π Zoom In or Out: Query Decomposition for Smarter Retrieval
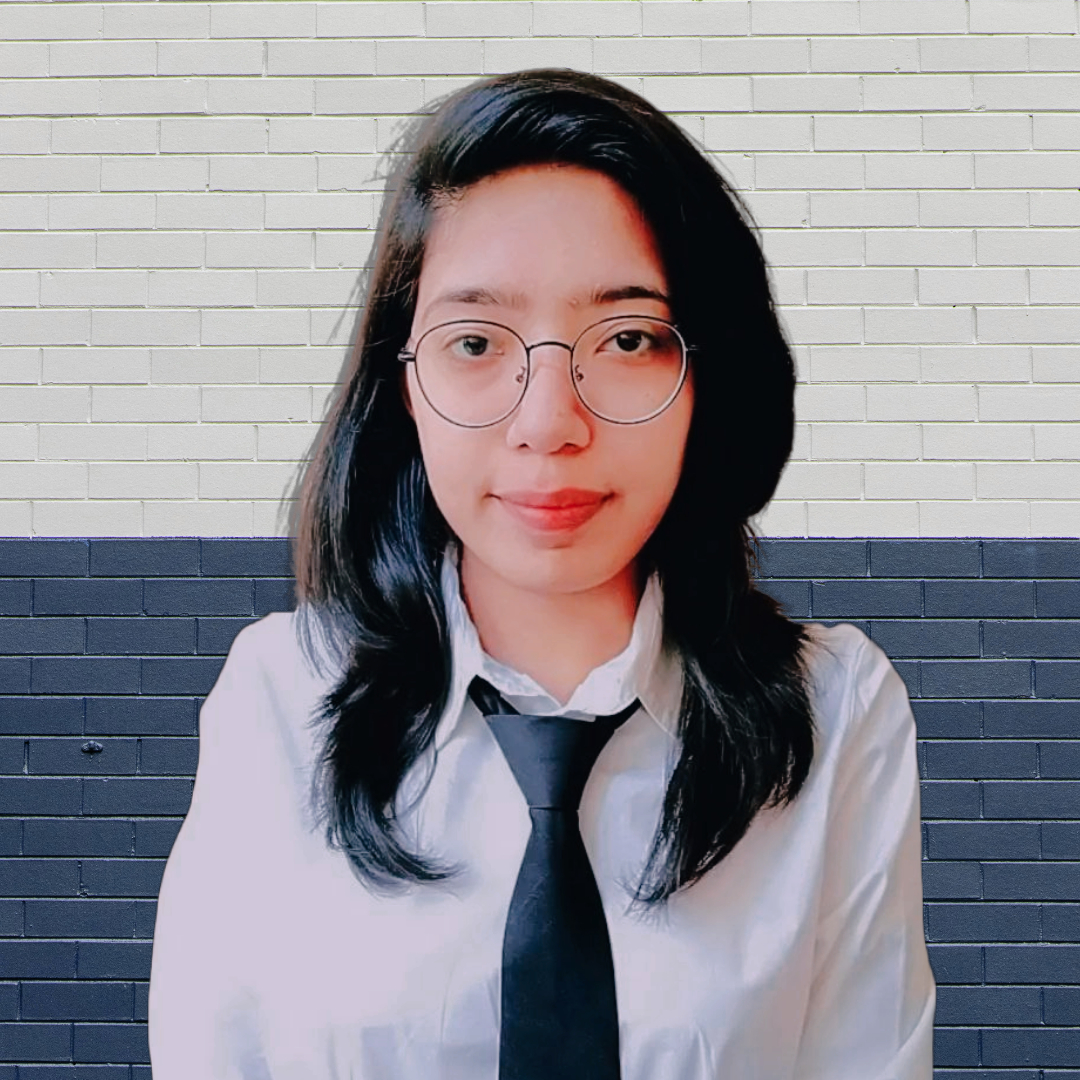

π§ What Is Query Decomposition?
Query Decomposition is a smart method that either breaks down complex queries into simpler parts (less abstraction) or expands narrow queries into broader topics (more abstraction). This helps AI systems retrieve the most relevant information β especially in multi-turn or research-heavy tasks.
π Two Modes of Decomposition
πΉ 1. Less Abstraction (Zooming In)
Breaks a complex or broad query into smaller, focused sub-questions.
