The Central Limit Theorem Demystified: A Data Scientist's Ultimate Guide
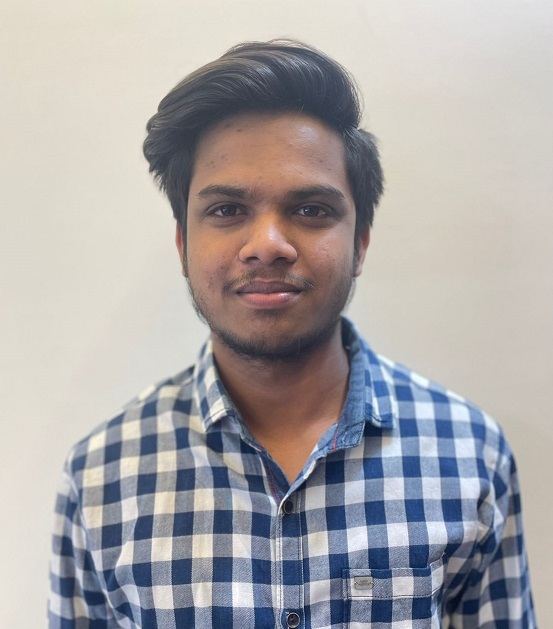
Table of contents
- Introduction
- CLT Explained: The Key Idea
- Why CLT is the Unsung Hero of Data Science
- Why Data Scientists Care About CLT
- Breaking Down CLT: Beyond the Textbook Definition
- The Math Behind CLT (Light Version)
- CLT in Practice: A Data Scientist's Toolkit
- Python Simulation: Watch CLT Transform Data
- When CLT Doesn't Apply: Know the Limits
- Pro Tips for Data Scientists
- Advanced Insights for Practitioners
- Conclusion: CLT as Your Data Science Superpower
#66DaysOfData – Day 2
Introduction
The Central Limit Theorem (CLT) is the statistical "magic trick" that lets data scientists make reliable inferences from real-world data—even when that data is messy, skewed, or non-normal.
CLT Explained: The Key Idea
In Plain English
"If you take large enough random samples from any population and calculate their averages, those averages will follow a normal distribution—even if the original data doesn’t."
Why This Matters
Real-world data is rarely normal (e.g., income, website dwell time).
CLT lets us use normal-distribution-based tools (confidence intervals, p-values) anyway.
Visual Analogy
Imagine rolling a die:
Single roll = Uniform distribution (1–6, equal probability).
Average of 30 rolls = Bell curve (peaks at 3.5).
Why CLT is the Unsung Hero of Data Science
In the chaotic world of real-world data—where skewed distributions, outliers, and non-normal patterns reign supreme—the Central Limit Theorem (CLT) emerges as statistical salvation. This fundamental principle is why we can:
✔ Compute confidence intervals from messy data
✔ Run A/B tests on non-normal metrics
✔ Trust p-values in hypothesis testing
✔ Apply machine learning algorithms that assume normality
Why Data Scientists Care About CLT
Application | How CLT Helps | Example |
A/B Testing | Compare means of two groups (e.g., webpage versions) | "Is Button A’s 5% higher click rate statistically significant?" |
Confidence Intervals | Estimate population parameters from samples | "Users spend 120 ± 10 mins/day (95% CI)" |
Machine Learning | Justifies normality assumptions in models | Linear regression residuals ~ Normal |
Quality Control | Monitor manufacturing processes | "Is today’s batch mean thickness abnormal?" |
Breaking Down CLT: Beyond the Textbook Definition
The Core Principle
"Take large enough random samples from any population (regardless of its shape), and the distribution of their means will approximate a normal curve."
Why This is Revolutionary
Real-world data is rarely normal (e.g., income, hospital wait times, website engagement).
CLT lets us use normal-distribution-based tools even when raw data looks nothing like a bell curve.
Visual Analogy: The Dice Experiment
🎲 Single die roll → Uniform distribution (1-6, equal probability)
🎲 Average of 30 rolls → Perfect bell curve (centered at 3.5)
The Math Behind CLT (Light Version)
For a population with:
Mean =
μ
Standard deviation =
σ
The sampling distribution of the mean has:
Mean =
μ
(same as population)Standard Error =
σ/√n
(shrinks asn
grows)
Key Takeaway:
Larger samples → Tighter distribution around
μ
.n ≥ 30 is a common rule of thumb for "large enough."
CLT in Practice: A Data Scientist's Toolkit
1. Confidence Intervals
# 95% CI for population mean using CLT
import scipy.stats as stats
mean = np.mean(sample)
std_error = np.std(sample)/np.sqrt(len(sample))
ci = stats.norm.interval(0.95, loc=mean, scale=std_error)
Why it works: CLT guarantees the sampling distribution is normal, so we can use norm.interval()
.
