🔀 One Question, Many Answers: Unlocking AI Accuracy with Fan-Out Retrieval
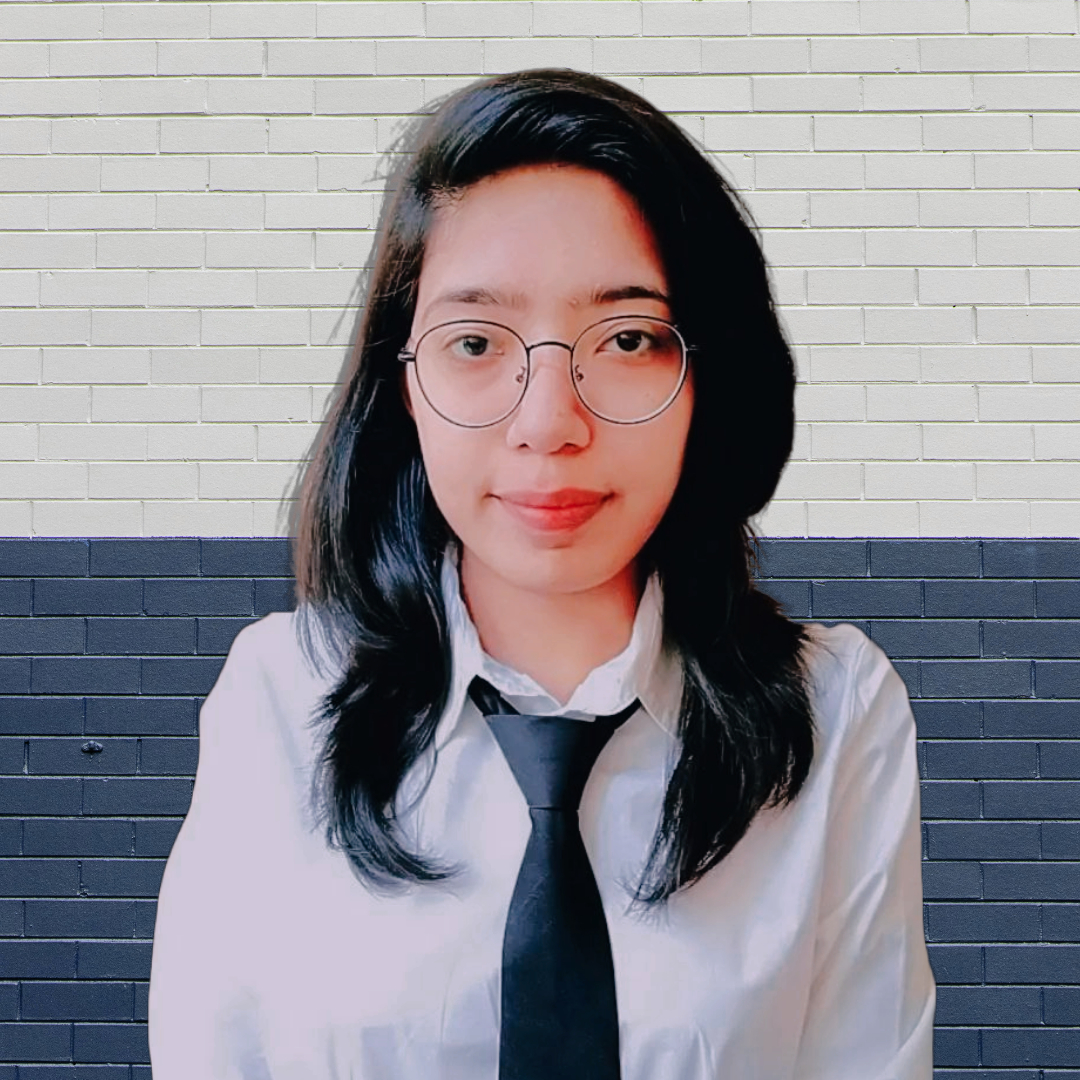

đź§ What is Fan-Out Retrieval?
Fan-Out Retrieval (also called Parallel Query Expansion) is a query transformation technique that takes a user's single query and creates multiple semantically similar versions of it. This helps ensure we retrieve diverse and relevant chunks from the knowledge base — especially useful in RAG (Retrieval-Augmented Generation) systems.
