Looker vs Tableau: Navigating the Future of Data Visualization in an AI-Driven World
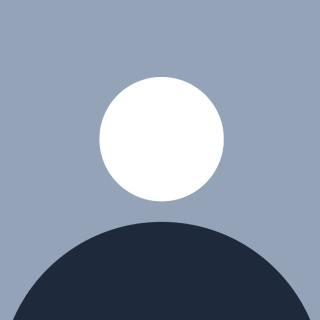
When it comes to data visualization and business intelligence tools, the Looker vs Tableau debate stands at the forefront of many organizations' decision-making processes. These powerful platforms offer distinct approaches to transforming raw data into actionable insights. While Looker emphasizes structured data modeling through its proprietary LookML language, Tableau focuses on flexible, user-driven visualization capabilities. Both tools have carved out significant market share in the data analytics space, but their fundamental differences in approach, coupled with the emergence of AI-powered alternatives, create important considerations for businesses seeking the right visualization solution. Understanding these differences is crucial for organizations looking to make informed decisions about their data visualization strategy.
Core Purpose and Fundamental Approaches
Looker's Structured Framework
Looker's foundation rests on its Structured Looker Modeling Language (LookML), creating a robust but relatively inflexible environment for data analysis. This structured approach enforces strict data governance and maintains consistency across an organization. Teams using Looker benefit from standardized metrics and definitions, ensuring that everyone works from the same source of truth. However, this rigid framework can limit creative exploration and requires specialized knowledge to implement changes or add new metrics.
Tableau's Flexible Analysis
Tableau takes an opposing approach, prioritizing user freedom and creative exploration. Its interface encourages analysts to investigate data through various angles without the constraints of predetermined models. This flexibility enables quick insights and experimental analysis, but it can lead to inconsistencies across departments. Without enforced standards, different teams might produce conflicting results from the same data sets, potentially creating confusion in organizational reporting.
The AI Evolution
Modern AI-driven tools are reshaping the landscape of data visualization by combining the best aspects of both approaches while eliminating their limitations. These emerging platforms use artificial intelligence to automate many traditional BI processes, from data modeling to visualization selection. They can suggest optimal ways to present data, identify patterns, and generate insights automatically, reducing the technical expertise required for meaningful analysis.
Impact on Business Operations
The choice between these approaches significantly affects how organizations handle their data analysis workflow. Looker's structured environment works well for enterprises requiring strict data governance and consistent reporting across departments. Its centralized model ensures data integrity but may slow down the implementation of new analytics requirements. Tableau's flexible approach better serves organizations that value rapid exploration and creative analysis, though this freedom may require additional oversight to maintain consistency. AI-driven tools offer a middle ground, providing both structure and flexibility while reducing the technical barriers to entry.
Visualization Capabilities and Implementation
Tableau's Visual Strengths
Tableau excels in visualization options, offering users a comprehensive suite of charting and graphing capabilities. Its robust platform supports everything from basic bar charts to complex statistical visualizations. The tool's extensive customization options allow analysts to fine-tune every aspect of their visual presentations. However, this wealth of options comes with a learning curve - users often need to invest significant time mastering Tableau's features to create sophisticated visualizations effectively.
Looker's Visualization Approach
Looker provides a more streamlined but limited set of visualization options. While it covers most common business reporting needs, its graphing capabilities are more constrained compared to Tableau. This limitation often requires users to work within predetermined visual frameworks, which can be restrictive for complex analytical presentations. The advantage lies in consistency and ease of implementation for standard business reporting, but creative visualization projects may face limitations.
The AI Visualization Revolution
Emerging AI-powered visualization tools are transforming how users interact with data. These platforms can automatically recommend the most effective visualization types based on the data structure and intended message. By analyzing patterns and relationships within datasets, AI tools can suggest optimal visual representations without requiring extensive user expertise. This automation streamlines the creation process and helps ensure that visualizations effectively communicate data insights.