2. A/B Testing
Compare conversion rates (binary) or session durations (right-skewed)
CLT validates using t-tests even when raw metrics are non-normal
3. Machine Learning
Linear regression assumes normally distributed errors
CLT justifies this for large datasets (
n > 30
)
4. Quality Control
Monitor manufacturing dimensions (often skewed due to tool wear)
Set control limits using
μ ± 3σ/√n
(thanks to CLT)
Python Simulation: Watch CLT Transform Data
Skewed Population → Normal Sample Means
import numpy as np
import matplotlib.pyplot as plt
# Highly skewed population (exponential)
population = np.random.exponential(scale=2.0, size=100000)
# CLT in action: sample means become normal
sample_means = [np.mean(np.random.choice(population, 30)) for _ in range(1000)]
plt.hist(population, bins=50, alpha=0.5, label='Original Data (Skewed)')
plt.hist(sample_means, bins=50, alpha=0.5, label='Sample Means (Normal)')
plt.legend()
plt.show()
Key Observation:
Left plot: Raw exponential data (peaked at 0, long right tail)
Right plot: Distribution of means (symmetric, normal)
When CLT Doesn't Apply: Know the Limits
Failure Cases
Scenario | Why CLT Fails | Alternative |
Small samples (n < 30) | Not enough for convergence | Non-parametric tests (Mann-Whitney) |
Heavy-tailed distributions | Infinite variance breaks CLT | Robust statistics (median-based) |
Dependent data | Autocorrelation violates i.i.d. | Time series models (ARIMA) |
Pro Tips for Data Scientists
🔹 Check sample size: n ≥ 30
is safe for mild skewness; n ≥ 50
for heavy skew.
🔹 Beware of outliers: CLT is robust but can be misled by extreme values.
🔹 Verify with Q-Q plots: Ensure sample means are truly normal.
Pro Tip: Diagnose with Q-Q Plots
import statsmodels.api as sm
sm.qqplot(sample_means, line='s') # Straight line = normality
plt.show()
Advanced Insights for Practitioners
1. How Large is "Large Enough"?
Mild skewness: n ≥ 30
Extreme skewness: n ≥ 50 (e.g., income data)
Binary data (p=0.01): Need np ≥ 10 (rule of thumb)
2. Edge Case: CLT for Proportions
For binary data (e.g., conversion rates), the sampling distribution of p̂ is normal if:
n \times p \geq 10 \quad \text{and} \quad n \times (1-p) \geq 10
3. Bootstrapping & CLT Synergy
Bootstrap resampling relies on CLT to justify normality of the bootstrap distribution
Works because mean of means always trends toward normal
Conclusion: CLT as Your Data Science Superpower
✅ Universal Applicability: Works on any distribution (with caveats)
✅ Enables Parametric Methods: t-tests, ANOVA, linear regression
✅ Scales with Data: More data → Better normality approximation
✅ CLT is why we can trust averages—even from weird data.
✅ Sample size matters: Bigger n
→ Better normality.
✅ Not a cure-all: Know when to use alternatives.
Final Wisdom:
"CLT doesn’t make your data normal—it makes your analysis of the data possible. Master it, and you unlock the true power of statistical inference."
Subscribe to my newsletter
Read articles from Ashutosh Kurwade directly inside your inbox. Subscribe to the newsletter, and don't miss out.
Written by
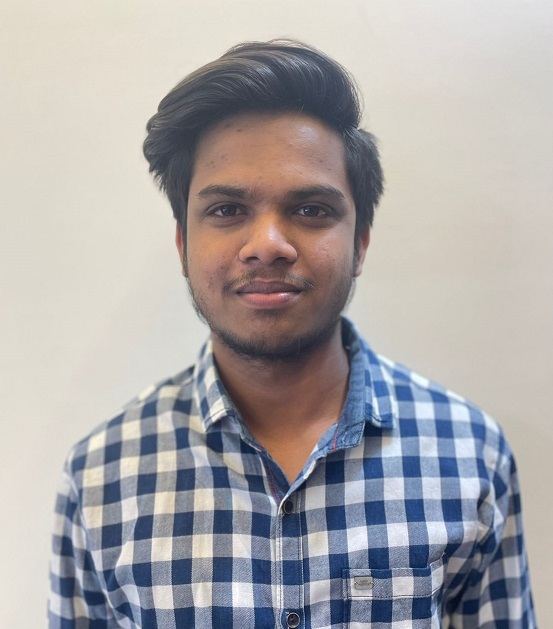