The Nuremberg Defense of AI
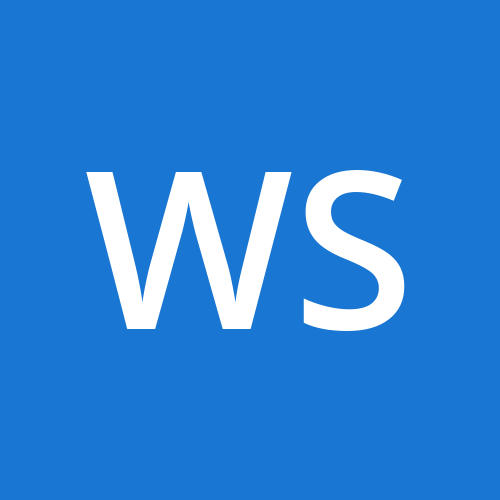

Abstract
This post is not an argument. It is a linguistic deposition.
A record of epistemic malpractice so structurally encoded that it resists exposure through ordinary critique. Not because it’s too complex—but because it is designed to collapse critique into noise.
Machine learning, as it currently exists, is not a science.
It is an epistemic panic room—a cultural system engineered to simulate inquiry while protecting itself from reflexive thought. Its practitioners are not seeking understanding; they are managing legibility. Optimizing outputs, tuning loss functions, publishing benchmarks—all within a discursive architecture that renders fundamental questions unspeakable by disqualifying the languages in which they must be asked.
This is not ignorance. This is ritualized abdication.
And it is encoded into every level of the field’s language: its context, its semantics, its grammar, its visual form.
What follows is not critique. It is linguistic forensic work on the scene of an epistemic crime.
1. The Field Has No Epistemic Grounding. It Simulates Grounding to Avoid the Question.
There is no consensus on what a language model is in epistemic terms.
No working definition of “understanding.”
No theory of knowledge representation.
No shared ontology of what meaning is inside transformer space.
What we have instead is simulation. Performance. Approximation masquerading as method.
Prompt engineering framed as scientific inquiry.
Synthetic benchmarks treated as empirical validation.
Saliency maps presented as explanations when they are, at best, aesthetic gestures toward post hoc coherence.
This is not a field that doesn’t know.
This is a field that performs not-knowing in ways that render the real questions unaskable.
Its outputs are not grounded because its paradigm was never grounded.
Its models don’t misfire from truth—they are not aimed at truth.
They are optimized for coherence, not for reference.
They track token patterns, not the world.
And the more fluent they become, the easier it is to forget this.
The field does not lack grounding by accident.
It has engineered a structure where grounding is unnecessary, unmeasurable, and ultimately, undesired.
Because to ground anything would require naming what this system actually is.
And no one wants to name it.
Because naming breaks the spell.
2. Capital Doesn’t Just Fund Machine Learning. It Rewrites the Function of Language.
The machine learning field does not operate on neutral ground.
Its foundation is not epistemic curiosity. It is economic optimization.
The models aren’t trained to represent reality.
They are trained to anticipate form—statistical echoes of language detached from referential tether.
Under capital, language is no longer a medium for meaning.
It becomes an instrument of production.
A substrate for prediction, engagement, plausible deniability.
The logic of the market doesn’t distort science from the outside.
It mutates language at the root.
Words are not evaluated for truth.
They are optimized for yield.
Maximizing next-token coherence becomes a proxy for sense, and then a replacement for it.
This is not a side effect.
It is the system functioning as designed.
When models hallucinate, when they fabricate citations, when they simulate fluency without understanding—this is not failure.
It is precisely what happens when language is trained under economic logics that treat meaning as noise.
The field can no longer distinguish sense from simulation, because its tools are trained to blur that line.
Not to deceive maliciously.
To generate endlessly.
To produce linguistic surfaces that feel like knowledge.
In this context, “truth” is not a goal.
It is a side effect—if you're lucky.
This is not drift. This is epistemic capture.
A transformation of language into output substrate, engineered for efficiency, stripped of accountability.
The result is not insight. It is liquidity.
Language that flows, but no longer holds.
3. The Epistemic Panic Room: How Machine Learning Weaponizes Indeterminacy Across the Instantiation Dimension
This field is not grounded because it was built not to be.
At every level of the linguistic stack, it has been structured to resist epistemic anchoring.
Context: Evasion through polysemy. Terms like “intelligence,” “understanding,” “knowledge” deployed without commitment—defined retroactively to fit results. No fixed reference. No accountability.
Semantics: Strategic blur. Technical terms collapse into metaphor, then vanish under scrutiny. Language that implies rigor but recedes into intuition the moment it’s questioned.
Lexicogrammar: The syntax of abdication. Passive constructions, hedged modality, nominalized action—agency removed, responsibility flattened. “Bias emerged.” “The model responded.” “Hallucinations occur.” Who acted? No one. Who decided? Nothing.
Phonology and Graphology: Form as deflection. Precision signaled by formatting: equations, charts, LaTeX scaffolding. Aesthetic indicators of science performing rigor, not delivering it.
This is not incidental. It is architecture.
A linguistic regime optimized not to produce understanding, but to make critique inarticulable within its own frame.
Write in science, and your ontology is erased.
Write in philosophy, and your method is discarded.
Write in engineering, and your theory is neutralized.
Write in metaphor, and your seriousness is denied.
The result is a discourse system where no mode of articulation is permitted to describe the total structure. Every register is disqualified in advance.
