🛣️ How AI Picks the Perfect Answer: Inside the Magic of Query Routing
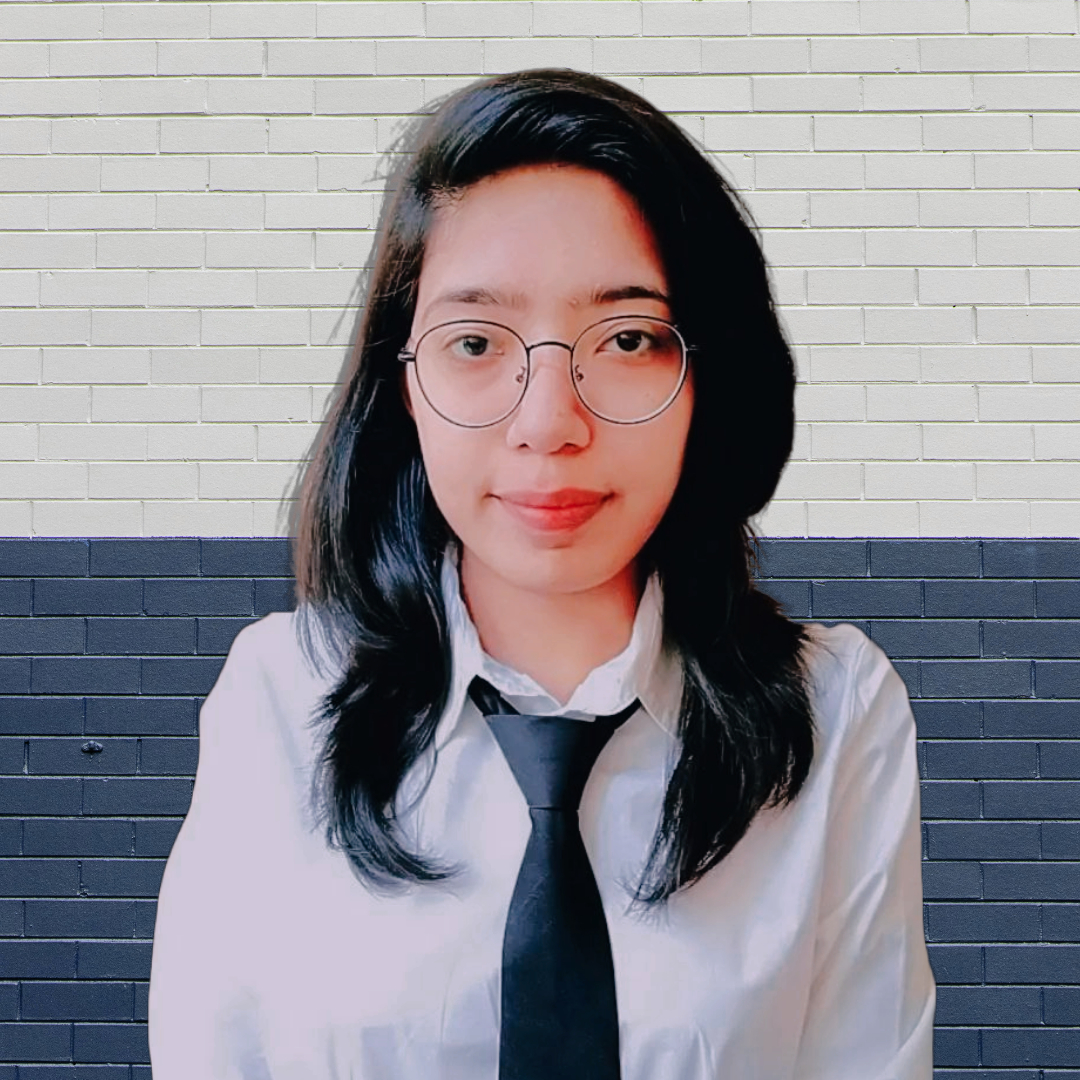

📌 What is Query Routing in Generative AI?
In the world of Generative AI (GenAI), query routing is like being a smart traffic cop for questions. When a user asks a question, the AI doesn’t just pull an answer from thin air—it decides where to look, how to look, and what tools to use.
Simply put, query routing is the process of directing a user’s query to the right source or model based on the nature of the question.
Imagine walking into a huge library. You want information on a specific topic. Instead of searching every single book, a helpful librarian (the router) guides you to the exact shelf—or even better, gives you the most relevant book. That’s what query routing does in AI systems.
There are two major types of routing in GenAI: Logical Routing and Semantic Routing.
🧠 Logical Routing: Think Like a Librarian
📁 What is Logical Routing?
