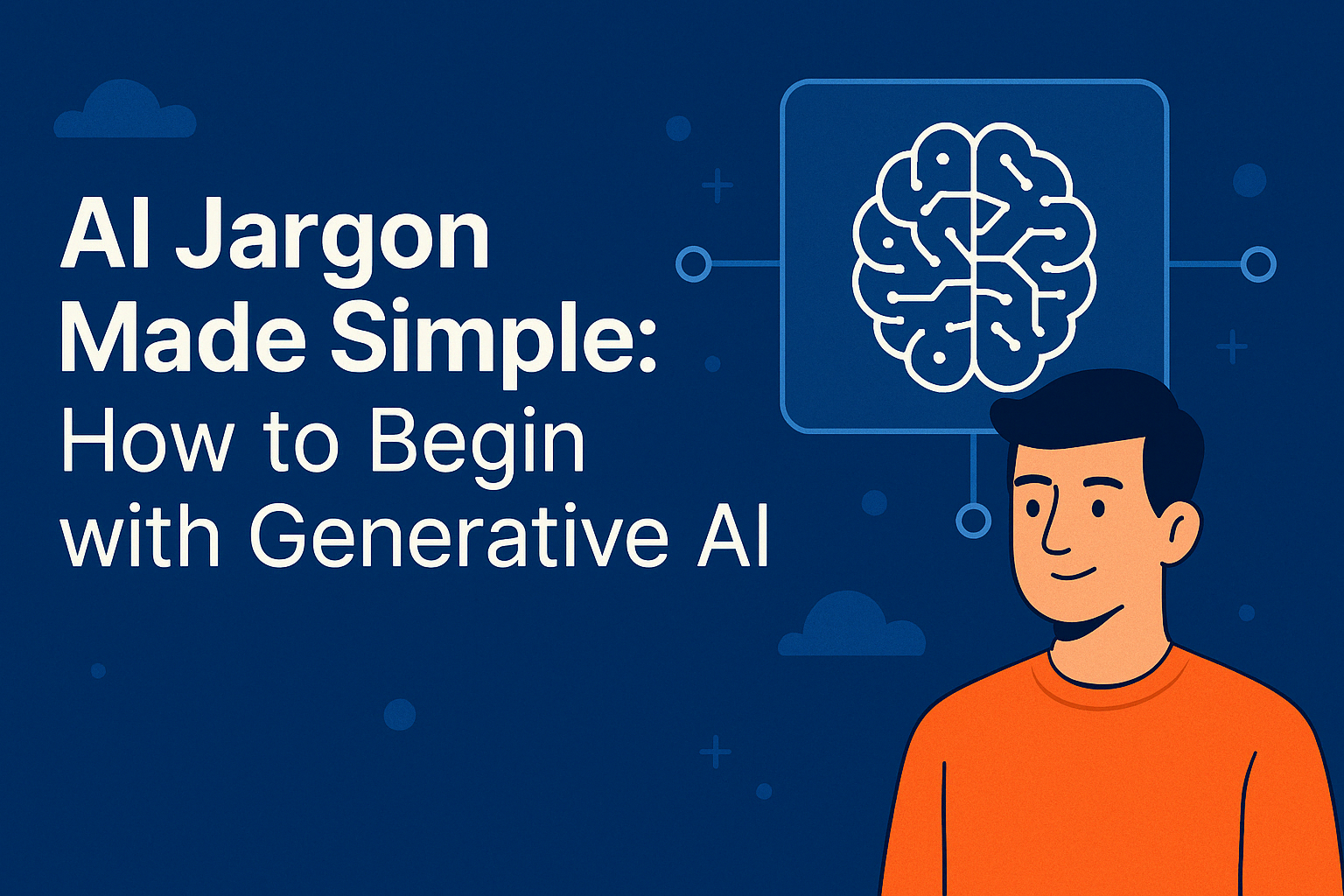
Back in 2023, I thought ChatGPT was some magical tool that could create anything I told it to. I had no idea how it worked. Now I know—it’s just a model that predicts the next most probable item based on input. Sounds simple? Let's break it all down.