🎯 Why Use Fan-Out?
Let’s say a user asks:
“What is machine learning?”
That question is broad, and a single search might miss key chunks. But if we also search using:
“How does machine learning work?”
“Basics of machine learning”
“Introduction to ML”
…we can pull in more context, reduce ambiguity, and improve the generated answer.
đź§Ş How It Works: The Fan-Out Process
User submits a query (e.g., “What is machine learning?”).
LLM creates 2-3 semantically similar versions of the query.
Each version is used to search the vector database (like Qdrant).
Retrieved chunks are merged and deduplicated.
Top results are fed into the generation model as context.
đź’» Python Code Example: Fan-Out with LangChain + Qdrant
from langchain.chat_models import ChatOpenAI
from langchain.prompts import PromptTemplate
from langchain.chains import LLMChain
from langchain.embeddings import OpenAIEmbeddings
from langchain.vectorstores import Qdrant
from qdrant_client import QdrantClient
from langchain.docstore.document import Document
# Step 1: Initialize services
qdrant_client = QdrantClient(url="http://localhost:6333")
embedding_model = OpenAIEmbeddings()
vector_store = Qdrant(client=qdrant_client, collection_name="my_collection", embeddings=embedding_model)
llm = ChatOpenAI()
# Step 2: Prompt for generating similar queries
prompt_template = PromptTemplate(
input_variables=["query"],
template="Generate 3 semantically similar queries to: {query}"
)
llm_chain = LLMChain(llm=llm, prompt=prompt_template)
# Step 3: Input from user
user_query = "What is machine learning?"
generated_queries = llm_chain.run(user_query).split('\n')
# Step 4: Retrieve documents using all similar queries
retrieved_docs = []
for query in generated_queries:
docs = vector_store.similarity_search(query, k=5)
retrieved_docs.extend(docs)
# Step 5: Deduplicate results
unique_docs = list({doc.page_content: doc for doc in retrieved_docs}.values())
# Display the results
print("đź“„ Retrieved Unique Chunks:")
for doc in unique_docs:
print(doc.page_content)
✅ Pros and ❌ Cons of Fan-Out Retrieval
Aspect | Pros 👍 | Cons 👎 |
Recall | Increases the chances of retrieving all relevant information | May retrieve too much irrelevant data (noise) |
Context Depth | Provides richer and more varied context for the model | Might lead to overlapping or duplicated results |
Vague Queries | Excellent for handling short or ambiguous user inputs | Quality depends on the model’s ability to generate useful query variations |
Flexibility | Adapts well across different domains and query types | Needs good prompt design for generating variations |
Implementation | Simple to integrate with LangChain + Qdrant | Slightly increases latency due to multiple retrieval calls |
Response Accuracy | Enhances grounding for better generated answers | Poorly chosen query variants can dilute relevance |
Combination Potential | Works well with other techniques like RRF or HYDE | Requires extra filtering and merging logic |
âś… Best Practices
Limit fan-out to 2-3 variations max to avoid noise.
Use embedding-based deduplication to remove near-duplicates.
Combine with Reciprocal Rank Fusion (RRF) for even better relevance (covered in Blog 3).
🔜 Next Up: Blog 3 – How AI Ranks Better with RRF: The Genius of Merging Search Results
Thank you for reading our article! We appreciate your support and encourage you to follow us for more engaging content. Stay tuned for exciting updates and valuable insights in the future. Don't miss out on our upcoming articles—stay connected and be part of our community!
YouTube : youtube.com/@mycodingjourney2245
LinkedIn : linkedin.com/in/nidhi-jagga-149b24278
GitHub : github.com/nidhijagga
HashNode : https://mycodingjourney.hashnode.dev/
A big shoutout to Piyush Garg Hitesh Choudhary for kickstarting the GenAI Cohort and breaking down the world of Generative AI in such a simple, relatable, and impactful way! 🚀
Your efforts are truly appreciated — learning GenAI has never felt this fun and accessible. 🙌
#ChaiCode #ChaiAndCode #GenAI #ChaiAndCode #GenAI #FanOutRetrieval #SemanticSearch #LangChain #QdrantDB #GenAI #ChaiCode #ChaiAndCode #LLMEngineering #VectorSearch
Subscribe to my newsletter
Read articles from Nidhi Jagga directly inside your inbox. Subscribe to the newsletter, and don't miss out.