Practical Implementation Challenges
Both traditional platforms face practical challenges in implementation. Tableau users often encounter situations where creating specific visualizations requires extensive research, custom coding, or community support. Looker users might find themselves limited by the platform's visualization options, necessitating workarounds or alternative solutions. The emergence of AI-driven tools addresses these challenges by providing intelligent assistance and automated solutions, though they're still evolving in terms of capabilities and market adoption.
Future Visualization Trends
The future of data visualization points toward more intuitive, AI-assisted interfaces that combine the flexibility of Tableau with the consistency of Looker. These tools will likely feature natural language processing for visualization creation, automated insight generation, and dynamic adaptation to user needs. This evolution promises to make sophisticated data visualization accessible to a broader range of users while maintaining professional standards and analytical depth.
Scalability and Enterprise Implementation
Tableau's Deployment Options
Tableau offers two primary enterprise deployment models: Tableau Server and Tableau Cloud. Tableau Server provides organizations with complete control over their infrastructure, allowing for customized resource allocation and security configurations. This on-premises solution enables direct connections to local data sources and offers superior flexibility in managing user loads. However, it requires dedicated IT resources for maintenance, updates, and performance optimization.
Tableau Cloud Considerations
The cloud-based version of Tableau presents a different set of advantages and challenges. While it eliminates the need for physical infrastructure management, it may introduce complexity when connecting to on-premises data sources. Organizations must often implement Tableau Bridge to facilitate these connections, which can impact performance and add another layer of technical complexity. Cloud deployment works best for companies primarily using cloud-based data sources and requiring minimal local data integration.
Looker's Cloud-Native Architecture
Looker's cloud-native approach simplifies deployment by eliminating the need for on-premises infrastructure. As a Google Cloud product, it integrates seamlessly with Google's ecosystem, particularly BigQuery. This architecture provides automatic scaling capabilities and reduces administrative overhead. However, organizations heavily invested in non-Google cloud platforms or requiring on-premises solutions may face integration challenges.
Resource Management and Performance
Both platforms require careful consideration of resource management as user bases grow. Tableau Server demands proactive monitoring and adjustment of hardware resources to maintain performance under increasing loads. Looker's cloud-native approach automatically handles many scaling aspects but may incur higher costs during peak usage periods. Organizations must balance performance requirements with budget constraints when choosing between these options.
Future Scalability Trends
The future of enterprise analytics points toward hybrid solutions that combine cloud flexibility with local processing power. AI-driven resource optimization is becoming increasingly important, automatically adjusting system resources based on usage patterns and demand. These advances will likely reduce the manual overhead currently required for scaling traditional BI platforms, while improving performance and cost efficiency. Organizations should consider their long-term scalability needs and the evolving capabilities of AI-enhanced analytics platforms when making infrastructure decisions.
Conclusion
The choice between Looker and Tableau represents a fundamental decision about an organization's approach to data visualization and analysis. Looker's structured, model-driven approach provides consistency and governance but requires specialized knowledge and accepts limited flexibility. Tableau offers creative freedom and powerful visualization capabilities but may lead to inconsistent reporting and requires significant user training for advanced features.
The emergence of AI-powered analytics platforms is reshaping this landscape, offering solutions that bridge the gap between these traditional tools. These new platforms combine the governance of Looker with the flexibility of Tableau, while reducing technical barriers through natural language processing and automated insights.
Organizations must evaluate their specific needs, considering factors such as:
Technical expertise of their team
Required level of data governance
Desired balance between flexibility and consistency
Integration requirements with existing systems
Long-term scalability needs
As AI continues to advance, the distinction between traditional visualization tools may become less relevant, with focus shifting toward platforms that can intelligently adapt to user needs while maintaining data integrity and analytical rigor. Organizations should remain aware of these evolving capabilities when planning their data visualization strategy.
Subscribe to my newsletter
Read articles from Mikuz directly inside your inbox. Subscribe to the newsletter, and don't miss out.
Written by