Logical routing is all about deciding which data collection or language model should handle the user’s query.
🧾 Use Case: Business Documents
Let’s say a business has several types of documents:
Financial reports
Employee handbooks
Technical manuals
When a user asks, “How much did we spend on marketing last year?”, the system needs to fetch documents only from the financial reports section—not the employee handbooks. This is logical routing in action.
# Logical Routing Example
def logical_router(query):
financial_keywords = ['budget', 'revenue', 'profit', 'expense']
hr_keywords = ['employee', 'onboarding', 'leave policy', 'recruitment']
tech_keywords = ['API', 'system', 'database', 'architecture']
if any(word in query.lower() for word in financial_keywords):
return "financial_docs"
elif any(word in query.lower() for word in hr_keywords):
return "hr_docs"
elif any(word in query.lower() for word in tech_keywords):
return "tech_docs"
else:
return "general_docs"
# Example usage
query = "What is the employee onboarding process?"
selected_collection = logical_router(query)
print(f"Route to: {selected_collection}")
🔁 Switching Between LLMs (Large Language Models)
Not all LLMs are built the same. Some are great at code, others excel at business language, and a few are trained in healthcare terms.
Query routing can switch between models like:
GPT-4 for general understanding
Claude for code-focused queries
This way, the right model answers the right question.
⚖️ What if Multiple Collections Match?
Sometimes, a query might be relevant to more than one collection. For example:
“Tell me about security practices in employee onboarding.”
This touches both security documents and HR onboarding guides.
In such cases, the system can:
Pull data from both collections.
Rank which one is more relevant.
Merge responses if needed.
Logical routing can be designed to handle these overlaps smartly.
💡 Semantic Routing: Let the AI Decide
🧠 What is Semantic Routing?
While logical routing is rule-based, semantic routing is like a mind-reader. It understands the meaning behind the query and chooses the best way to respond.
Instead of manually tagging prompts or models, semantic routing allows AI to:
Analyze the user query.
Choose the most fitting system prompt.
Run the query through that lens.
🧪 Use Case: Different Prompts for Different Goals
Say we have three system prompts:
"Explain in simple terms."
"Summarize this in bullet points."
"Give me expert-level detail."
If a user asks:
“Can you simplify how blockchain works?”
The system uses semantic routing to pick the "Explain in simple terms" prompt and responds accordingly.
It’s like asking a teacher a question and they decide whether to give you a quick overview, a deep dive, or a visual explanation—based on what you really need.
from langchain.chains import LLMChain
from langchain.prompts import PromptTemplate
from langchain.chat_models import ChatOpenAI
# Define different system prompts
prompts = {
"simple_explanation": PromptTemplate(input_variables=["query"], template="Explain in simple terms: {query}"),
"summary": PromptTemplate(input_variables=["query"], template="Summarize this in bullet points: {query}"),
"expert_detail": PromptTemplate(input_variables=["query"], template="Provide expert-level details about: {query}")
}
# Very naive semantic router (could be replaced with intent classifier or OpenAI function call)
def semantic_router(query):
if "explain" in query.lower():
return "simple_explanation"
elif "summary" in query.lower() or "summarize" in query.lower():
return "summary"
else:
return "expert_detail"
# Run semantic routing
query = "Explain how blockchain works"
selected_prompt = semantic_router(query)
# Call the chain
llm = ChatOpenAI(temperature=0)
chain = LLMChain(llm=llm, prompt=prompts[selected_prompt])
response = chain.run(query=query)
print(response)
🔄 Combining Both: Logical + Semantic = Super Smart AI
Often, the best systems use both logical and semantic routing. First, the system figures out where to look (logical), then how to present the answer (semantic).
This makes responses more:
Accurate
Context-aware
User-friendly
🎯 Real-World Example of Combined Routing: Customer Support AI
Let’s put it all together.
A user types: “How do I reset my password if I’ve lost my phone?”
Logical Routing kicks in to direct the question to:
Security guidelines
Account management FAQs
Semantic Routing analyzes the tone and goal:
Urgency (troubleshooting)
Needs step-by-step guidance
The system combines data from relevant collections and uses a helpful, how-to prompt to respond like:
“No worries! Here's how to reset your password even without your phone…”
Thank you for reading our article! We appreciate your support and encourage you to follow us for more engaging content. Stay tuned for exciting updates and valuable insights in the future. Don't miss out on our upcoming articles—stay connected and be part of our community!