Example:
User asks: βWhat is machine learning?β
It becomes:
What is a machine?
What is learning?
How do machines learn?
Helps fetch foundational context.
πΈ 2. More Abstraction (Zooming Out)
Expands a specific query into a more generalized one to capture broader context.
Example:
User asks: βWhere was Elon Musk born?β
Transformed into: βWhat is the life history of Elon Musk?β
Now, the model can retrieve a richer narrative, not just a fact.
π― Why Use Query Decomposition?
Handle vague, broad, or overloaded questions.
Improve grounding of answers.
Fetch facts and context.
Great for education and chatbot use cases.
π» Python Code: Query Decomposition with LangChain + Qdrant
from langchain.chat_models import ChatOpenAI
from langchain.prompts import PromptTemplate
from langchain.chains import LLMChain
from langchain.embeddings import OpenAIEmbeddings
from langchain.vectorstores import Qdrant
from qdrant_client import QdrantClient
from collections import defaultdict
# Initialize components
llm = ChatOpenAI()
qdrant_client = QdrantClient(url="http://localhost:6333")
embedding_model = OpenAIEmbeddings()
vector_store = Qdrant(client=qdrant_client, collection_name="my_collection", embeddings=embedding_model)
# === PROMPTS ===
# Prompt for LESS Abstraction (zoom in)
less_abstraction_prompt = PromptTemplate(
input_variables=["query"],
template="Break the following query into 3 simpler sub-questions to increase clarity: {query}"
)
# Prompt for MORE Abstraction (zoom out)
more_abstraction_prompt = PromptTemplate(
input_variables=["query"],
template="Reframe the following specific query into a broader context-based question or topic: {query}"
)
# === CHAINS ===
less_chain = LLMChain(llm=llm, prompt=less_abstraction_prompt)
more_chain = LLMChain(llm=llm, prompt=more_abstraction_prompt)
# === INPUT ===
user_query = "Where was Elon Musk born?"
# === Decompose Queries ===
less_sub_queries = less_chain.run(user_query).split('\n')
more_sub_query = more_chain.run(user_query) # One broader query
print("πΉ LESS Abstraction Queries (Zoomed In):")
for q in less_sub_queries:
print(f"- {q}")
print("\nπΈ MORE Abstraction Query (Zoomed Out):")
print(f"- {more_sub_query}")
# === RETRIEVE CHUNKS ===
all_queries = less_sub_queries + [more_sub_query]
retrieved_chunks = []
for query in all_queries:
docs = vector_store.similarity_search(query, k=5)
retrieved_chunks.extend(docs)
# === DEDUPLICATE ===
unique_chunks = list({doc.page_content: doc for doc in retrieved_chunks}.values())
# === DISPLAY RESULTS ===
print("\nπ Retrieved Chunks from Combined Decomposition:")
for doc in unique_chunks:
print(doc.page_content)
π Pros and Cons of Query Decomposition
Pros π | Cons π |
Helps resolve vague or overloaded queries | LLM might over-decompose or misinterpret intent |
Great for educational or research-style questions | Slight increase in processing time |
Captures both high-level and low-level information | Needs deduplication logic |
Works well in chain-of-thought reasoning models | May return overly generic chunks if not carefully prompted |
β Best Practices
Use prompt tuning to control whether you want more or less abstraction.
Limit to 3β4 sub-queries to avoid excessive noise.
Combine with RRF if merging chunks from multiple decompositions.
π Decomposition vs. Fan-Out: What's the Difference?
Feature | Fan-Out | Decomposition |
Purpose | Similar phrasing of same query | Simplifying or expanding the query |
Focus | Semantic variety | Conceptual breakdown |
Ideal For | Broad coverage | Depth and clarity |
Use them together for maximum retrieval power.
π Next Up: Blog 5 β No Data? No Problem. Meet HYDE β The AI That Writes Before It Retrieves
Thank you for reading our article! We appreciate your support and encourage you to follow us for more engaging content. Stay tuned for exciting updates and valuable insights in the future. Don't miss out on our upcoming articlesβstay connected and be part of our community!