1. Introduction
Have you ever thought how Chatgpt and other AI tools work. In background they use something called Transformer. (Not from the cybertron, but a neural network or we can say a Machine learning Model.)
In 2017, Google published a research paper titled "Attention is All You Need". This paper introduced the Transformer model.which become the base of Ai tools including chatGPT.
Now , What is GPT.
Gpt stands for Generative Pre-trained Transformer.
Generative: It means it can generate new text form the based on your input text. For example You give input as write a story and it will generate a new story from scratch by predicting the next.
Pre-trained: It means the model is trained on a very large data from the internet from different blogs,websites,books,repositories and any other data available on internet.Model must be pre-trained to answer your queries.And data that is used for training should be original (Human generated) not synthetic data (generated by some other ai model).Training help model to understand the grammar,text,relation between different things and context.
Transformer: Transformers are special deep learning models that process sequences of text by understanding context. They power models like GPT-4, BERT, and T5.
🧠 Example:
If you say:
"I went to the bank to deposit money."
The model understands that "bank" refers to a financial institution—not a riverbank. This is possible because Transformers use self-attention to understand context.
Transformers use self-attention to understand context.
🏗️ 2. Encoder
An encoder reads the input text and converts it into a format the model understands—usually a numerical vector (embedding).
🔍 Example:
Input: “I love ice cream.”
Encoder turns it into vectors like:
[0.23, 0.58, -0.12, ..., 0.91] for each token in the sentence.
🛠️ 3. Decoder
The decoder is responsible for generating text. It takes the encoded input (or previous words) and predicts the next word in the output.
📝 Example:
Prompt: “AI is going to”
Decoder might generate: “revolutionize”, then “the”, then “world.”
So output becomes:
“AI is going to revolutionize the world.”
📊 4. Embeddings (Vector Representation)
Embeddings turn words into high-dimensional numbers that capture meaning and relationships.
🎯 Example:
Words like:
“king” and “queen” → embeddings are close together.
“king” - “man” + “woman” ≈ “queen” (This is real vector math done in models!)
🧭 5. Positional Encoding
Transformers don’t process text word-by-word in order like RNNs. So we need a way to tell them the position of each word.
⏳ Example:
Sentence A: “Dog bites man.”
Sentence B: “Man bites dog.”
Same words, different meaning. Positional encoding helps the model distinguish this.
🧠 6. Semantic Meaning
Semantic meaning is the contextual meaning of a word.
💡 Example:
“I deposited money at the bank.”
“The picnic was near the river bank.”
Same word “bank,” different meanings. Transformers use attention mechanisms to detect these differences.
🧲 7. Self-Attention
Self-attention allows each word to look at every other word in the sentence to figure out meaning.
🔗 Example:
I told my friend I passed because I studied hard.
Self-attention helps the model understand that “I” is related to “passed” and that “studied” is the reason why.
🧠 8. Multi-Head Attention
This is like having multiple attention filters. Each head focuses on different things—syntax, grammar, long-range context.
🎥 Example:
In “The boy kicked the ball and it rolled away,”
One head might focus on “boy” → “kicked”
Another on “ball” → “rolled”
This diversity helps the model better understand complex sentences.
📈 9. Softmax
Softmax converts raw model scores (logits) into probabilities.
🎯 Example:
If possible words are:
“cat” (0.9)
“dog” (0.6)
“bat” (0.1)
Softmax chooses 'cat' because it has the highest score but if you adjust the temperature, it might consider lower-scoring words to make the output more creative.
🌡️ 10. Temperature
Temperature controls creativity. It affects how confident the model is when picking a word.
🔥 Example:
Temperature 0.2: Picks “cat” 99% of the time.
Temperature 1.0: Might sometimes pick “dog” or “bat” to make output more diverse.
Use higher temp in storytelling, lower for factual Q&A.
📅 11. Knowledge Cutoff
A model can only talk about things it was trained on. The knowledge cutoff is the last date of training data.
🧭 Example:
If the cutoff is April 2023, it won’t know about iPhone 16 unless you tell it.
✂️ 12. Tokenization
Tokenization is the process of breaking input text into smaller units called tokens, which are then converted into numbers so the model can understand them.