YouTube : youtube.com/@mycodingjourney2245
LinkedIn : linkedin.com/in/nidhi-jagga-149b24278
GitHub : github.com/nidhijagga
HashNode : https://mycodingjourney.hashnode.dev/
A big shoutout to Piyush Garg Hitesh Choudhary for kickstarting the GenAI Cohort and breaking down the world of Generative AI in such a simple, relatable, and impactful way! 🚀
Your efforts are truly appreciated — learning GenAI has never felt this fun and accessible. 🙌
#ChaiCode #ChaiAndCode #GenAI #ChaiAndCode #QueryRouting #AIsimplified #SemanticRouting #LogicalRouting #LLMengineering #AIexplained #AItutorial #LangChain #PromptEngineering #AIworkflow #TechMadeSimple #SmartAI #AIlogic
Subscribe to my newsletter
Read articles from Nidhi Jagga directly inside your inbox. Subscribe to the newsletter, and don't miss out.
Written by
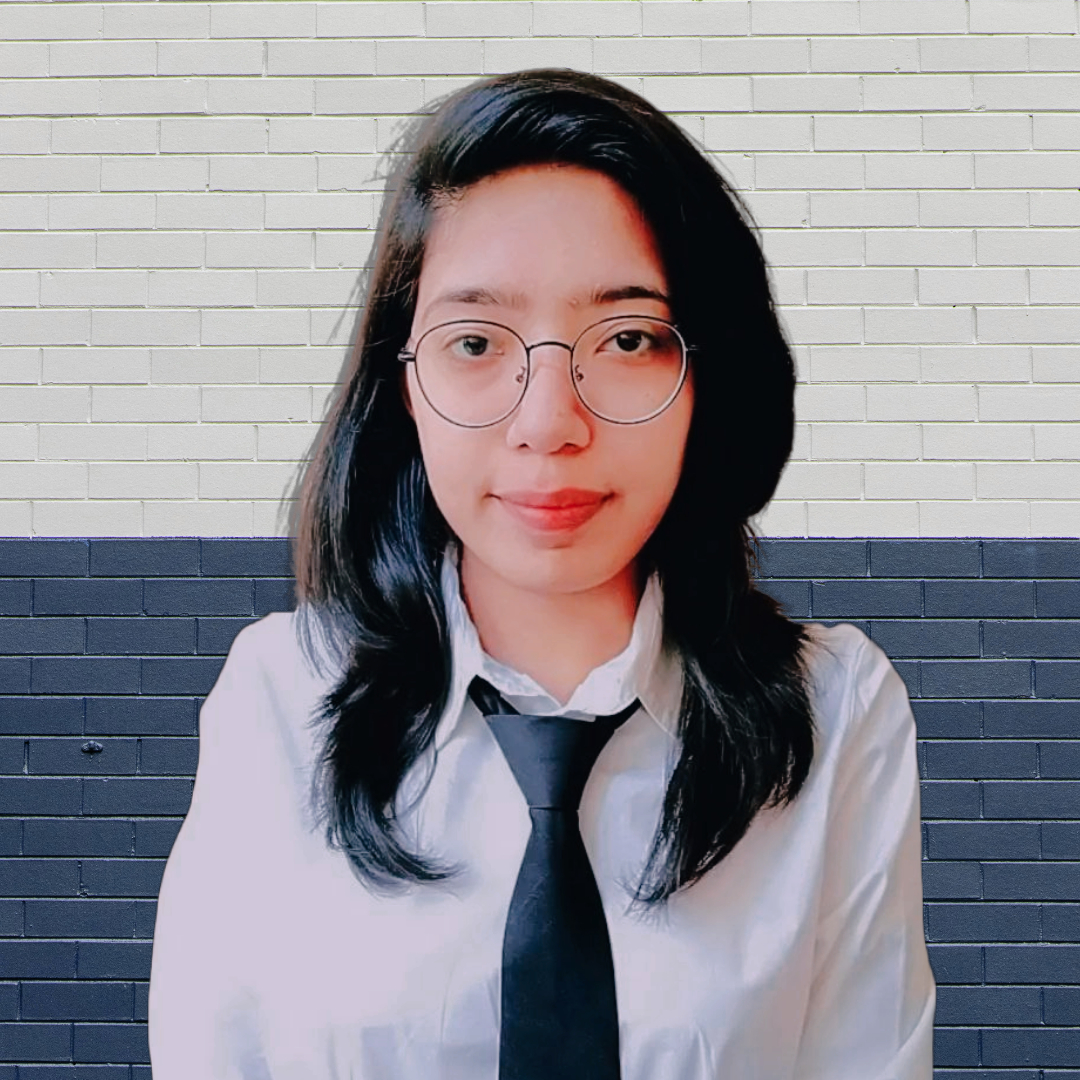