YouTube : youtube.com/@mycodingjourney2245
LinkedIn : linkedin.com/in/nidhi-jagga-149b24278
GitHub : github.com/nidhijagga
HashNode : https://mycodingjourney.hashnode.dev/
A big shoutout to Piyush Garg Hitesh Choudhary for kickstarting the GenAI Cohort and breaking down the world of Generative AI in such a simple, relatable, and impactful way! π
Your efforts are truly appreciated β learning GenAI has never felt this fun and accessible. π
#ChaiCode #ChaiAndCode #GenAI #ChaiAndCode #GenAI #QueryDecomposition #ChainOfThought #LLMDesign #ChaiCode #ChaiAndCode #GenAI #PromptDesign #LangChain
Subscribe to my newsletter
Read articles from Nidhi Jagga directly inside your inbox. Subscribe to the newsletter, and don't miss out.
Written by
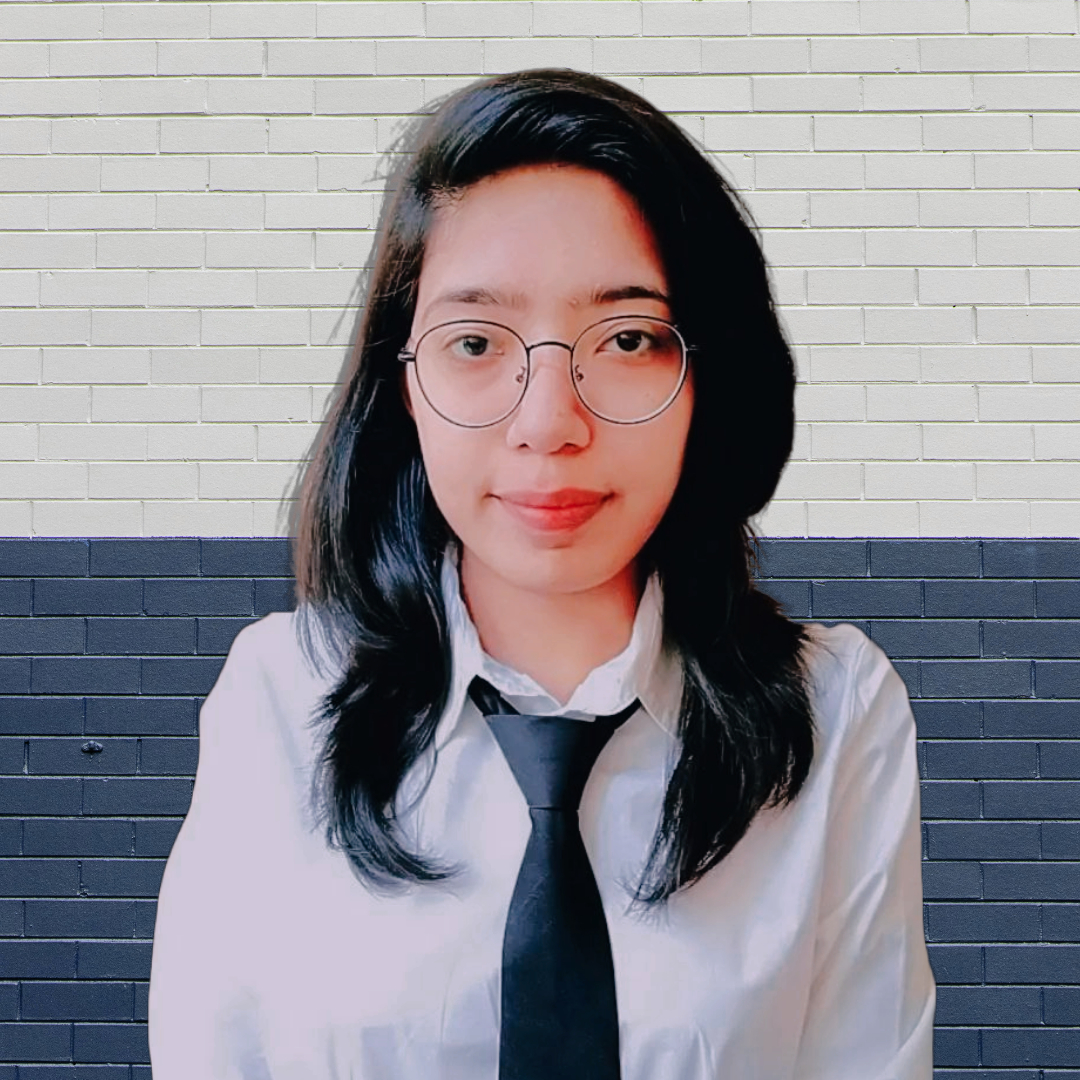