AI-Powered Sentiment Analysis in Social Media for Mental Health Trends

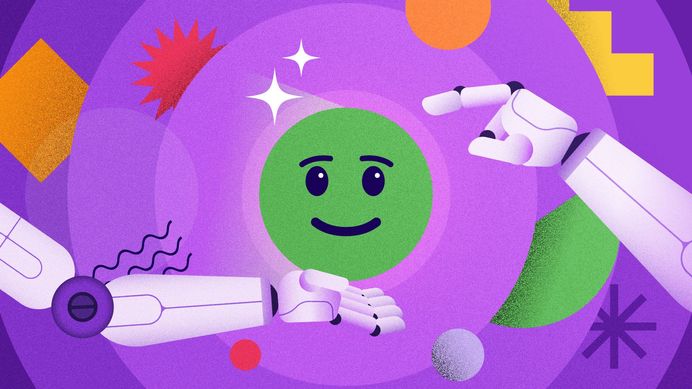
Introduction
In recent years, social media has become a pivotal platform for individuals to express emotions, share experiences, and seek support for mental health issues. From Twitter and Facebook to Instagram and TikTok, these platforms have revolutionized how people communicate, often serving as both a refuge and a battleground for mental health discussions. While social media can facilitate connection, it also comes with its own set of challenges, including the potential for misinformation, stigma, and harmful content. In response, a growing field of research and application has emerged focusing on leveraging artificial intelligence (AI) to analyze sentiment and detect mental health trends within these online platforms. AI-powered sentiment analysis offers an innovative way to monitor, assess, and predict mental health concerns based on individuals’ posts and interactions, enabling early intervention and improved understanding of public mental health.
Understanding Sentiment Analysis
Sentiment analysis, also known as opinion mining, is a branch of natural language processing (NLP) that uses AI and machine learning techniques to assess the emotions or sentiments expressed in textual data. This can involve classifying text as positive, negative, or neutral, or even identifying more specific emotional states, such as happiness, sadness, anger, or anxiety. In the context of social media, sentiment analysis can be applied to user posts, comments, tweets, or discussions to gauge the overall emotional tone of conversations. The results can then be aggregated to identify patterns, track shifts in public sentiment over time, and even predict potential mental health crises within specific populations or regions.
Eq.1. Equation for Sentiment Score of a Post:
The Role of AI in Mental Health Sentiment Analysis
The combination of sentiment analysis and AI has proven to be a powerful tool in understanding mental health trends. Traditional methods of mental health research often involve time-consuming surveys, interviews, and clinical assessments. However, AI-driven sentiment analysis offers the ability to process vast amounts of data in real time, identifying emerging trends and capturing subtle nuances in language that may indicate distress or mental health issues. AI models can be trained to detect signs of mental health conditions such as depression, anxiety, loneliness, and suicidal ideation by analyzing the language and emotional tone used in social media posts.
For instance, deep learning models can be trained on labeled datasets of text that include various mental health-related topics. These models learn to recognize patterns and keywords associated with mental health issues, such as terms related to mood (e.g., "sad," "hopeless," "anxious") or behaviors (e.g., "isolated," "withdrawn"). By continuously analyzing social media data, AI systems can help identify groups or individuals who may be at risk, offering valuable insights for mental health professionals, public health agencies, or community support systems.
Applications of AI Sentiment Analysis in Mental Health
Early Detection of Mental Health Issues
One of the most promising applications of AI-powered sentiment analysis is in early detection. By analyzing social media posts for negative emotional signals, AI systems can help identify individuals who may be experiencing mental health challenges before they seek formal treatment. Early intervention is crucial in managing mental health disorders, as it can lead to more effective treatment outcomes. Sentiment analysis can provide an unobtrusive way to monitor the mental state of individuals, offering an additional layer of awareness for caregivers, therapists, and family members.Tracking Public Mental Health Trends
On a broader scale, sentiment analysis can track the mental health of entire populations or communities. By analyzing large datasets of social media content, researchers and policymakers can gain insights into the mental well-being of the general public. For example, patterns of increased anxiety or sadness during major events such as natural disasters, political upheaval, or pandemics can be identified, allowing governments and healthcare organizations to tailor responses and resources. Additionally, monitoring public sentiment can help assess the effectiveness of mental health campaigns, therapy interventions, or policy changes.
Eq.2. Aggregation Over Time:
Supporting Crisis Management
AI sentiment analysis can be used in real-time to detect signs of distress, such as suicidal ideation or severe depression, in social media posts. When such posts are identified, they can be flagged for immediate attention by mental health professionals or crisis intervention teams. This type of intervention can be vital in providing support to individuals in moments of acute need, potentially saving lives. Several organizations are already utilizing AI-powered systems to monitor online platforms for signs of self-harm or suicidal thoughts, allowing them to offer support or direct individuals to appropriate resources.
Improving Mental Health Services
By aggregating sentiment data, AI can help identify gaps in mental health services and pinpoint areas where additional support is needed. For example, if a particular region exhibits a higher volume of distress signals related to a specific mental health issue, healthcare providers can deploy targeted interventions to address the problem. This data can also help improve the quality of care by informing the development of better therapeutic approaches, online support networks, or community outreach programs.
Challenges and Ethical Considerations
While AI-powered sentiment analysis holds great potential for improving mental health awareness and care, there are several challenges and ethical considerations that must be addressed. One major concern is privacy. Social media platforms often contain sensitive personal information, and analyzing posts for sentiment may inadvertently expose private details about users' mental health. It is essential that AI systems respect users' privacy and adhere to ethical guidelines when processing sensitive data. Additionally, there is the issue of algorithmic bias. AI models are only as good as the data they are trained on, and if these datasets contain biased or incomplete information, the analysis may be inaccurate or discriminatory. Careful attention must be paid to ensure that AI systems are trained on diverse and representative datasets to avoid reinforcing harmful stereotypes or overlooking certain mental health conditions.
Another concern is the potential for over-reliance on AI for diagnosing or monitoring mental health. While AI can provide valuable insights, it should not replace human intervention or professional evaluation. Instead, AI should be viewed as a tool to augment and support mental health care, not as a substitute for it.
Conclusion
AI-powered sentiment analysis offers a promising approach to understanding mental health trends on social media. By leveraging the vast amounts of data generated on these platforms, AI systems can identify early signs of mental health issues, track public sentiment, and provide valuable insights for mental health professionals and policymakers. While there are challenges related to privacy, bias, and the ethical use of AI, these technologies have the potential to significantly improve mental health care and support systems. As AI continues to evolve, it will undoubtedly play a crucial role in shaping the future of mental health research and intervention.
Subscribe to my newsletter
Read articles from Chandrashekhar Pandugula directly inside your inbox. Subscribe to the newsletter, and don't miss out.
Written by