🔠 Example:
Let’s say you have the sentence:
“Transformers are awesome”
The model might tokenize it as:
["Transform", "ers", "are", "awesome"]
Then, each token is converted into a number using the model’s vocabulary:
["Transform" → 3021, "ers" → 7891, "are" → 67, "awesome" → 9823]
These numbers are then passed to the neural network for processing.
📚 13. Vocabulary Size
The model only knows a fixed number of tokens—this is called vocabulary size.
📘 Example:
If vocab size is 50,000, and the word “omniverse” is not in it, the model splits it into known parts like “omni” + “verse”.
🎯 Conclusion
What once felt like magic is actually a beautiful combination of math, data, and smart algorithms. Transformers and the models built on them.like ChatGPT aren’t just guessing. they’re using deep learning, attention mechanisms, and language understanding to make informed predictions one token at a time.
Now that you’ve explored the core concepts like tokenization, self-attention, embeddings, and more, you’ve taken your first step into the world of modern AI.
you now understand what’s happening under the hood and that’s pretty awesome.
Thanks for reading!
Subscribe to my newsletter
Read articles from Hitendra Singh directly inside your inbox. Subscribe to the newsletter, and don't miss out.
Written by
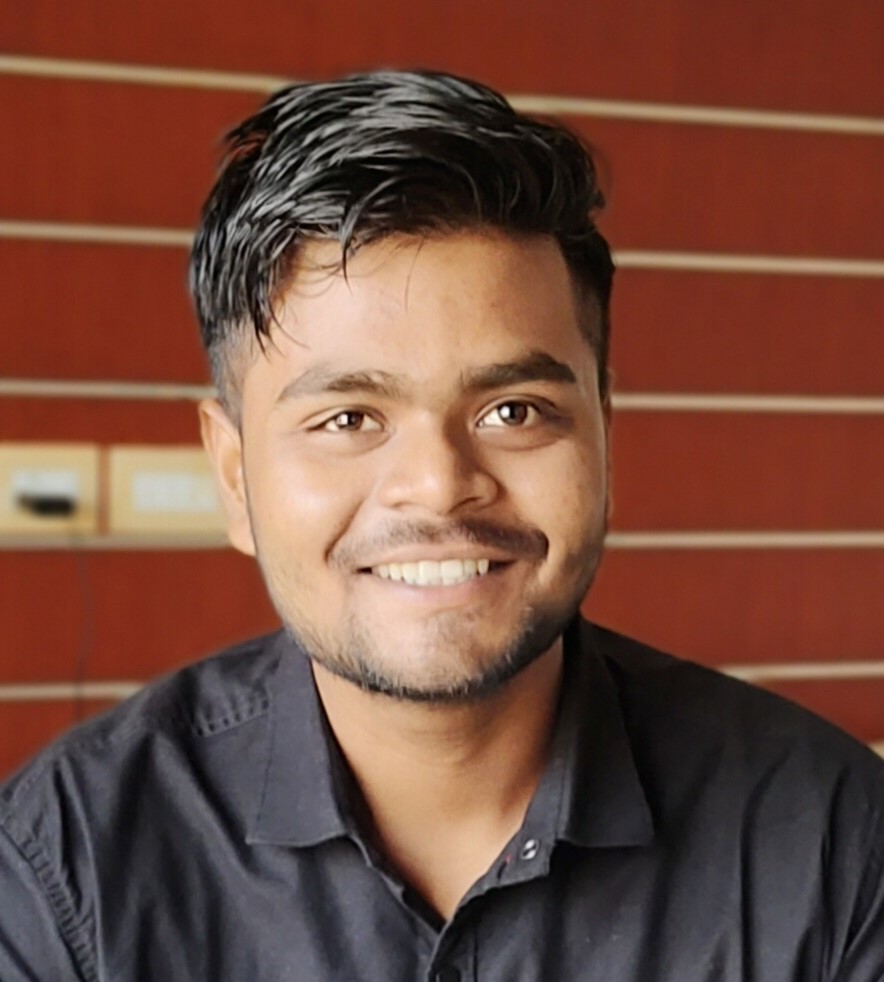
Hitendra Singh
Hitendra Singh
I am a Full Stack Developer