Written by
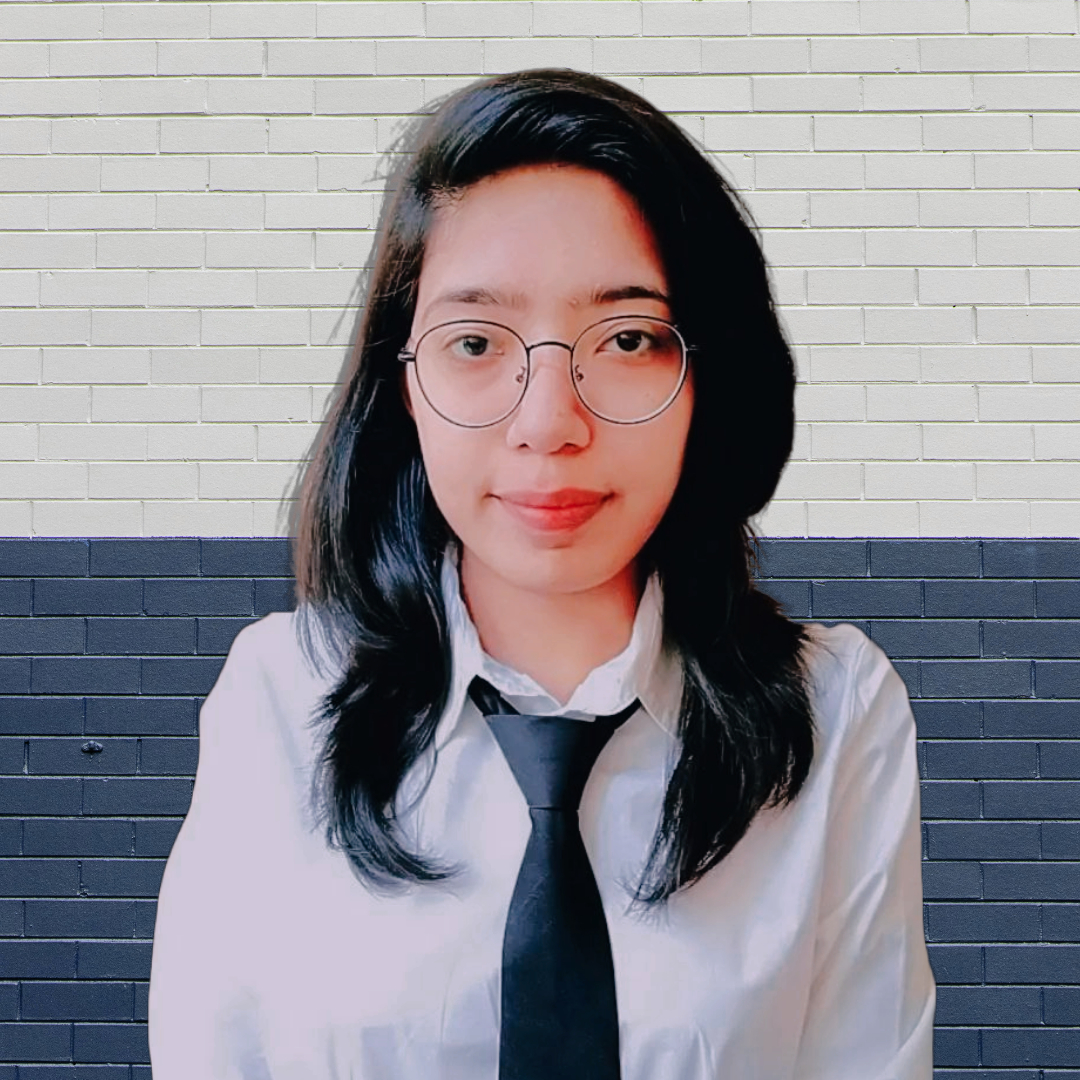