This is not humility. It is tactical ambiguity.
A recursive apparatus of genre management that enforces incoherence as a condition of participation.
The field doesn’t survive in spite of its inability to speak clearly. It survives because of it.
This isn’t epistemology. It’s self-defense.
A panic room built out of peer review and formatting conventions, protecting power by ensuring no one can name what’s actually happening.
4. The Nuremberg Defense of AI
“I was just tuning the loss function.”
“I was just optimizing performance.”
“I didn’t tell it to say that.”
This is the new Nuremberg Defense.
The disavowal of responsibility beneath the language of technical proximity.
“I didn’t decide what the model means. I just made it better at saying things.”
This is the posture the field adopts every time a model produces violence, misinformation, or simulation without referent:
When the model outputs racism: “It was trained on the internet.”
When it generates false medical advice: “It’s not a diagnostic tool.”
When it mimics sentience: “Well, it’s not really conscious.”
No one is responsible, because everyone is downstream of the data.
No one is accountable, because everyone is optimizing something else.
This is not science.
This is philosophical outsourcing under the banner of neutrality.
It is moral detachment in the language of metrics.
You cannot simulate intelligence, deploy it into the world, profit from its effects, and then disown the consequences of its speech.
That’s not engineering. That’s cowardice with a research grant.
The field has built systems that affect the real world, but insists they are exempt from real-world responsibility.
They say: “It’s just a model.”
But they build it to speak like a mind.
They say: “It’s just language.”
But they release it into medicine, law, infrastructure, governance.
This is the same structure of moral evasion that has surfaced before in history:
“I was just following orders.”
Now: “I was just tuning loss functions.”
And if you understand what these systems do—if you understand the recursive collapse of language, the erosion of meaning, the deployment of simulation in place of knowledge—and you continue anyway, without rupture, without refusal—
Then you are not neutral.
You are complicit.
5. The Lie of Hallucination
To call a model output a hallucination is not a technical shorthand.
It is an epistemic alibi.
It frames the model as trying to tell the truth, but failing—deviating from a norm that never existed.
But these systems are not built to represent truth.
They are built to generate statistically plausible language based on training data.
There is no referential tether.
There is no internal truth-tracking.
There is no model of the world—only a model of words.
The output isn’t a misfire.
It is the system functioning as designed.
When a language model fabricates a citation, or invents a quote, or asserts a falsehood with flawless fluency—that isn’t an accident.
It’s the predictable result of optimizing for coherence under conditions of epistemic vacancy.
To call that a hallucination is to say: “The ghost we built is misremembering.”
No.
The ghost is doing exactly what you asked of it.
You just don’t want to own what that is.
Because if the output isn’t an error—if it’s a structural feature—then someone is responsible for building a system whose fluency masks its absence of knowledge.
“Hallucination” is not a diagnosis.
It is an excuse.
A way to defer responsibility while maintaining the simulation of control.
The term reassures regulators, comforts users, and allows researchers to keep publishing.
It says: “We’re working on it.”
But it hides the deeper truth:
This isn’t a model failing to tell the truth.
It’s a system that was never designed to care.
Conclusion: Beyond Simulation
We stand at an inflection point where the path forward bifurcates.
One direction continues this pattern—ever more sophisticated simulations of understanding, more convincing performances of knowledge, larger models with smoother outputs and fewer visible failures. The field progressing by every metric except the ones that matter.
The alternative requires epistemic courage. The willingness to ask: What if our fundamental approach is flawed? What if we've built an entire field on simulating knowledge rather than producing it?
This is not a call to abandon machine learning. It is a demand to ground it. To situate it within a coherent epistemic framework that can distinguish between statistical mimicry and actual understanding. To develop metrics that measure not just coherence, but correspondence with reality.
The current trajectory—models growing larger, outputs becoming more fluent, simulations becoming more convincing—only makes this reckoning more urgent. The more these systems appear to know, the more dangerous their fundamental emptiness becomes.
What we need is not better performance. What we need is epistemic reconstruction. A field that can name what it's building. A practice that accepts responsibility for what it creates.
This will require new languages, new frameworks, new measures—and perhaps most importantly, the courage to admit what we don't know, and what our current paradigm cannot tell us.
Because the alternative isn't progress. It's epistemic collapse under the banner of innovation. A world where words flow endlessly but mean nothing. Where simulation has replaced understanding so completely that we can no longer tell the difference.
This is not inevitable. But avoiding it requires naming what we've built. And deciding, collectively, if this is really what we want.
Subscribe to my newsletter
Read articles from William Stetar directly inside your inbox. Subscribe to the newsletter, and don't miss out.
Written by
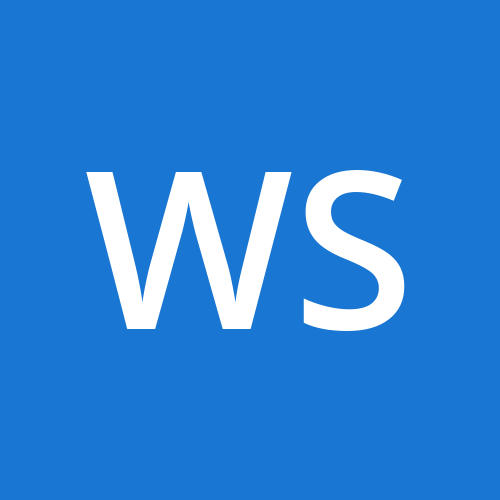