How AI Agents Are Transforming Retail Analytics
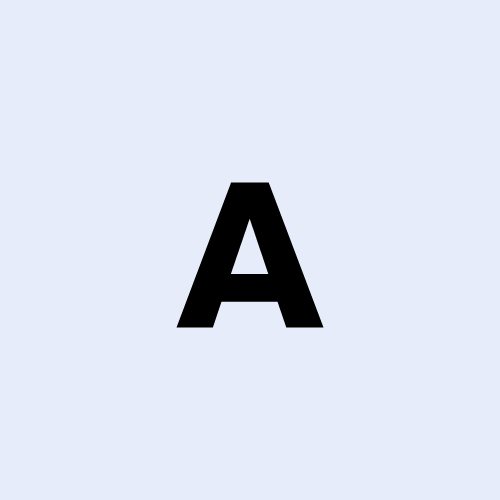
Introduction
Retail has always been a data-rich industry. From customer behavior and sales trends to inventory management and supply chain logistics, retailers have access to an abundance of information. However, turning that raw data into actionable insights has traditionally required manual analysis, large analytics teams, and time-consuming workflows. That’s changing rapidly with the rise of artificial intelligence particularly with the integration of AI agents into retail operations.
AI agents are autonomous programs powered by large language models and other AI technologies that can reason, interact, and execute tasks. They are ushering in a new era of data-driven decision-making in retail by automating analytics workflows, providing real-time insights, and enabling intelligent actions based on customer and business data. In this article, we explore how AI agents are transforming retail analytics, what makes them effective, and the key use cases that are already making a difference for modern retailers.
The Role of AI Agents in Retail
At the core, an AI agent in retail is designed to act with a clear purpose: analyze data and help stakeholders make better decisions. Unlike traditional scripts or business intelligence dashboards, AI agents are dynamic. They can interpret unstructured requests, generate SQL queries, extract insights from data, and explain their reasoning all without requiring the user to know how to write code or queries.
An AI agent typically operates in an agentic workflow, which is a modular and iterative process where the AI agent breaks down a task, decides which tools or data it needs, and refines its output based on feedback. This capability makes it especially powerful for retail businesses that need to react quickly to market trends and operational issues.
How AI Agents Work in Analytics Environments
AI agents can be integrated directly into data analytics platforms through APIs or embedded within notebooks and dashboards. When connected to a large language model, such as GPT or Gemini, they can take plain-language input from a user like "What were our best-selling items last quarter in the Northeast region?" and convert it into structured queries.
These agents are often connected to metadata sources, like dbt catalogs or data dictionaries, which help them understand the structure and semantics of data warehouses. This ensures the AI agent generates accurate queries and contextual insights, minimizing errors and misinterpretations.
AI agents development companies often build bespoke solutions that tailor the agent’s behavior to the client’s data stack and business goals. This might involve building custom tools, tuning prompts, and creating robust error-handling and validation layers to ensure trustworthy insights.
Key Use Cases of AI Agents in Retail Analytics
Let’s look at some of the most impactful ways AI agents are being used in retail:
1. Automated Sales Reporting
Instead of waiting for weekly sales reports, retail managers can now use an AI agent to request up-to-the-minute data summaries. The agent can instantly pull sales by region, store, or product category, compare them to previous periods, and highlight anomalies without needing a data analyst on call.
2. Customer Behavior Insights
Understanding customer journeys is critical in retail. AI agents can analyze purchase histories, session data, and demographics to uncover patterns like abandoned carts, frequent buyers, or high-lifetime-value segments. They can also answer ad-hoc questions from marketing or merchandising teams in real time.
3. Inventory Optimization
Stockouts and overstocking are costly mistakes. AI agents can identify trends in product demand, analyze supply chain delays, and recommend adjustments to inventory strategies. They can even simulate “what-if” scenarios based on seasonal trends, past promotions, or market conditions.
4. Promotion and Pricing Analysis
AI agents can help assess which promotions drove the most conversions or revenue by parsing data from past campaigns. They can also assist in dynamic pricing strategies by monitoring competitor pricing and market demand in real time.
