Ethical AI in Insurance: Preventing Bias in Risk Assessment Models
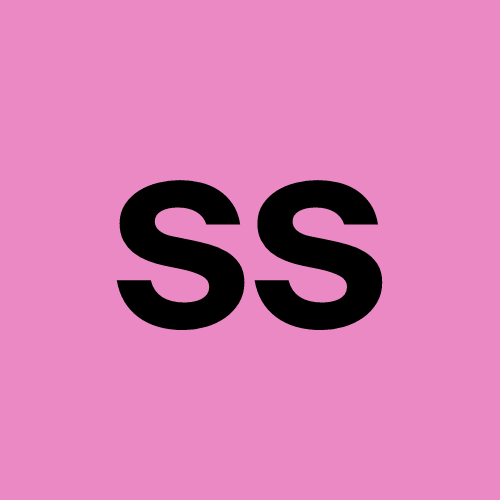

Artificial Intelligence (AI) is revolutionizing the insurance industry by streamlining operations, personalizing offerings, and enhancing risk assessment models. With advanced algorithms capable of processing vast datasets, insurers can now predict risks with remarkable precision. However, the growing reliance on AI raises a critical concern: the potential for algorithmic bias. When left unchecked, biased AI models can lead to unfair treatment of individuals or groups, particularly in areas like underwriting, claims assessment, and premium pricing. Ethical AI practices are essential to ensure fairness, transparency, and trust in the insurance sector.
The Promise and Peril of AI in Risk Assessment
Traditionally, insurers relied on historical data and actuarial science to assess risk and set premiums. AI enhances this process by integrating non-traditional data sources such as social media behavior, IoT data from connected devices, and credit scores. This expansion allows for a more granular and individualized understanding of risk.
However, AI systems learn from data, and data often reflects historical and societal biases. For instance, if past insurance decisions were influenced by discriminatory practices, such as redlining or gender-based pricing, AI models trained on such data may replicate or even amplify these biases.
EQ.1 : Disparate Impact (DI) Ratio
Real-World Consequences of Bias
Algorithmic bias in insurance is not just a technical issue—it has real-world implications. Consider the following scenarios:
Discriminatory Pricing: A model that disproportionately increases premiums for individuals from certain ZIP codes may unintentionally penalize people from marginalized communities.
Unfair Claim Denials: AI used in claims processing may flag certain demographic groups as high-risk based on biased patterns, leading to unjust denials or delays.
Exclusion from Coverage: Automated underwriting models might misclassify individuals with nontraditional employment or education backgrounds as high-risk, excluding them from affordable coverage.
These examples underscore the importance of developing AI systems that align with ethical and regulatory standards.
Understanding Sources of Bias in AI Models
To prevent bias, it's essential to first understand how it enters AI systems. Common sources include:
Data Bias: Historical data used for training may reflect existing prejudices or imbalances.
Sample Bias: If the training data does not adequately represent all segments of the population, the model's predictions will be skewed.
Label Bias: Outcomes used to train the model (e.g., "fraud" or "high risk") may themselves be biased if human decisions were flawed.
Measurement Bias: Inputs such as credit scores or health data may not accurately capture an individual’s true risk level, especially across diverse populations.
Strategies for Preventing Bias in AI-Driven Insurance
1. Diverse and Representative Data Collection
Ensuring that the training data is representative of the population the model will serve is fundamental. This includes collecting data across various demographics such as race, gender, age, geography, and income levels. Additionally, insurers must avoid over-relying on proxies that may indirectly introduce sensitive attributes (e.g., using ZIP codes as proxies for race).
2. Bias Audits and Fairness Metrics
Insurers should implement regular audits to evaluate their models for fairness using established metrics like demographic parity, equal opportunity, and disparate impact analysis. These audits can identify patterns where the model may be treating certain groups unfairly.
3. Algorithmic Transparency and Explainability
Black-box models, while powerful, often lack transparency. To foster trust and accountability, insurers should prioritize interpretable models or implement tools that explain decisions in human-readable terms. This enables regulators, customers, and internal stakeholders to understand how a particular outcome was reached.
4. Human-in-the-Loop Oversight
AI should augment—not replace—human judgment. Critical decisions, especially those that impact an individual’s access to insurance, should be reviewed by human experts who can assess contextual factors that the model might overlook. Human oversight can serve as a fail-safe against unjust outcomes.
5. Ethical AI Governance Frameworks
Developing a comprehensive governance framework is key to managing AI ethics. This includes setting clear policies around data usage, model development, accountability, and compliance with legal standards like the EU’s AI Act or the U.S. Equal Credit Opportunity Act (ECOA). Ethical review boards and cross-functional AI ethics committees can help ensure these policies are consistently enforced.
6. Consumer Transparency and Consent
Insurers should clearly communicate how AI is used in decision-making and obtain informed consent when collecting data. Consumers have a right to know how their personal information influences pricing, eligibility, and claims decisions.
Regulatory Landscape and Compliance
Governments and regulators are increasingly scrutinizing the use of AI in high-stakes domains like insurance. For instance:
The European Union’s AI Act proposes risk-based regulation of AI systems, requiring greater oversight for models that impact access to financial services.
In the United States, several states have introduced or passed laws mandating transparency and fairness in algorithmic decision-making in insurance.
The National Association of Insurance Commissioners (NAIC) has emphasized the need for governance frameworks that address AI ethics, data privacy, and consumer protection.
Insurers that proactively address these issues will not only avoid legal pitfalls but also gain a competitive edge by demonstrating commitment to ethical innovation.
EQ.2 : Equal Opportunity Difference (EOD)
Building Trust in AI-Powered Insurance
Ultimately, the ethical use of AI in insurance is about building trust—between insurers and consumers, between businesses and regulators, and between technology and society. Trust cannot be achieved through technology alone; it requires intentional design, robust oversight, and continuous engagement with ethical principles.
Companies that lead with fairness and transparency will differentiate themselves in a market where consumers are increasingly aware and concerned about how their data is used. Ethical AI practices are not just a moral obligation; they are a strategic imperative.
Conclusion
As AI continues to reshape the insurance landscape, it brings both immense potential and significant ethical challenges. Bias in risk assessment models can perpetuate inequalities and undermine consumer confidence. By prioritizing fairness, transparency, and accountability, insurers can harness the benefits of AI while safeguarding the rights and dignity of all individuals. Ethical AI in insurance is not merely about compliance—it is about ensuring that technological advancement serves the broader goal of social good.
Subscribe to my newsletter
Read articles from Sneha Singireddy directly inside your inbox. Subscribe to the newsletter, and don't miss out.
Written by
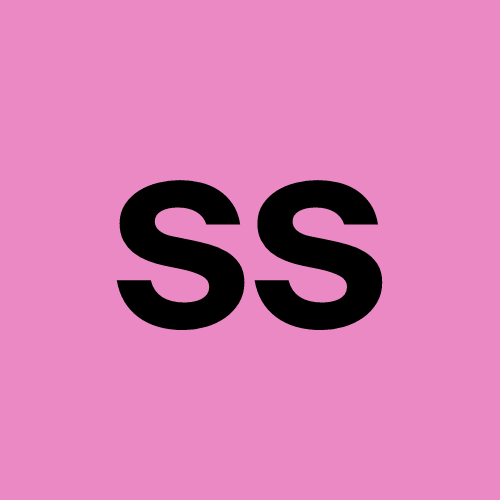