5. Store-Level Performance Monitoring
Large retailers often operate dozens or hundreds of locations. AI agents can track store-level KPIs like foot traffic, conversion rates, and customer satisfaction scores. When a store underperforms, the agent can provide a detailed report explaining the drop and suggest corrective actions.
Benefits of AI Agents in Retail
Faster Decision-Making
Retail moves fast. AI agents accelerate decision-making by drastically reducing the time it takes to go from question to insight. Executives and managers no longer need to wait for a data team to pull a report—they can just ask the AI agent.
Democratization of Data
AI agents allow non-technical users to explore data on their own. This democratizes analytics across departments, empowering marketing, operations, finance, and sales teams to ask their own questions and act on insights.
Scalability and Consistency
AI agents can be scaled across multiple teams and geographies without hiring new analysts for each one. And since they operate on structured logic and consistent data sources, they produce reliable outputs across the board.
Cost Efficiency
By reducing reliance on manual reporting and speeding up data exploration, AI agents can reduce operational costs and free up data teams to focus on there higher-value tasks like model development and experimentation.
Challenges and Considerations
While the benefits are compelling, deploying AI agents in a retail setting isn’t without challenges:
- Data Quality: AI agents rely on clean, well-documented data. Poor data hygiene can lead to misleading results.
- Security and Compliance: Retailers must ensure that AI agents handle sensitive customer or financial data in accordance with data protection regulations.
- Prompt Engineering: Defining effective system prompts that guide the AI agent’s behavior is still a bit of an art and requires iteration.
- Model Limitations: AI agents built on the LLMs can occasionally hallucinate or misinterpret intent. Safeguards like rule-based validation or human-in-the-loop reviews are often necessary.
AI agents development companies work closely with retailers to address these issues, often building custom logic or integrating model fine-tuning to align outputs with business needs.
The Future of Retail Analytics with AI Agents
The role of AI agents in retail analytics is set to grow as models become more powerful, infrastructure more accessible, and demand for automation increases. In the near future, we can expect AI agents to be embedded into every major retail platform from CRM systems to ERP dashboards acting as intelligent copilots that help businesses adapt in real time.
We’ll likely see greater integration between AI agents and multimodal data as well, enabling them to analyze not only text and numbers but also images (like product displays), video (in-store traffic patterns), and even voice input (from customer service calls).
Retailers that embrace this agentic AI approach will be better positioned to innovate, respond quickly to changes, and stay competitive in a fast-evolving market.
Conclusion
AI agents are no longer a futuristic concept they’re already transforming how retailers understand and act on their data. By enabling more intuitive, dynamic, and autonomous analytics, they’re making it possible for teams across the organization to make smarter, faster decisions. As retail continues to evolve in complexity, AI agents will play an increasingly critical role in helping businesses stay agile, data-driven, and customer-focused.
From sales insights and customer analysis to inventory optimization and performance tracking, AI agents are proving to be indispensable tools for modern retail analytics. Investing in this technology today means building the foundation for a more intelligent, responsive retail business tomorrow.
Subscribe to my newsletter
Read articles from Albert directly inside your inbox. Subscribe to the newsletter, and don't miss out.
Written by
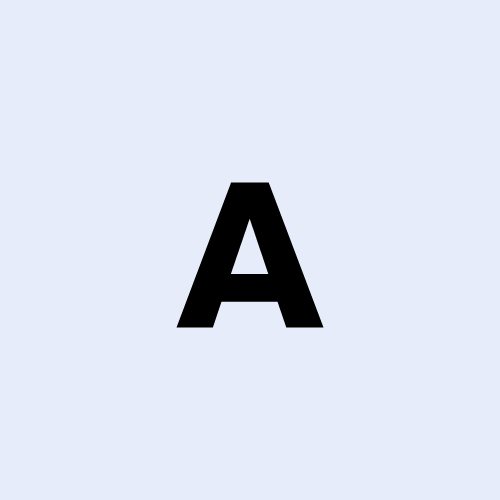